Which Of The Following Research Designs Will Allow Cause-and-effect Conclusions
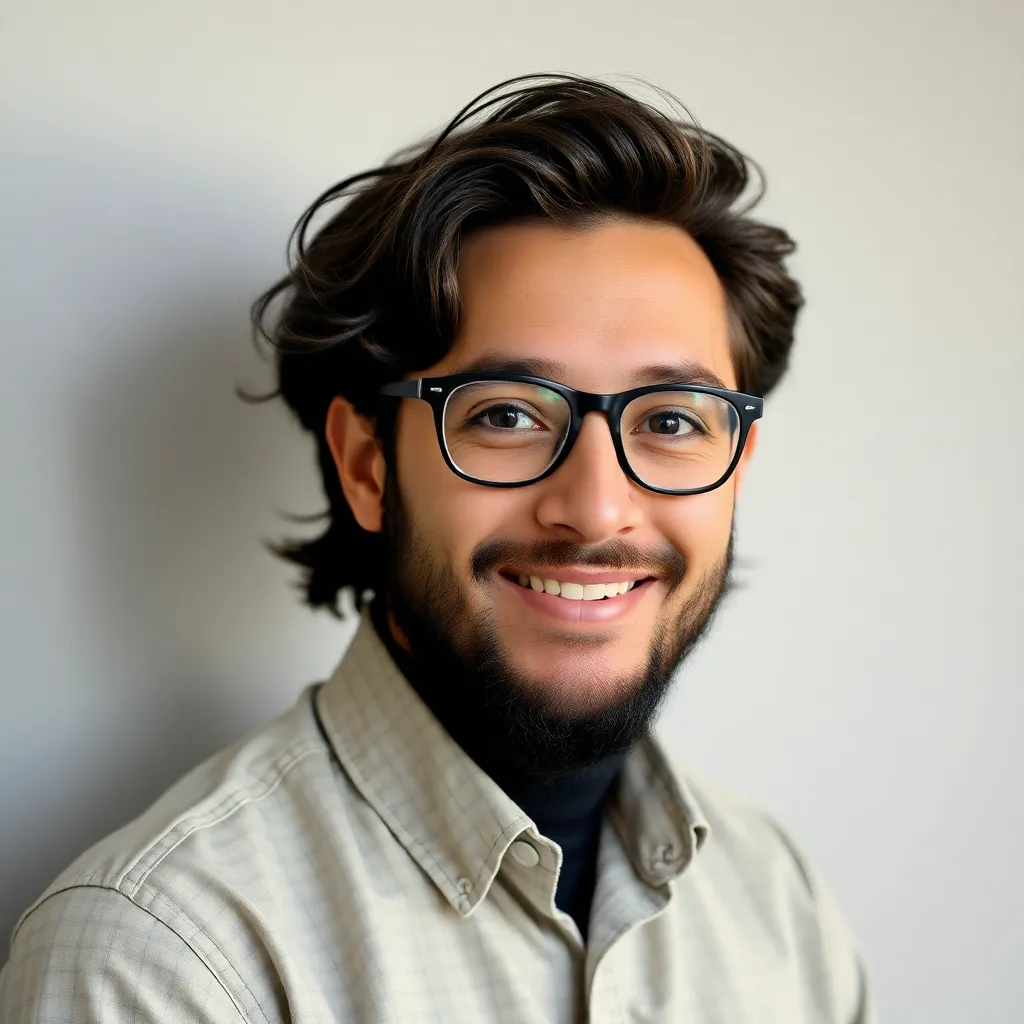
Holbox
Mar 26, 2025 · 6 min read
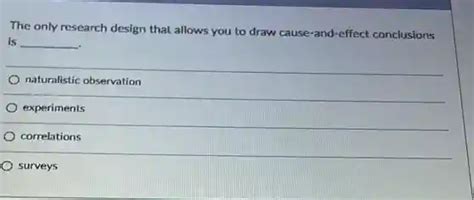
Table of Contents
- Which Of The Following Research Designs Will Allow Cause-and-effect Conclusions
- Table of Contents
- Which Research Designs Allow Cause-and-Effect Conclusions?
- The Importance of Establishing Causality
- Research Designs and Their Ability to Infer Causality
- 1. Experimental Designs: The Gold Standard for Causality
- 2. Observational Designs: Exploring Causality Without Manipulation
- 3. Strengthening Causal Inference in Observational Studies
- Conclusion: Choosing the Right Research Design
- Latest Posts
- Latest Posts
- Related Post
Which Research Designs Allow Cause-and-Effect Conclusions?
Understanding causality is crucial in research. We often want to know not just what is happening, but why. This means determining if one variable directly influences another – establishing a cause-and-effect relationship. However, simply observing a correlation between two variables isn't enough to conclude causality. This article delves into various research designs and their capabilities in establishing cause-and-effect relationships, highlighting the strengths and limitations of each.
The Importance of Establishing Causality
Before we dive into specific research designs, let's understand why establishing causality is so important. Causality allows us to:
- Make accurate predictions: If we know X causes Y, we can predict that changes in X will lead to predictable changes in Y.
- Develop effective interventions: Understanding causal relationships is essential for designing interventions aimed at changing outcomes. For example, if we know a specific factor causes a disease, we can develop targeted treatments or preventative measures.
- Advance theoretical understanding: Establishing causal links helps refine and expand existing theories, leading to a more complete understanding of the phenomena under study.
- Improve decision-making: Causal knowledge informs better decisions in various fields, from public policy to personal choices.
Research Designs and Their Ability to Infer Causality
Several research designs can be used to investigate causal relationships. However, their capacity to establish causality varies significantly. The strength of causal inference depends on how well the design controls for confounding variables and establishes temporal precedence.
1. Experimental Designs: The Gold Standard for Causality
Experimental designs, often considered the gold standard for establishing causality, involve manipulating an independent variable (the presumed cause) to observe its effect on a dependent variable (the presumed effect). Key features include:
- Random assignment: Participants are randomly assigned to different groups (e.g., treatment and control groups), minimizing pre-existing differences that could confound the results. This is crucial for ensuring that any observed difference between groups is due to the manipulation of the independent variable, not other factors.
- Manipulation of the independent variable: The researcher actively manipulates the independent variable, creating different levels or conditions. This allows for direct observation of the independent variable's impact on the dependent variable.
- Control group: A control group, which doesn't receive the treatment or manipulation, provides a baseline for comparison. This allows researchers to determine if the observed changes in the treatment group are significantly different from what would be expected without the intervention.
Types of Experimental Designs:
- Pre-experimental designs: These designs lack the rigor of true experimental designs and often don't adequately control for confounding variables. Examples include one-shot case studies and one-group pretest-posttest designs. They offer weak support for causal inferences.
- True experimental designs: These designs employ random assignment and manipulation of the independent variable, providing stronger evidence for causality. Examples include pre-test/post-test control group designs, post-test only control group designs, and Solomon four-group designs.
- Quasi-experimental designs: These designs lack random assignment but still manipulate the independent variable. They are useful when random assignment is not feasible or ethical, but their ability to establish causality is weaker than true experimental designs due to the potential for confounding variables. Examples include non-equivalent control group designs and interrupted time series designs.
Strengths of Experimental Designs:
- Strongest evidence for causality: The combination of random assignment and manipulation of the independent variable allows for strong inferences about cause-and-effect relationships.
- Control over confounding variables: Random assignment minimizes the influence of confounding variables, increasing the internal validity of the study.
- Replication: Experimental designs are often easily replicated, which strengthens the reliability of the findings.
Limitations of Experimental Designs:
- Artificiality: The controlled environment of experiments can sometimes lack ecological validity, meaning the findings may not generalize well to real-world settings.
- Ethical limitations: In some cases, manipulating the independent variable may be unethical or impractical.
- Cost and time: Experimental designs can be expensive and time-consuming to conduct.
2. Observational Designs: Exploring Causality Without Manipulation
Observational designs do not involve manipulating the independent variable. Researchers observe naturally occurring variations in the independent and dependent variables. While they can't establish causality with the same certainty as experimental designs, they can provide valuable insights and suggest causal relationships.
Types of Observational Designs:
- Cohort studies: Follow a group of individuals over time to observe the incidence of a particular outcome. This design allows for the examination of temporal precedence, which is crucial for inferring causality.
- Case-control studies: Compare individuals with a particular outcome (cases) to individuals without the outcome (controls) to identify potential risk factors. While they don't establish temporal precedence definitively, they can suggest potential causal relationships.
- Cross-sectional studies: Examine the relationship between variables at a single point in time. This design cannot establish temporal precedence, limiting its ability to infer causality. It can, however, reveal correlations that might suggest further investigation using more rigorous designs.
Strengths of Observational Designs:
- Ethical feasibility: Observational studies can be conducted when manipulating the independent variable is unethical or impossible.
- Ecological validity: Observational studies are often conducted in real-world settings, increasing their generalizability.
- Cost-effectiveness: Observational studies are often less expensive and time-consuming than experimental designs.
Limitations of Observational Designs:
- Weaker evidence for causality: The lack of manipulation and random assignment makes it difficult to rule out confounding variables, weakening causal inferences.
- Difficulties in establishing temporal precedence: Determining which variable occurred first can be challenging, making it difficult to determine directionality.
- Susceptibility to bias: Observational studies are vulnerable to various biases, such as selection bias and recall bias, which can affect the validity of the findings.
3. Strengthening Causal Inference in Observational Studies
While observational studies don't provide the same level of causal certainty as experimental designs, several strategies can help strengthen causal inferences:
- Controlling for confounders: Statistical techniques, such as regression analysis, can be used to control for the influence of confounding variables, improving the accuracy of causal estimates.
- Propensity score matching: This technique matches individuals in different groups based on their probability of receiving the treatment or exposure, reducing selection bias.
- Instrumental variables: This method uses a third variable (the instrument) that is related to the independent variable but not directly to the dependent variable, to estimate the causal effect.
- Longitudinal studies: Following participants over extended periods enhances the ability to establish temporal precedence and reduce the impact of confounding variables.
Conclusion: Choosing the Right Research Design
The choice of research design depends heavily on the research question, available resources, and ethical considerations. While experimental designs offer the strongest evidence for causality, observational designs can be valuable when experimentation is not feasible. Regardless of the chosen design, careful consideration of potential biases and confounding variables is crucial for drawing accurate conclusions about cause-and-effect relationships. By employing rigorous methods and appropriate statistical techniques, researchers can enhance the validity of their causal inferences, leading to more robust and meaningful findings. The key takeaway is to understand the strengths and limitations of each design and select the approach that best suits the specific research context to maximize the potential for drawing reliable conclusions about cause and effect. Always critically evaluate the methodology and the strength of the evidence presented before accepting a causal claim.
Latest Posts
Latest Posts
-
Marine Magnetic Anomaly Belts Run Parallel To
Mar 30, 2025
-
Which Of The Following Describes Gene Cloning
Mar 30, 2025
-
Solubility Iodoform And Oxidation Of Aldehydes And Ketones
Mar 30, 2025
-
Draw The Structure Of 3 4 Dimethyl 1 Pentene
Mar 30, 2025
-
What Happens To Cdks In The Absence Of Cyclins
Mar 30, 2025
Related Post
Thank you for visiting our website which covers about Which Of The Following Research Designs Will Allow Cause-and-effect Conclusions . We hope the information provided has been useful to you. Feel free to contact us if you have any questions or need further assistance. See you next time and don't miss to bookmark.