Construct A 95 Confidence Interval For The Population Mean
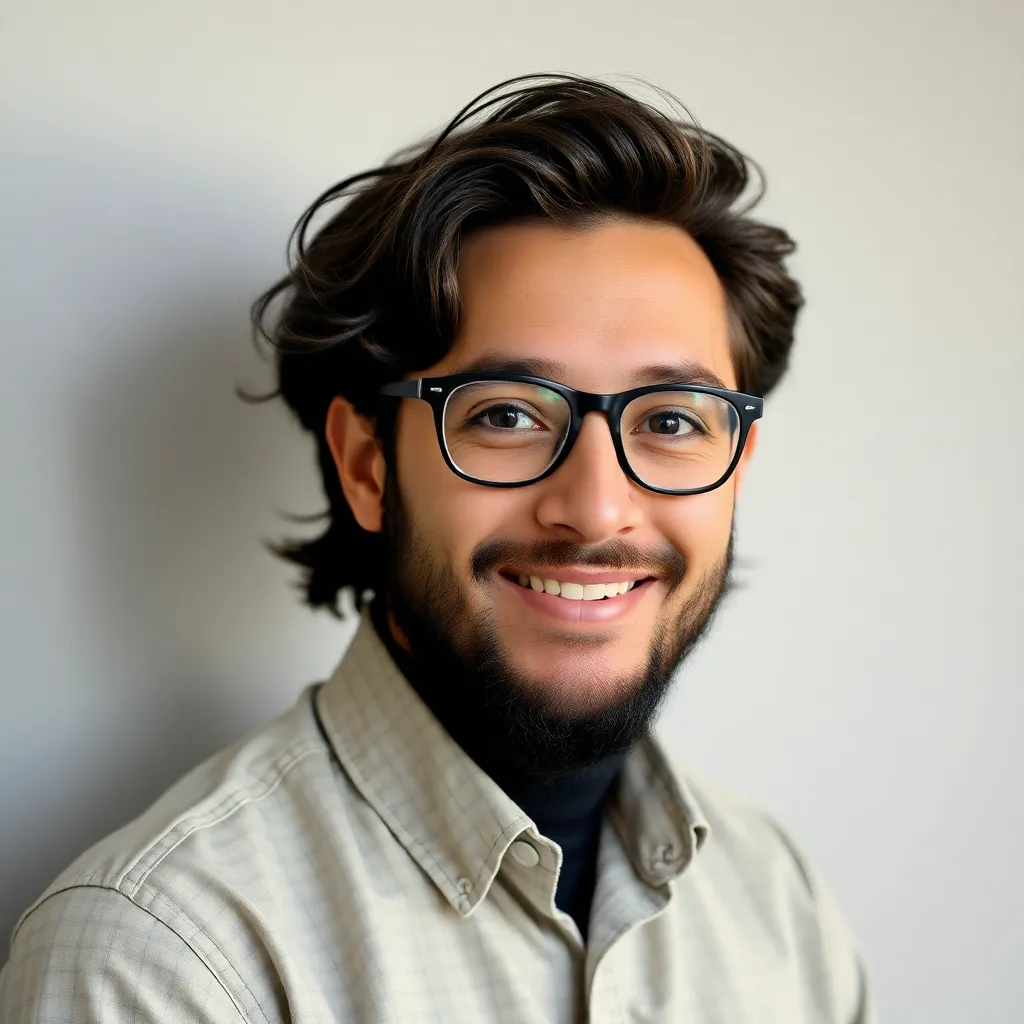
Holbox
Mar 27, 2025 · 5 min read

Table of Contents
- Construct A 95 Confidence Interval For The Population Mean
- Table of Contents
- Constructing a 95% Confidence Interval for the Population Mean
- What is a Confidence Interval?
- Assumptions Before Calculation
- Calculating the 95% Confidence Interval: Known Population Standard Deviation
- Calculating the 95% Confidence Interval: Unknown Population Standard Deviation
- Interpreting the Confidence Interval
- Factors Affecting Confidence Interval Width
- Using Statistical Software
- Conclusion
- Latest Posts
- Latest Posts
- Related Post
Constructing a 95% Confidence Interval for the Population Mean
Understanding and constructing confidence intervals is a fundamental concept in statistics. This article will delve into the specifics of constructing a 95% confidence interval for the population mean, explaining the underlying principles, the necessary calculations, and the crucial interpretations. We'll cover scenarios with both known and unknown population standard deviations.
What is a Confidence Interval?
A confidence interval provides a range of values within which we are confident that the true population parameter (in this case, the population mean) lies. A 95% confidence interval means that if we were to repeat the sampling process many times, 95% of the calculated intervals would contain the true population mean. It's crucial to understand that this doesn't mean there's a 95% chance the true mean lies within this specific interval; the true mean is either within the interval or it isn't. The 95% refers to the long-run frequency of intervals capturing the true mean.
Assumptions Before Calculation
Before diving into the calculations, several assumptions need to be met for the confidence interval to be valid:
- Random Sampling: The data must be obtained through a simple random sample. This ensures that each member of the population has an equal chance of being selected, minimizing bias.
- Independence: Observations within the sample must be independent of each other. This means the selection of one data point doesn't influence the selection of another.
- Normality: The sampling distribution of the sample mean should be approximately normal. This is often satisfied by the Central Limit Theorem, which states that the distribution of sample means approaches normality as the sample size increases (generally, n ≥ 30). However, for smaller samples, the underlying population should be normally distributed or the data should be reasonably symmetric with no extreme outliers.
Calculating the 95% Confidence Interval: Known Population Standard Deviation
When the population standard deviation (σ) is known, the calculation is straightforward. The formula for a 95% confidence interval is:
CI = x̄ ± Z * (σ / √n)
Where:
- CI: Represents the confidence interval.
- x̄: Is the sample mean (the average of your data).
- Z: Is the Z-score corresponding to the desired confidence level (for 95%, Z = 1.96). This value comes from the standard normal distribution table.
- σ: Is the population standard deviation.
- n: Is the sample size.
Example:
Let's say we have a sample of 100 light bulbs, and we want to estimate the average lifespan. We know the population standard deviation (σ) is 100 hours, and our sample mean (x̄) is 1000 hours.
-
Calculate the margin of error: Margin of error = Z * (σ / √n) = 1.96 * (100 / √100) = 19.6 hours
-
Construct the confidence interval: CI = x̄ ± Margin of Error = 1000 ± 19.6 = (980.4, 1019.6) hours
Therefore, we are 95% confident that the true average lifespan of the light bulbs lies between 980.4 and 1019.6 hours.
Calculating the 95% Confidence Interval: Unknown Population Standard Deviation
In most real-world scenarios, the population standard deviation is unknown. In such cases, we estimate it using the sample standard deviation (s). This necessitates using the t-distribution instead of the Z-distribution. The formula becomes:
CI = x̄ ± t * (s / √n)
Where:
- x̄: Is the sample mean.
- t: Is the t-score corresponding to the desired confidence level and degrees of freedom (df = n - 1). You'll need a t-table or statistical software to find this value.
- s: Is the sample standard deviation.
- n: Is the sample size.
Example:
Suppose we're studying the average weight of adult dogs of a certain breed. We have a sample of 25 dogs, with a sample mean (x̄) of 25 kg and a sample standard deviation (s) of 3 kg.
-
Determine the degrees of freedom: df = n - 1 = 25 - 1 = 24
-
Find the t-score: For a 95% confidence interval and 24 degrees of freedom, the t-score (from a t-table) is approximately 2.064.
-
Calculate the margin of error: Margin of error = t * (s / √n) = 2.064 * (3 / √25) = 1.238 kg
-
Construct the confidence interval: CI = x̄ ± Margin of Error = 25 ± 1.238 = (23.762, 26.238) kg
Therefore, we are 95% confident that the true average weight of adult dogs of this breed lies between 23.762 kg and 26.238 kg.
Interpreting the Confidence Interval
The interpretation of the confidence interval is crucial. It's not about the probability of the true mean being in a specific interval. Instead, it's about the long-run performance of the method. If we were to repeat this process many times, obtaining numerous samples and calculating confidence intervals for each, approximately 95% of those intervals would contain the true population mean.
Factors Affecting Confidence Interval Width
Several factors influence the width of the confidence interval:
-
Confidence Level: A higher confidence level (e.g., 99%) leads to a wider interval because we need to be more certain that the true mean is captured.
-
Sample Size: A larger sample size leads to a narrower interval because larger samples provide more precise estimates of the population mean. The relationship is inverse; as the square root of n increases, the margin of error decreases.
-
Population Standard Deviation (or Sample Standard Deviation): A larger standard deviation (reflecting greater variability in the data) results in a wider interval, indicating more uncertainty in the estimate of the population mean.
Using Statistical Software
Statistical software packages like R, SPSS, Python (with libraries like SciPy and Statsmodels), and Excel can greatly simplify the calculation of confidence intervals. These programs automate the calculations and often provide additional information, such as p-values and standard errors.
Conclusion
Constructing a 95% confidence interval for the population mean is a vital statistical procedure for estimating the range within which the true mean likely lies. The choice between using the Z-distribution or the t-distribution depends on whether the population standard deviation is known. Understanding the underlying assumptions, the calculation process, and the correct interpretation of the results are critical for making informed inferences about population parameters based on sample data. Remember that the confidence interval provides a range of plausible values, not a definitive statement about the exact value of the population mean. Always consider the context of your data and the limitations of your sample when interpreting the results. The use of statistical software can significantly streamline the process, allowing for more efficient analysis and interpretation of your results. This detailed understanding of confidence intervals is essential for anyone involved in data analysis and interpretation across various fields.
Latest Posts
Latest Posts
-
In The Term Agranulocyte What Does The Prefix Mean
Mar 30, 2025
-
Why Are Currency And Checkable Deposits Money
Mar 30, 2025
-
A Monopolist Does Not Have A Supply Curve Because
Mar 30, 2025
-
1 3 Butadiene Undergoes An Electrophilic Addition With Hbr
Mar 30, 2025
-
When We Say That Momentum Is Conserved We Mean
Mar 30, 2025
Related Post
Thank you for visiting our website which covers about Construct A 95 Confidence Interval For The Population Mean . We hope the information provided has been useful to you. Feel free to contact us if you have any questions or need further assistance. See you next time and don't miss to bookmark.