Identify The Advantages And Disadvantages Of Cloud-based Ai
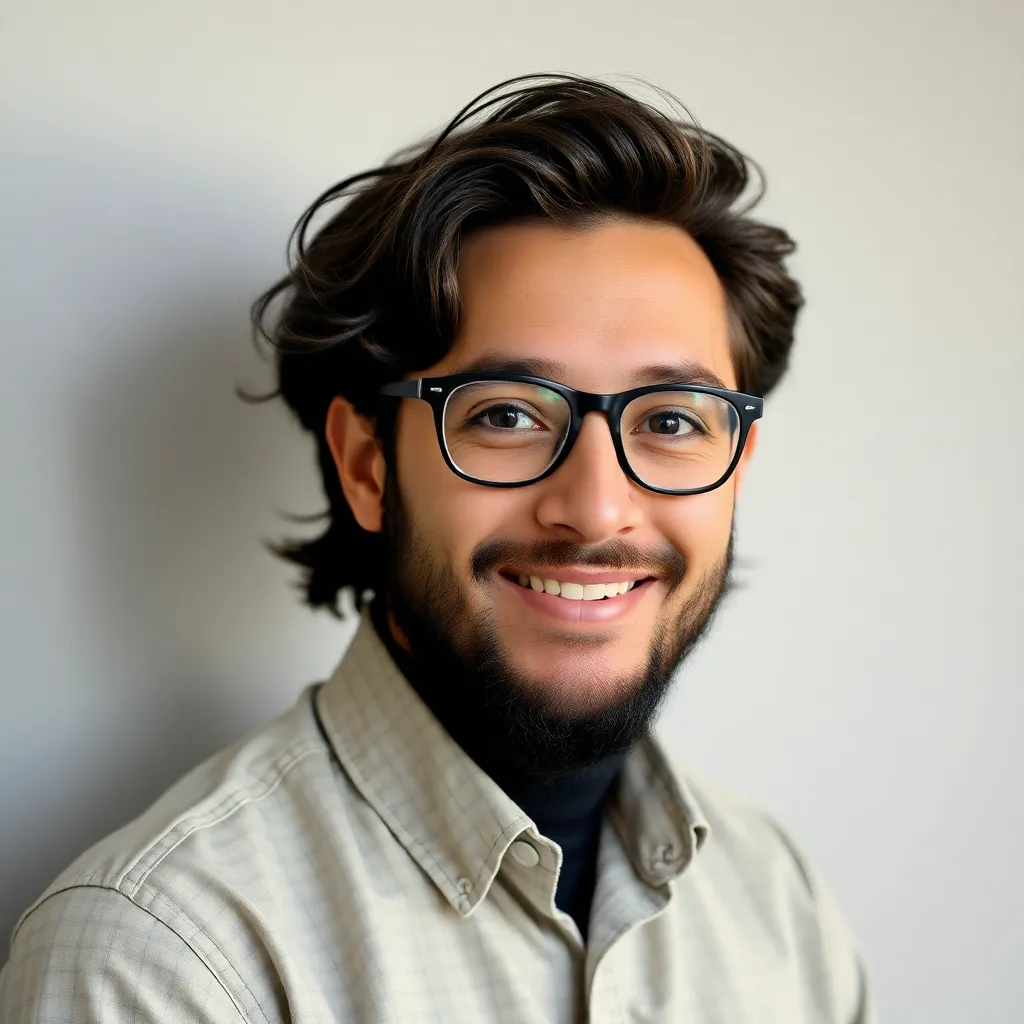
Holbox
Apr 08, 2025 · 7 min read
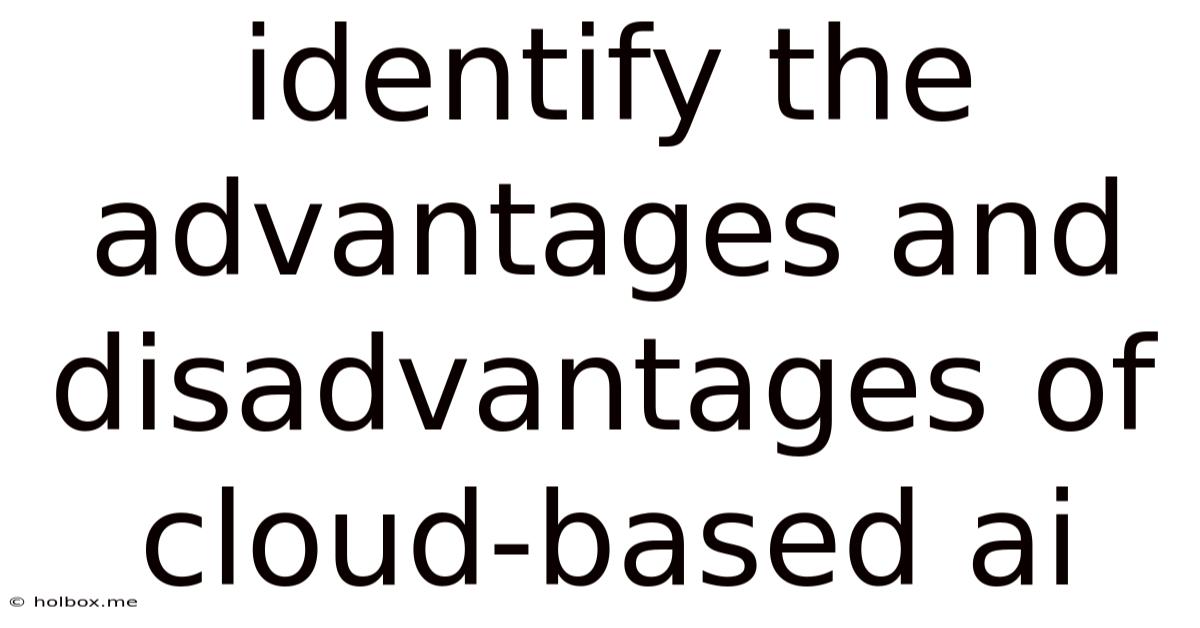
Table of Contents
- Identify The Advantages And Disadvantages Of Cloud-based Ai
- Table of Contents
- Identify the Advantages and Disadvantages of Cloud-Based AI
- Advantages of Cloud-Based AI
- 1. Scalability and Flexibility: Effortless Growth
- 2. Cost-Effectiveness: Optimized Resource Allocation
- 3. Accessibility and Collaboration: Breaking Down Silos
- 4. Enhanced Security and Data Protection: Robust Infrastructure
- 5. Access to Advanced Technologies and Tools: Cutting-Edge Innovation
- Disadvantages of Cloud-Based AI
- 1. Internet Dependency: The Need for Reliable Connectivity
- 2. Vendor Lock-in: The Challenge of Switching Providers
- 3. Security Concerns: Data Breaches and Vulnerabilities
- 4. Latency Issues: The Impact on Real-Time Applications
- 5. Cost Management: Unexpected Expenses and Overspending
- 6. Data Privacy and Compliance: Navigating Regulations
- Choosing the Right Approach: Balancing Advantages and Disadvantages
- Latest Posts
- Latest Posts
- Related Post
Identify the Advantages and Disadvantages of Cloud-Based AI
The rise of artificial intelligence (AI) is transforming industries, and cloud computing is playing a pivotal role in this revolution. Cloud-based AI, the deployment of AI algorithms and models on cloud infrastructure, offers a compelling blend of scalability, cost-effectiveness, and accessibility. However, it also presents unique challenges and drawbacks. This article delves into a comprehensive analysis of the advantages and disadvantages of cloud-based AI, providing a balanced perspective for businesses and individuals considering its adoption.
Advantages of Cloud-Based AI
Cloud-based AI offers numerous benefits that make it an attractive option for organizations of all sizes. Let's examine these advantages in detail:
1. Scalability and Flexibility: Effortless Growth
One of the most significant advantages of cloud-based AI is its inherent scalability. Unlike on-premise AI solutions which require significant upfront investment in hardware and infrastructure, cloud-based AI allows businesses to easily scale their resources up or down based on their needs. This means that you can start small and gradually increase your computational power as your AI projects grow, without the need for costly hardware upgrades or replacements. This flexibility is crucial for businesses facing fluctuating demands or experiencing rapid growth. Need more processing power for a complex machine learning task? Simply provision it on demand. Need less? Reduce your allocation just as easily, minimizing wasted resources and optimizing costs.
2. Cost-Effectiveness: Optimized Resource Allocation
The pay-as-you-go model of cloud computing translates directly to cost savings in AI deployment. You only pay for the resources you consume, eliminating the need for large upfront investments in expensive hardware, software licenses, and IT personnel dedicated to managing on-premise infrastructure. This eliminates the burden of maintaining and updating hardware, saving on maintenance costs, energy consumption, and IT support. The cloud provider handles all the infrastructure management, allowing your team to focus on developing and deploying your AI models.
3. Accessibility and Collaboration: Breaking Down Silos
Cloud-based AI fosters collaboration and accessibility like never before. Teams can access and work on AI projects from anywhere with an internet connection, regardless of their physical location. This fosters seamless collaboration between geographically dispersed teams and accelerates project development. Data scientists can easily share models, datasets, and results, breaking down data silos and improving overall efficiency. The shared environment also facilitates knowledge sharing and team learning, fostering a more collaborative and productive AI development process.
4. Enhanced Security and Data Protection: Robust Infrastructure
Reputable cloud providers invest heavily in robust security measures to protect your data and AI models. Their state-of-the-art security protocols, including encryption, access control, and threat detection systems, often surpass what many organizations can achieve on their own. This is particularly crucial when dealing with sensitive data used in AI applications. However, it's crucial to carefully select a provider with a strong security track record and understand the security features offered within their services. Due diligence in this area is paramount to protect your valuable data and intellectual property.
5. Access to Advanced Technologies and Tools: Cutting-Edge Innovation
Cloud platforms provide access to a wide array of advanced AI tools, libraries, and pre-trained models. This accelerates AI development by eliminating the need to build everything from scratch. This includes access to powerful machine learning frameworks like TensorFlow and PyTorch, pre-trained models for various tasks, and specialized hardware like GPUs and TPUs, all accessible through the cloud. This readily available infrastructure significantly lowers the barrier to entry for AI development, enabling even small companies to leverage the power of cutting-edge AI technologies.
Disadvantages of Cloud-Based AI
While cloud-based AI offers many advantages, it also presents certain challenges and drawbacks that need careful consideration:
1. Internet Dependency: The Need for Reliable Connectivity
Cloud-based AI systems rely heavily on a stable and reliable internet connection. Without consistent connectivity, access to your AI models, data, and computational resources is severely compromised, potentially disrupting operations and impacting performance. This dependency can be a significant drawback in areas with limited or unreliable internet infrastructure. Downtime can result in lost productivity, data breaches, and project delays. Therefore, a robust and redundant internet connection is critical for successful cloud-based AI deployment.
2. Vendor Lock-in: The Challenge of Switching Providers
Migrating AI solutions from one cloud provider to another can be a complex and costly undertaking. The proprietary nature of some cloud services and the integration of AI models with specific cloud platforms can create vendor lock-in, making it difficult and expensive to switch providers later on. This can limit your flexibility and potentially reduce your negotiating power with your cloud provider over time. Thorough planning and choosing a provider with robust migration capabilities is critical to mitigate this risk.
3. Security Concerns: Data Breaches and Vulnerabilities
While cloud providers invest heavily in security, the risk of data breaches and security vulnerabilities always exists. The potential for data loss, unauthorized access, or malicious attacks is a valid concern, particularly when dealing with sensitive data. Furthermore, reliance on a third-party provider means relinquishing some degree of control over your data security and requiring trust in the provider's security measures. Careful due diligence, including thorough vetting of the provider’s security practices and compliance certifications, is crucial to mitigate this risk.
4. Latency Issues: The Impact on Real-Time Applications
Latency, the delay in data transmission, can be a significant issue for cloud-based AI, particularly in real-time applications like autonomous driving or robotics. High latency can result in delayed responses or inaccurate predictions, compromising the performance and effectiveness of the AI system. Minimizing latency requires careful selection of cloud regions geographically close to the application's deployment location and optimization of data transfer mechanisms.
5. Cost Management: Unexpected Expenses and Overspending
While cloud-based AI offers cost-effectiveness, unexpected expenses can arise from inefficient resource allocation or unforeseen spikes in usage. Without careful monitoring and optimization of resource consumption, costs can quickly escalate. Understanding the pricing models of cloud providers, implementing effective cost management strategies, and regularly monitoring resource usage are crucial for controlling expenses and avoiding cost overruns. Detailed cost analysis and proactive planning are essential to avoid unexpected bills.
6. Data Privacy and Compliance: Navigating Regulations
Compliance with data privacy regulations, such as GDPR or CCPA, is crucial when using cloud-based AI. Understanding the data privacy policies and security certifications of your chosen cloud provider is essential to ensure compliance. Failure to comply with these regulations can lead to significant fines and reputational damage. Carefully review the provider's compliance certifications and data handling policies to ensure they align with your regulatory requirements.
Choosing the Right Approach: Balancing Advantages and Disadvantages
The decision of whether to use cloud-based AI depends on various factors, including the specific application, budget, available resources, and risk tolerance. For organizations with limited resources or those seeking scalability and cost-effectiveness, cloud-based AI presents a compelling option. However, organizations with stringent security requirements, real-time constraints, or concerns about vendor lock-in might need to consider alternative approaches.
A hybrid approach, combining cloud-based AI with on-premise solutions, might be the optimal strategy for some organizations. This approach allows them to leverage the advantages of cloud-based AI for scalable and cost-effective processing while maintaining control over sensitive data and critical infrastructure.
Ultimately, a thorough understanding of both the advantages and disadvantages of cloud-based AI is crucial for making informed decisions and successfully implementing AI solutions that meet your organizational needs. Careful planning, due diligence, and a clear understanding of your requirements are critical to maximizing the benefits and mitigating the risks of cloud-based AI. By thoughtfully weighing the pros and cons and adapting your approach accordingly, you can harness the transformative power of cloud-based AI while minimizing its potential drawbacks.
Latest Posts
Latest Posts
-
Identify Which Balance Was Used To Make Each Mass Reading
Apr 26, 2025
-
When Was The Aphid Population Growing Fastest
Apr 26, 2025
-
Molecular Clocks Are Based On The Idea That
Apr 26, 2025
-
The Latest Start Of An Activity Is The
Apr 26, 2025
-
The Hydroelectric Turbine Shown In Fig
Apr 26, 2025
Related Post
Thank you for visiting our website which covers about Identify The Advantages And Disadvantages Of Cloud-based Ai . We hope the information provided has been useful to you. Feel free to contact us if you have any questions or need further assistance. See you next time and don't miss to bookmark.