Big Data Is Characterized By Each Of These Except
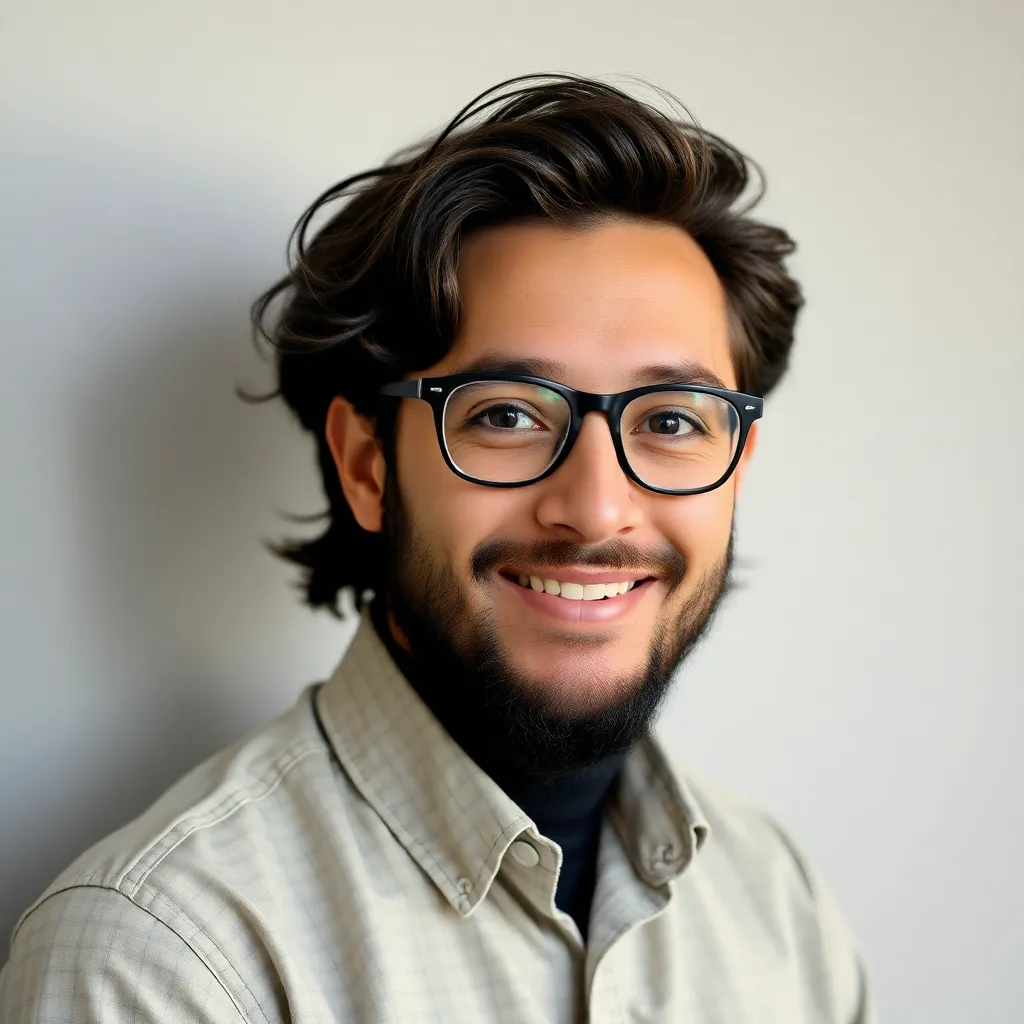
Holbox
Apr 26, 2025 · 7 min read
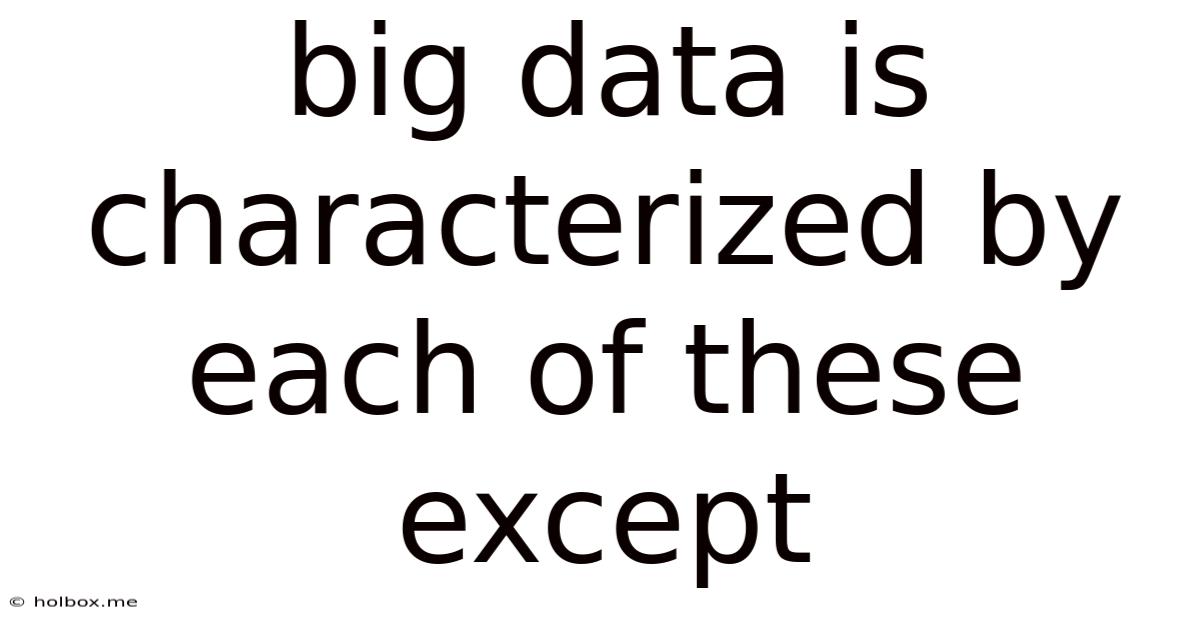
Table of Contents
- Big Data Is Characterized By Each Of These Except
- Table of Contents
- Big Data is Characterized by Each of These Except… Volatility
- The Five Vs of Big Data: A Deep Dive
- 1. Volume: The Sheer Scale of Data
- 2. Velocity: The Speed of Data Generation and Processing
- 3. Variety: The Diverse Forms of Data
- 4. Veracity: The Trustworthiness of Data
- 5. Value: The Potential for Actionable Insights
- Why Volatility is NOT a Defining Characteristic
- The Importance of Context: Volatility vs. Velocity
- Handling Volatility within Big Data
- Conclusion: Understanding the True Nature of Big Data
- Latest Posts
- Latest Posts
- Related Post
Big Data is Characterized by Each of These Except… Volatility
Big data, a term thrown around frequently in today's technological landscape, refers to extremely large and complex datasets that are difficult to process using traditional data processing applications. These datasets are characterized by several key attributes often remembered by the acronym “Volume, Velocity, Variety, Veracity, and Value.” But what about the outlier? What characteristic is not typically associated with big data? The answer is volatility. While data can certainly change rapidly, volatility itself isn't a defining characteristic of big data. Let's delve deeper into this and explore the five Vs of big data in detail, highlighting why volatility doesn't fit the bill.
The Five Vs of Big Data: A Deep Dive
Understanding the core characteristics of big data is crucial for effectively leveraging its potential. The five Vs—Volume, Velocity, Variety, Veracity, and Value—provide a comprehensive framework for analyzing and managing big data.
1. Volume: The Sheer Scale of Data
This refers to the massive size of big data. We're talking terabytes, petabytes, and even exabytes of data—far exceeding the capabilities of traditional data management tools. Think about the data generated by social media platforms, online transactions, sensor networks, and scientific experiments. The sheer volume demands specialized infrastructure and processing techniques to handle and analyze it effectively. The scale necessitates parallel processing, distributed databases, and cloud computing solutions. Without the ability to manage this extreme volume, extracting meaningful insights becomes nearly impossible.
2. Velocity: The Speed of Data Generation and Processing
Velocity refers to the speed at which data is generated and processed. In today's fast-paced digital world, data streams in at an incredible rate from various sources. Think of real-time sensor data from connected devices, high-frequency trading data, and social media feeds. This rapid influx of data necessitates real-time or near real-time processing capabilities to extract actionable insights before the data becomes outdated. Traditional batch processing methods simply can't keep up with this speed, hence the requirement for advanced streaming analytics and real-time data processing solutions. Analyzing this velocity efficiently is critical for timely decision-making.
3. Variety: The Diverse Forms of Data
Big data comes in a wide variety of formats—structured, semi-structured, and unstructured. Structured data, neatly organized into tables and rows, is relatively easy to manage. Semi-structured data, like JSON and XML, offers some organizational structure but lacks the rigidity of structured data. Unstructured data, the most challenging type, includes text, images, audio, and video files—lacking any predefined format. The ability to handle this diversity is a core challenge and opportunity with big data, demanding the use of diverse analytical techniques tailored to specific data types. Effectively combining insights from different data types often reveals richer and more insightful patterns.
4. Veracity: The Trustworthiness of Data
Veracity refers to the accuracy, reliability, and trustworthiness of the data. Big data sets often contain inconsistencies, errors, and inaccuracies. This poses a significant challenge as unreliable data can lead to flawed analyses and poor decision-making. Data cleaning, validation, and verification processes are critical to ensure the veracity of the data before analysis. The quality of insights directly correlates to the trustworthiness of the data used, hence the emphasis on accurate and reliable sources. Techniques like data cleansing, data governance, and robust data validation procedures are crucial in establishing data veracity.
5. Value: The Potential for Actionable Insights
Ultimately, the value of big data lies in its potential to generate actionable insights. By analyzing large and diverse datasets, organizations can uncover trends, patterns, and anomalies that would be impossible to identify using traditional methods. These insights can be used to improve operational efficiency, enhance customer experience, develop new products and services, and gain a competitive advantage. Extracting value, however, isn't automatic; it requires sophisticated analytical techniques, skilled data scientists, and a clear understanding of the business problem being addressed. The true measure of big data success is not simply in the volume or velocity of data but in the valuable insights it unlocks and the actions that result.
Why Volatility is NOT a Defining Characteristic
While big data is often dynamic and rapidly changing, volatility itself is not a core defining characteristic. While data within a big data set might be volatile—constantly updated, modified, or replaced—the fundamental characteristics of volume, velocity, variety, veracity, and value remain consistent. The sheer size (volume), the speed of generation (velocity), the diversity of formats (variety), the need for accuracy (veracity), and the potential for valuable insights (value) all persist regardless of how frequently the data changes. Volatility is a characteristic that can be present in big data, but it's not what fundamentally defines it. You could have a massive dataset (high volume) updated slowly (low velocity), still qualifying as big data despite lacking significant volatility.
The Importance of Context: Volatility vs. Velocity
It’s crucial to distinguish volatility from velocity. While both relate to the dynamism of data, they differ significantly. Velocity focuses on the rate of data inflow, how quickly new data is generated and added to the dataset. Volatility, on the other hand, focuses on the rate of data change within the dataset itself. A highly volatile dataset might have frequent updates and modifications to existing data points, even if the overall rate of new data inflow is relatively low. Conversely, a dataset with high velocity might have a relatively stable composition, with minimal changes to existing data after it's been ingested.
Therefore, a high-velocity dataset doesn’t necessarily imply high volatility, and vice-versa. A high-frequency trading database, for example, has extremely high velocity (constant stream of new trade data), but individual data points (once recorded) might not change significantly, resulting in lower volatility. Conversely, a social media sentiment analysis dataset might have lower velocity (less frequent data updates), yet be highly volatile because individual sentiments might change rapidly based on new events or trends.
Handling Volatility within Big Data
Although not a defining characteristic, dealing with volatile data within big data contexts is crucial. Several techniques are employed to handle the challenges presented by rapidly changing data:
-
Real-time processing: Techniques like stream processing allow for immediate analysis of data as it's generated, ensuring that insights are based on the most up-to-date information.
-
Change data capture (CDC): This method tracks and captures only the changes made to data, rather than processing the entire dataset, improving efficiency when dealing with frequent updates.
-
Incremental updates: Instead of completely replacing the entire dataset with each update, incremental updates only incorporate the changes, reducing processing time and resource consumption.
-
Version control: Maintaining version history allows tracking data changes over time, enabling rollback capabilities if needed and facilitating analysis of data evolution.
-
Event sourcing: This architectural pattern stores all data as a sequence of events, allowing for reconstruction of the dataset's state at any point in time, useful for auditing and analysis of historical changes.
Conclusion: Understanding the True Nature of Big Data
Big data is a complex phenomenon, defined by its scale, speed, variety, veracity, and, most importantly, its potential value. While data within a big data system can be highly volatile, volatility itself is not a fundamental defining attribute. Understanding the distinctions between velocity and volatility, and employing appropriate data management and processing techniques, is critical for effectively harnessing the power of big data and extracting meaningful insights from even the most dynamic datasets. By focusing on the five Vs and addressing the challenges posed by data dynamism, organizations can leverage big data to gain a competitive edge and drive informed decision-making in today's rapidly evolving digital world. The real challenge lies not just in managing the volume, velocity, and variety, but in ensuring the veracity and, ultimately, unlocking the true value hidden within those massive datasets.
Latest Posts
Latest Posts
-
When A Firm Experiences Diseconomies Of Scale
May 07, 2025
-
Deploy Verify Monitor And Respond Are All Activities Of What
May 07, 2025
-
Identify The Correct Molecular Formula For The Illustrated Compound
May 07, 2025
-
Process Costing Is Not Used When
May 07, 2025
-
The Foundation Of Any Organizational Culture Is Based On
May 07, 2025
Related Post
Thank you for visiting our website which covers about Big Data Is Characterized By Each Of These Except . We hope the information provided has been useful to you. Feel free to contact us if you have any questions or need further assistance. See you next time and don't miss to bookmark.