Assesses The Consistency Of Observations By Different Observers
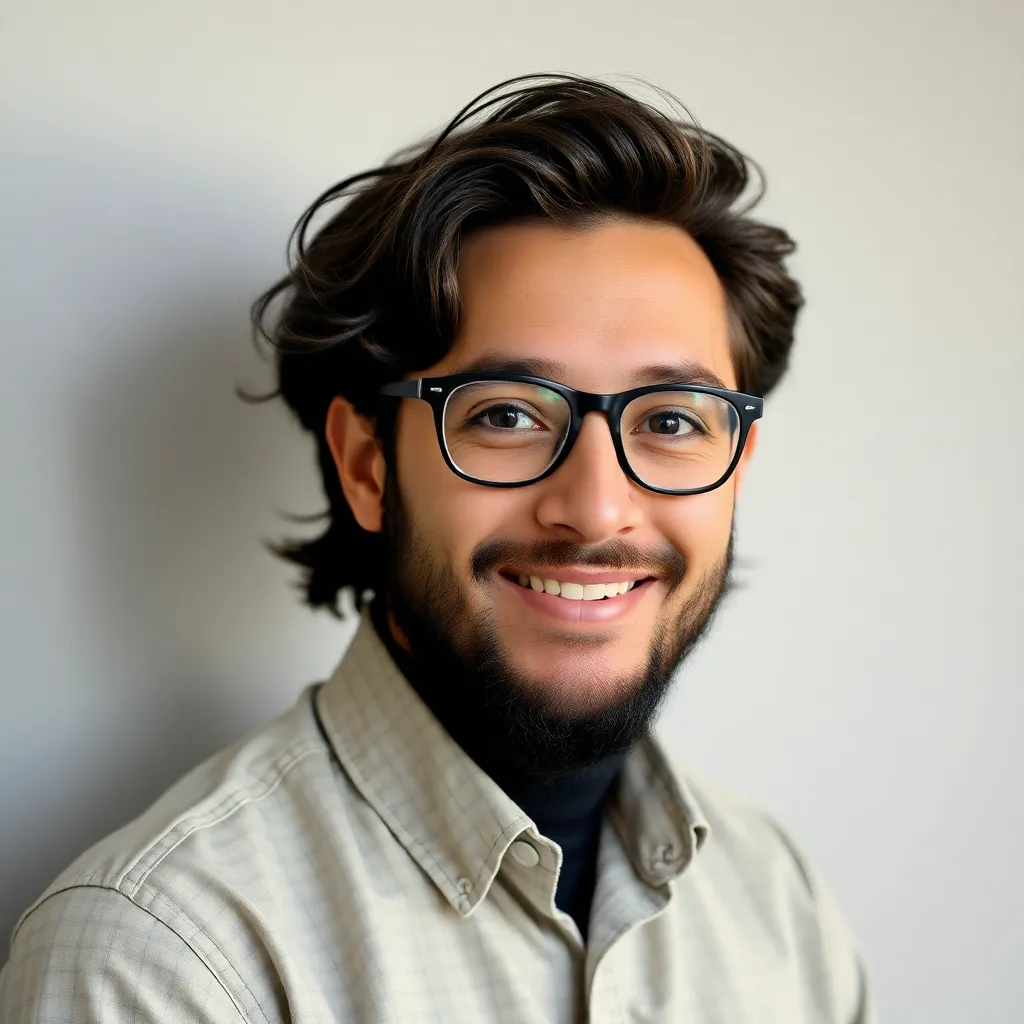
Holbox
Mar 31, 2025 · 7 min read
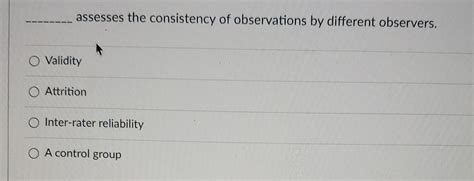
Table of Contents
- Assesses The Consistency Of Observations By Different Observers
- Table of Contents
- Assessing the Consistency of Observations by Different Observers: A Deep Dive into Inter-rater Reliability
- The Importance of Inter-rater Reliability
- Methods for Assessing Inter-rater Reliability
- 1. Percent Agreement: A Simple Start
- 2. Cohen's Kappa: Accounting for Chance Agreement
- 3. Fleiss' Kappa: For Multiple Raters
- 4. Intraclass Correlation Coefficient (ICC): For Continuous Data
- 5. Weighted Kappa: Addressing the Severity of Disagreement
- Strategies for Improving Inter-rater Reliability
- 1. Clear and Detailed Training: Setting the Standard
- 2. Standardized Procedures: Maintaining Consistency
- 3. Regular Calibration and Feedback: Continuous Improvement
- 4. Selecting Appropriate Measurement Tools: Using the Right Instruments
- 5. Pilot Testing: Identifying and Addressing Issues Early
- Interpreting Inter-rater Reliability Coefficients
- Conclusion: The Foundation of Trustworthy Data
- Latest Posts
- Latest Posts
- Related Post
Assessing the Consistency of Observations by Different Observers: A Deep Dive into Inter-rater Reliability
Inter-rater reliability, also known as inter-observer reliability, is a crucial concept in research and various professional fields. It refers to the degree of agreement among different raters or observers who independently assess the same phenomenon. High inter-rater reliability indicates that the observations are consistent and not influenced significantly by individual biases or subjective interpretations. Conversely, low inter-rater reliability raises concerns about the validity and trustworthiness of the observations. This article will delve into the importance of inter-rater reliability, explore different methods for assessing it, and discuss strategies for improving consistency among observers.
The Importance of Inter-rater Reliability
The significance of inter-rater reliability cannot be overstated. It directly impacts the credibility and generalizability of research findings and the accuracy of assessments in various applied settings. Consider the following scenarios:
-
Research Studies: In qualitative research, data often relies heavily on observations and interpretations by researchers. Low inter-rater reliability means that the conclusions drawn from the study might be subjective and not truly reflective of the phenomenon being studied. This significantly undermines the validity and reliability of the research. In quantitative research, consistent scoring across raters is essential for accurate data analysis and meaningful results.
-
Clinical Diagnosis: In healthcare, consistent diagnosis is paramount. If different doctors evaluating the same patient reach vastly different conclusions, it can lead to inconsistencies in treatment plans and potentially harm the patient. Inter-rater reliability ensures that diagnoses are objective and reliable, regardless of the clinician involved.
-
Performance Evaluations: Performance reviews in workplaces often involve subjective assessments of employee behavior and performance. Inconsistencies in evaluations by different supervisors can lead to unfairness and demotivation among employees. High inter-rater reliability ensures that performance evaluations are fair and consistent across the board.
-
Educational Assessments: In education, consistent grading across teachers is critical for ensuring that students are evaluated fairly. If different teachers have widely varying standards for grading, it can lead to inconsistencies in student scores and affect academic progression.
Methods for Assessing Inter-rater Reliability
Several statistical methods exist for assessing inter-rater reliability, each with its own strengths and weaknesses. The choice of method depends on the nature of the data (nominal, ordinal, interval, or ratio) and the number of raters.
1. Percent Agreement: A Simple Start
The simplest method is calculating the percent agreement. This involves comparing the observations of two or more raters and determining the percentage of times they agreed on the same rating or category. While easy to calculate, it has limitations, particularly with a large number of categories or when chance agreement is high. It doesn't account for the possibility of agreement occurring by chance.
2. Cohen's Kappa: Accounting for Chance Agreement
Cohen's kappa (κ) is a more sophisticated measure that corrects for chance agreement. It ranges from -1 to +1, with values closer to +1 indicating stronger agreement. A κ value of 0 indicates agreement no better than chance. Cohen's kappa is suitable for nominal data, meaning categorical data without any inherent order. However, it can be sensitive to the marginal distributions (the proportion of observations in each category by each rater).
3. Fleiss' Kappa: For Multiple Raters
Fleiss' kappa extends Cohen's kappa to situations with more than two raters. This is particularly useful when multiple observers are involved in the assessment process. Like Cohen's kappa, it considers chance agreement.
4. Intraclass Correlation Coefficient (ICC): For Continuous Data
The intraclass correlation coefficient (ICC) is used when the data is continuous (interval or ratio). It assesses the consistency of ratings across raters, considering both the mean and variance of the ratings. Different ICC formulas exist depending on the specific research design. ICC values range from 0 to 1, with higher values indicating greater reliability.
5. Weighted Kappa: Addressing the Severity of Disagreement
Weighted kappa is an extension of Cohen's kappa that allows for weighting the disagreements based on their severity. For example, a disagreement between "strongly agree" and "strongly disagree" might be weighted more heavily than a disagreement between "agree" and "neutral". This is particularly useful when the levels of disagreement have varying degrees of importance.
Strategies for Improving Inter-rater Reliability
Achieving high inter-rater reliability requires careful planning and execution. Here are some key strategies:
1. Clear and Detailed Training: Setting the Standard
Before the observation process begins, all raters must receive comprehensive training on the assessment procedures. This training should include:
- Operational Definitions: Clear and unambiguous definitions of the behaviors or characteristics being observed. Vague descriptions can lead to inconsistencies in interpretation.
- Rating Scales: Consistent use of rating scales or scoring systems. All raters should understand how to use the scales and the criteria for assigning each rating.
- Examples and Practice: Provide raters with ample examples and practice opportunities to ensure consistent application of the assessment criteria. Review and discussion of practice cases can help to identify and address any ambiguities.
2. Standardized Procedures: Maintaining Consistency
Standardized procedures are essential for maintaining consistency across raters. This includes:
- Structured Observation Protocols: The use of structured observation protocols provides a consistent framework for recording observations. This minimizes variability in data collection.
- Data Recording Methods: Consistent use of data recording methods, such as checklists or rating scales, ensures that data is collected in a standardized manner.
- Environmental Control: Wherever possible, control for environmental factors that might influence observations. Consistent environmental conditions can reduce variability in observations.
3. Regular Calibration and Feedback: Continuous Improvement
Regular calibration sessions help to maintain consistency over time. This involves:
- Periodic Meetings: Regular meetings between raters to review observations, discuss discrepancies, and refine the assessment criteria.
- Feedback and Discussion: Providing raters with feedback on their performance and opportunities to discuss any challenges or ambiguities.
- Blind Scoring and Comparisons: Periodically comparing ratings on a subset of cases without revealing the identities of the raters. This helps identify patterns of disagreement and areas requiring further clarification.
4. Selecting Appropriate Measurement Tools: Using the Right Instruments
Choosing the right measurement tools is crucial for achieving high reliability. Consider the following:
- Validity and Reliability of Instruments: Use established instruments with demonstrated validity and reliability. This ensures that the assessment tool itself is accurate and consistent.
- Specificity of Measures: Employ measures that are specific to the behavior or characteristics being assessed. This minimizes ambiguity and enhances consistency.
5. Pilot Testing: Identifying and Addressing Issues Early
Before conducting the main study, conduct pilot testing to identify and address potential issues with the assessment procedures. Pilot testing allows for refinement of the assessment procedures and training materials, thereby increasing the likelihood of achieving high inter-rater reliability.
Interpreting Inter-rater Reliability Coefficients
Interpreting inter-rater reliability coefficients requires careful consideration of the context and the specific method used. There is no universally accepted cutoff for acceptable reliability. However, general guidelines suggest that:
- Excellent reliability: Values above 0.80 (for ICC) or above 0.75 (for Cohen’s kappa) generally indicate excellent agreement.
- Good reliability: Values between 0.60 and 0.79 (for ICC) or between 0.60 and 0.74 (for Cohen’s kappa) suggest good agreement.
- Moderate reliability: Values between 0.40 and 0.59 (for ICC) or between 0.40 and 0.59 (for Cohen’s kappa) indicate moderate agreement.
- Poor reliability: Values below 0.40 (for both ICC and Cohen’s kappa) usually indicate poor agreement and raise concerns about the validity of the observations.
It is crucial to consider the specific context of the study when interpreting these values. A reliability coefficient that is considered acceptable in one setting might be unacceptable in another.
Conclusion: The Foundation of Trustworthy Data
Inter-rater reliability is a cornerstone of trustworthy data in research and applied settings. It ensures that observations are consistent, objective, and not unduly influenced by individual biases. By implementing the strategies outlined in this article, researchers and professionals can significantly improve the consistency of observations by different observers, ultimately leading to more credible and reliable findings. Remember that achieving high inter-rater reliability is an ongoing process that requires careful attention to detail, meticulous planning, and continuous monitoring and improvement. The effort invested in ensuring high inter-rater reliability is always worthwhile, as it ultimately safeguards the validity and integrity of the research or assessment process.
Latest Posts
Latest Posts
-
The Distinction Between Impulse And Force Involves The
Apr 04, 2025
-
Which Of The Following Is True Of The
Apr 04, 2025
-
Your Restaurants Fish Delivery Just Arrived
Apr 04, 2025
-
Turbine Blades Mounted To A Rotating Disc
Apr 04, 2025
-
Identify The Type Of Surface Represented By The Given Equation
Apr 04, 2025
Related Post
Thank you for visiting our website which covers about Assesses The Consistency Of Observations By Different Observers . We hope the information provided has been useful to you. Feel free to contact us if you have any questions or need further assistance. See you next time and don't miss to bookmark.