A Significant Weakness Of The Ordinal Scale Is
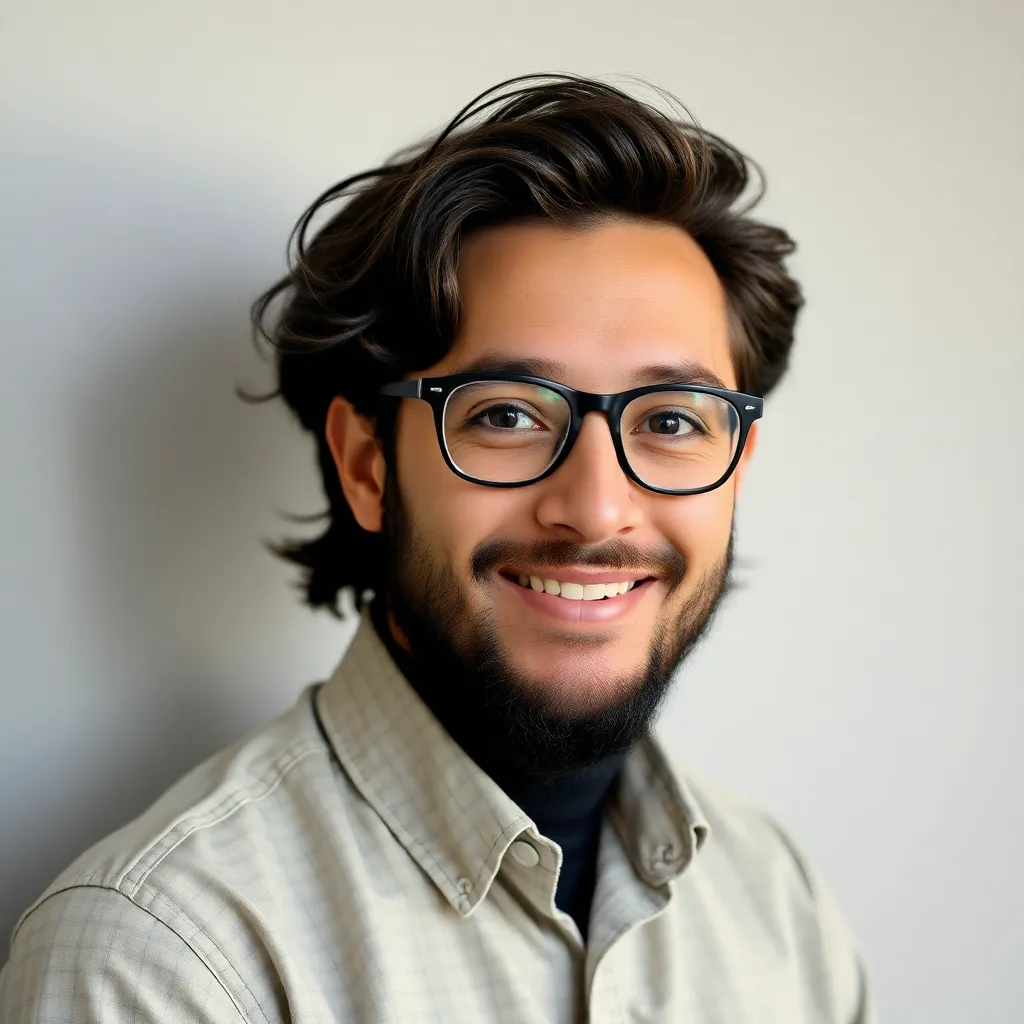
Holbox
Mar 28, 2025 · 6 min read
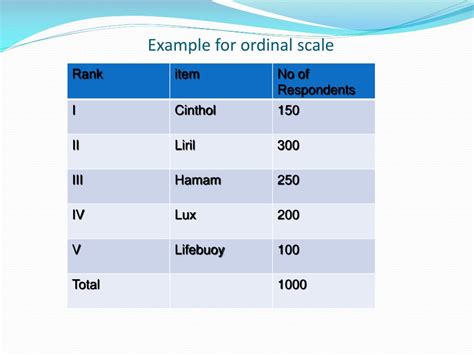
Table of Contents
- A Significant Weakness Of The Ordinal Scale Is
- Table of Contents
- A Significant Weakness of the Ordinal Scale: The Problem of Unequal Intervals and Its Implications
- Understanding the Ordinal Scale and its Limitations
- Examples Illustrating Unequal Intervals
- Implications of Unequal Intervals in Data Analysis
- Restricted Statistical Operations:
- Potential for Misinterpretation:
- Mitigating the Weakness of Ordinal Scales: Strategies and Approaches
- 1. Utilize Appropriate Statistical Techniques:
- 2. Consider Transforming Ordinal Data:
- 3. Enhance Data Collection Methods:
- 4. Careful Interpretation and Reporting:
- Conclusion: Harnessing the Utility While Acknowledging Limitations
- Latest Posts
- Latest Posts
- Related Post
A Significant Weakness of the Ordinal Scale: The Problem of Unequal Intervals and Its Implications
The ordinal scale, a common type of measurement in research, ranks data according to order or rank. While it provides valuable information about the relative position of data points, a significant weakness lies in its inability to quantify the difference between ranks. This limitation, stemming from the unequal intervals between ordinal data points, has significant implications for analysis and interpretation. This article will delve deep into this weakness, exploring its consequences and suggesting strategies to mitigate its impact.
Understanding the Ordinal Scale and its Limitations
The ordinal scale organizes data into categories that possess a meaningful order. Think of rankings like "first place," "second place," "third place" in a race, or customer satisfaction levels ranging from "very dissatisfied" to "very satisfied". Unlike nominal scales which simply categorize data without any inherent order (e.g., colors, gender), ordinal scales incorporate a clear ranking system. However, the crucial difference, and the source of its weakness, is the absence of information on the magnitude of the difference between ranks.
The core problem: The gap between "first" and "second" might be vastly different from the gap between "second" and "third." In a race, the difference in time between the first and second runner could be a mere tenth of a second, while the difference between the second and third could be several seconds. The ordinal scale fails to capture this crucial quantitative information. All it tells us is the order; it doesn't quantify the distance between the ranks.
Examples Illustrating Unequal Intervals
Consider these examples to further understand the unequal intervals problem:
- Customer Satisfaction Surveys: A customer rating their experience as "satisfied" doesn't inherently tell us how much more satisfied they are compared to someone rating their experience as "neutral." The difference in underlying sentiment might be substantial, or it might be negligible.
- Educational Grades: An A student is superior to a B student, but the difference in academic performance between an A and a B might be smaller than the difference between a B and a C, depending on the grading system and individual performance.
- Socioeconomic Status: Classifying individuals into "low," "middle," and "high" socioeconomic status provides an ordered ranking, but the economic disparities between these groups can vary significantly depending on the context and definitions used.
Implications of Unequal Intervals in Data Analysis
The unequal intervals inherent in ordinal data restrict the types of statistical analyses that can be meaningfully applied. Many statistical techniques rely on the assumption of equal intervals or at least an approximately known interval scale. Using inappropriate statistical methods on ordinal data can lead to misleading or inaccurate conclusions.
Restricted Statistical Operations:
- Arithmetic Mean: Calculating the mean (average) of ordinal data is generally inappropriate. The mean assumes equal intervals, which doesn't hold true for ordinal data. Averaging ranks can produce a nonsensical result, failing to reflect the true underlying distribution.
- Standard Deviation: Similarly, the standard deviation, a measure of data dispersion, is not suitable for ordinal data. It depends on the assumption of equal intervals, and its application to ordinal data will yield misleading results about the spread of the data.
- Ratio Comparisons: It's meaningless to say that someone ranked "first" is "twice as good" as someone ranked "second." Such ratio comparisons are only valid for interval or ratio scales where the intervals between data points are known and equal.
Potential for Misinterpretation:
The inability to quantify differences between ranks can lead to inaccurate conclusions drawn from the data. For example, if analyzing customer satisfaction scores, concluding that a slight increase in the number of "satisfied" responses represents a major improvement in overall satisfaction might be unwarranted without further investigation. The increase might reflect very small, inconsequential changes in customer experience.
Mitigating the Weakness of Ordinal Scales: Strategies and Approaches
While the inherent limitations of ordinal data cannot be entirely eliminated, researchers can employ several strategies to mitigate their impact and draw more meaningful conclusions from their analysis:
1. Utilize Appropriate Statistical Techniques:
Ordinal data necessitates the use of non-parametric statistical tests. These tests are designed for data that doesn't meet the assumptions of parametric tests (like the t-test or ANOVA) which require equal intervals. Examples include:
- Spearman's Rank Correlation: Measures the monotonic relationship between two ordinal variables.
- Mann-Whitney U Test: Compares the distributions of two independent ordinal variables.
- Kruskal-Wallis Test: Compares the distributions of three or more independent ordinal variables.
- Wilcoxon Signed-Rank Test: Compares the distributions of two related ordinal variables.
These non-parametric methods avoid the problematic assumption of equal intervals.
2. Consider Transforming Ordinal Data:
While not always appropriate, in some cases, it might be possible to transform ordinal data to approximate an interval scale. Techniques like:
- Rank-based transformations: Assigning scores to ranks based on some scaling assumption (e.g., assigning 5 to "very satisfied", 4 to "satisfied", etc.). This is subjective and must be justified based on the context.
- Cumulative scaling: Assigning scores based on the cumulative proportion of responses. This approach can be more robust than arbitrary scoring.
However, any such transformation should be approached cautiously and its limitations clearly acknowledged. The transformation itself introduces assumptions that may not always be accurate.
3. Enhance Data Collection Methods:
The best approach is often to improve the quality of data collection in the first place. Consider using:
- Interval or ratio scales: Wherever possible, collecting data using interval or ratio scales (e.g., using visual analog scales or Likert scales with many points instead of just a few categories) provides much richer data with far fewer limitations.
- More granular scales: Instead of a few broad categories (e.g., "satisfied," "neutral," "dissatisfied"), employing a more granular scale (e.g., a 7-point Likert scale) allows for finer distinctions and captures more nuanced information.
- Open-ended questions: Combining ordinal scale questions with open-ended questions allows for qualitative data that can provide context and better interpret the quantitative data. This can illuminate the underlying reasons for responses and help explain the variations between ranks.
4. Careful Interpretation and Reporting:
Irrespective of the analytical method, cautious interpretation is crucial. Avoid making unwarranted generalizations or drawing conclusions that are not supported by the data. Clearly state the limitations of ordinal data and the methods used in the analysis. Transparency regarding the limitations helps avoid misinterpretations.
Conclusion: Harnessing the Utility While Acknowledging Limitations
Ordinal scales remain a valuable tool in many research settings, particularly when dealing with subjective measures or when numerical precision is not feasible. The key is to understand the inherent limitations imposed by unequal intervals. By choosing appropriate statistical techniques, carefully considering data collection methods, and interpreting results cautiously, researchers can effectively harness the utility of ordinal data while mitigating the risks associated with its limitations. Remembering the weakness of unequal intervals, and taking steps to address this issue, ensures more robust and reliable conclusions from research involving ordinal data. By acknowledging and proactively managing this weakness, we can ensure the appropriate use and interpretation of ordinal scales, leading to clearer understanding and more impactful findings.
Latest Posts
Latest Posts
-
Which Of The Following Is Not A Possible R Value
Apr 01, 2025
-
Record The Adjusting Entry For Uncollectible Accounts
Apr 01, 2025
-
Como Se Llama El Padre De Sara
Apr 01, 2025
-
Managerial Reporting Systems Are Rigid Flexible Standardized
Apr 01, 2025
-
Physical Examination And Health Assessment By Jarvis
Apr 01, 2025
Related Post
Thank you for visiting our website which covers about A Significant Weakness Of The Ordinal Scale Is . We hope the information provided has been useful to you. Feel free to contact us if you have any questions or need further assistance. See you next time and don't miss to bookmark.