Data Analytics Simulation Strategic Decision Making
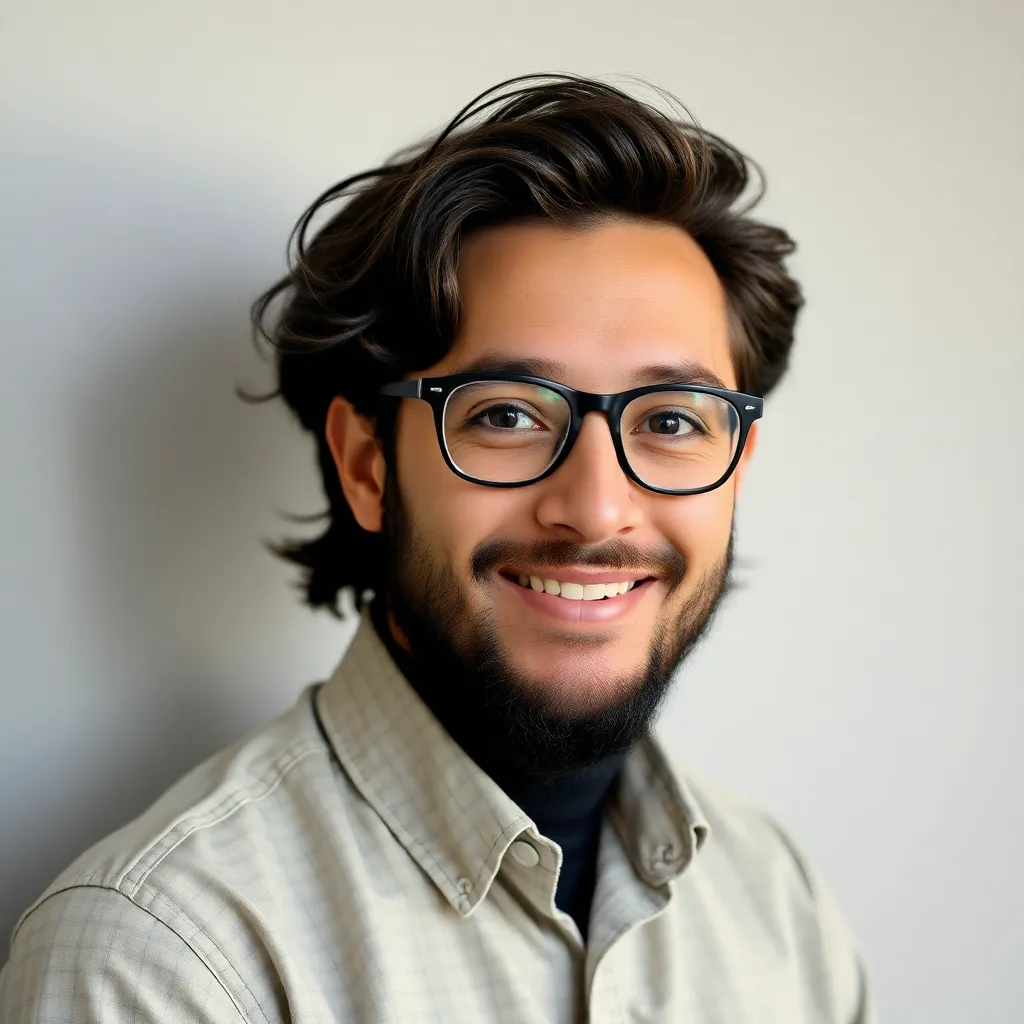
Holbox
Apr 06, 2025 · 6 min read

Table of Contents
- Data Analytics Simulation Strategic Decision Making
- Table of Contents
- Data Analytics Simulation for Strategic Decision Making: A Deep Dive
- Understanding Data Analytics Simulation
- Key Components of a Data Analytics Simulation
- Benefits of Using Data Analytics Simulation in Strategic Decision Making
- Methodologies for Data Analytics Simulation
- Challenges in Implementing Data Analytics Simulation
- Future Trends in Data Analytics Simulation for Strategic Decision Making
- Conclusion
- Latest Posts
- Latest Posts
- Related Post
Data Analytics Simulation for Strategic Decision Making: A Deep Dive
The business world is increasingly data-driven. Strategic decision-making, once reliant on intuition and gut feeling, now heavily leverages data analytics. However, applying data analysis to real-world scenarios can be risky. This is where data analytics simulation comes into play. This powerful tool allows businesses to test different strategies, analyze their potential outcomes, and make more informed, data-backed decisions before committing resources in the real world. This article delves into the crucial role of data analytics simulation in strategic decision-making, exploring its benefits, methodologies, challenges, and future trends.
Understanding Data Analytics Simulation
Data analytics simulation is a technique that uses computer models to mimic real-world business processes. By inputting historical data, market trends, and various assumptions, analysts can simulate different scenarios and predict potential outcomes. This allows for a risk-free environment to experiment with various strategies, optimize operations, and ultimately improve decision-making. It's essentially a virtual sandbox where you can test hypotheses without risking real-world consequences.
Key Components of a Data Analytics Simulation
Several key components contribute to the success of a data analytics simulation:
-
Data Collection and Preparation: High-quality data is crucial. This involves gathering relevant historical data, cleaning it to remove inaccuracies and inconsistencies, and transforming it into a usable format for the simulation model. This stage often involves techniques like data mining and data cleansing.
-
Model Development: The heart of the simulation lies in building a realistic model. This could involve statistical models, agent-based modeling, system dynamics modeling, or a combination of approaches. The choice of model depends on the complexity of the business problem and the available data.
-
Scenario Definition: Analysts need to define the specific scenarios they want to simulate. This involves identifying key variables, defining their ranges, and specifying the relationships between them. For example, in a marketing simulation, scenarios could include varying advertising budgets, different pricing strategies, and changes in competitor actions.
-
Simulation Execution and Analysis: Once the model is built and scenarios defined, the simulation is run. The results are then analyzed to identify trends, patterns, and potential outcomes. This may involve statistical analysis, visualization techniques, and sensitivity analysis to understand the impact of different variables.
-
Decision Support: The final stage is using the insights generated from the simulation to inform strategic decisions. This involves interpreting the results, considering their limitations, and integrating them with other sources of information to make well-informed decisions.
Benefits of Using Data Analytics Simulation in Strategic Decision Making
The advantages of incorporating data analytics simulation into strategic decision-making are numerous and impactful:
-
Reduced Risk: By testing strategies in a simulated environment, businesses can minimize the risks associated with implementing new initiatives in the real world. This is particularly crucial for high-stakes decisions with potentially significant financial or reputational consequences.
-
Improved Forecasting Accuracy: Simulations can provide more accurate forecasts compared to traditional methods. By incorporating various factors and their interactions, simulations provide a holistic view of potential outcomes.
-
Enhanced Resource Allocation: Simulations can help optimize resource allocation by identifying the most effective strategies for achieving specific goals. This leads to better utilization of resources and improved efficiency.
-
Faster Decision Making: Simulations enable faster decision-making by providing quick insights into the potential outcomes of different strategies. This can be critical in rapidly changing market conditions.
-
Competitive Advantage: Businesses that leverage data analytics simulation can gain a significant competitive advantage by making more informed and strategic decisions. This can lead to increased market share, profitability, and sustained growth.
-
Better Understanding of Complex Systems: Simulations provide a powerful tool for understanding the dynamics of complex systems and the interactions between various factors. This helps in developing more effective and resilient strategies.
-
Improved Collaboration and Communication: Simulations can facilitate better collaboration and communication among stakeholders by providing a shared platform for exploring different scenarios and discussing their potential implications.
Methodologies for Data Analytics Simulation
Various methodologies can be employed for data analytics simulation, each with its strengths and weaknesses:
-
Monte Carlo Simulation: This probabilistic method uses random sampling to generate a large number of possible outcomes. It's particularly useful for analyzing scenarios with uncertainty and variability.
-
Agent-Based Modeling (ABM): This approach simulates the behavior of individual agents (e.g., customers, employees, competitors) and their interactions within a system. It's useful for understanding emergent behavior and complex systems.
-
System Dynamics Modeling: This methodology focuses on the feedback loops and dynamic relationships within a system. It's useful for understanding long-term trends and the impact of policy changes.
-
Discrete Event Simulation (DES): This technique simulates the occurrence of events over time. It's useful for modeling systems with distinct events, such as customer arrivals in a queue or machine breakdowns in a manufacturing plant.
The choice of methodology depends on the specific business problem, the available data, and the desired level of detail.
Challenges in Implementing Data Analytics Simulation
Despite its numerous benefits, implementing data analytics simulation presents some challenges:
-
Data Availability and Quality: High-quality and sufficient data is essential for building accurate and reliable simulations. Data scarcity or poor data quality can limit the effectiveness of the simulation.
-
Model Complexity: Developing realistic and accurate simulation models can be complex and time-consuming. It requires expertise in statistical modeling, programming, and domain knowledge.
-
Computational Resources: Running complex simulations can require significant computational resources, which can be costly.
-
Validation and Verification: Ensuring the accuracy and reliability of the simulation model is crucial. This involves validating the model against real-world data and verifying its internal consistency.
-
Interpretation of Results: Interpreting the results of a simulation can be challenging, particularly for complex models. It requires careful consideration of the assumptions made and the limitations of the model.
Future Trends in Data Analytics Simulation for Strategic Decision Making
The field of data analytics simulation is constantly evolving, with several exciting future trends emerging:
-
Increased Use of Artificial Intelligence (AI) and Machine Learning (ML): AI and ML are being increasingly incorporated into simulation models to improve their accuracy, efficiency, and adaptability. This includes using AI for model calibration, prediction, and optimization.
-
Integration with Cloud Computing: Cloud computing platforms offer scalable and cost-effective solutions for running complex simulations. This allows businesses to leverage the power of simulation without significant upfront investment in hardware.
-
Development of More User-Friendly Simulation Tools: The development of user-friendly simulation tools is making simulation accessible to a wider range of users, including those without specialized technical expertise.
-
Greater Emphasis on Real-Time Simulation: Real-time simulation capabilities are becoming increasingly important for making timely decisions in dynamic environments. This allows for continuous monitoring and adjustment of strategies based on real-time data.
-
Expansion into New Application Areas: Data analytics simulation is being applied to a growing range of business problems, including supply chain optimization, risk management, and financial forecasting.
Conclusion
Data analytics simulation is a powerful tool that can significantly enhance strategic decision-making. By providing a safe and controlled environment to test different strategies, it enables businesses to make more informed, data-driven decisions, reducing risks, improving efficiency, and gaining a competitive advantage. While challenges remain, the continuous advancements in technology and methodologies promise to make data analytics simulation even more accessible and impactful in the years to come. Embracing this powerful technique is crucial for businesses aiming to thrive in today's increasingly complex and competitive environment. The future of strategic decision-making is inextricably linked to the effective application of data analytics simulation.
Latest Posts
Latest Posts
-
How Can You Refine Your Content Distribution Strategy
Apr 14, 2025
-
Density Of Water In Lb In3
Apr 14, 2025
-
How Are Electric Field And Temperature Field Different
Apr 14, 2025
-
Decide State Equivalence With Implication Table
Apr 14, 2025
-
Which Term Best Describes The Angle Below
Apr 14, 2025
Related Post
Thank you for visiting our website which covers about Data Analytics Simulation Strategic Decision Making . We hope the information provided has been useful to you. Feel free to contact us if you have any questions or need further assistance. See you next time and don't miss to bookmark.