Which Of The Following Is Not A Possible R Value
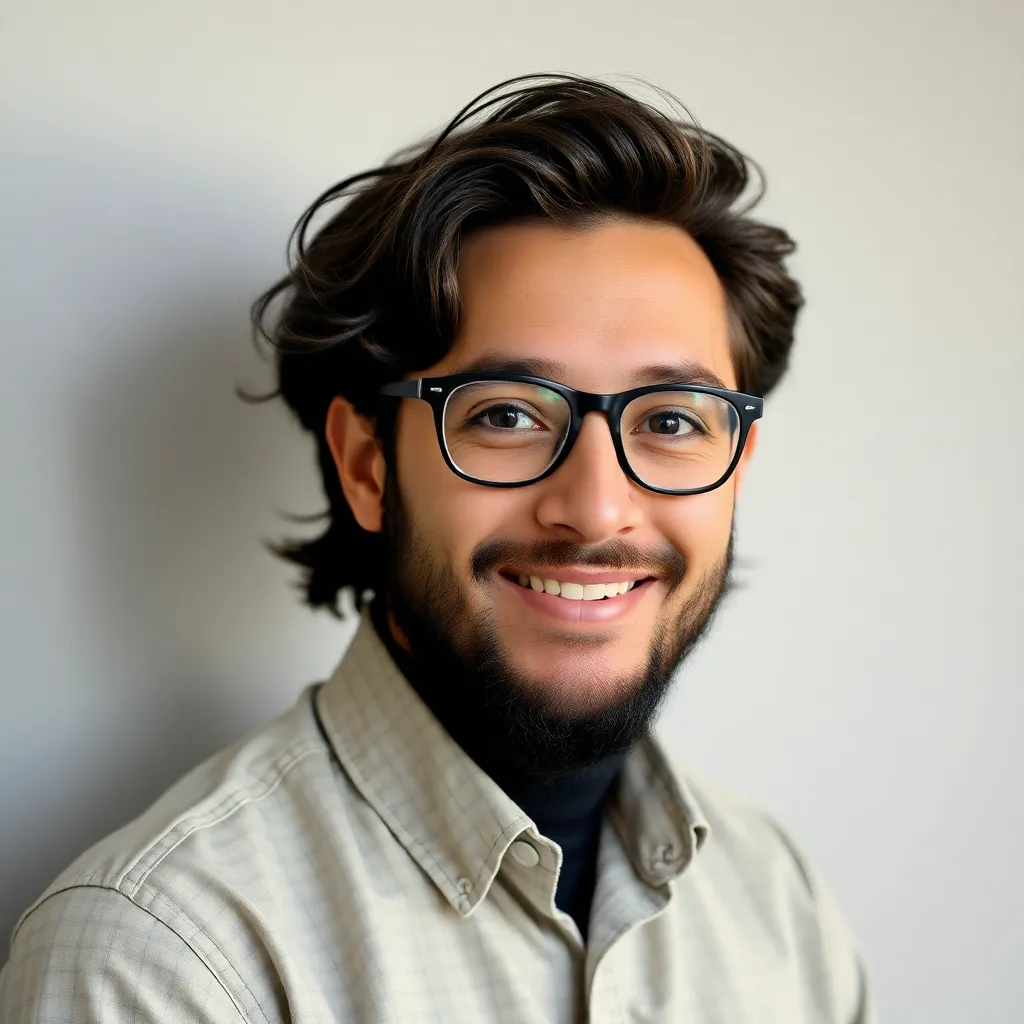
Holbox
Apr 01, 2025 · 5 min read

Table of Contents
- Which Of The Following Is Not A Possible R Value
- Table of Contents
- Which of the following is not a possible r value? Understanding Correlation Coefficients
- What is a Correlation Coefficient (r)?
- The Impossible r Values: Why Values Outside -1 to +1 are Impossible
- Common Misconceptions about the Correlation Coefficient
- Examples of Impossible r Values and Their Implications
- Interpreting Different Ranges of r Values
- Beyond Pearson's r: Other Correlation Measures
- Conclusion: Mastering Correlation Coefficients for Accurate Data Analysis
- Latest Posts
- Latest Posts
- Related Post
Which of the following is not a possible r value? Understanding Correlation Coefficients
The correlation coefficient, denoted by 'r', is a crucial statistical measure that quantifies the linear relationship between two variables. It tells us the strength and direction of this relationship. Understanding the possible values of 'r' is fundamental to interpreting correlation analyses and drawing accurate conclusions from data. This article delves deep into the concept of the correlation coefficient, explaining what it represents, its range, and why certain values are impossible. We'll also explore common misconceptions and provide practical examples to solidify your understanding.
What is a Correlation Coefficient (r)?
The correlation coefficient (r), also known as Pearson's r, measures the strength and direction of a linear association between two continuous variables. It ranges from -1 to +1, inclusive.
-
r = +1: Indicates a perfect positive linear correlation. As one variable increases, the other increases proportionally. The data points would lie perfectly on a straight line with a positive slope.
-
r = -1: Indicates a perfect negative linear correlation. As one variable increases, the other decreases proportionally. The data points would lie perfectly on a straight line with a negative slope.
-
r = 0: Indicates no linear correlation. There's no linear relationship between the variables, although other types of relationships (non-linear) might still exist.
-
Values between -1 and +1: Represent varying degrees of linear correlation. Values closer to -1 or +1 indicate stronger correlations, while values closer to 0 indicate weaker correlations.
Important Note: Correlation does not imply causation. Just because two variables are correlated doesn't mean one causes the other. There might be a third, unmeasured variable influencing both.
The Impossible r Values: Why Values Outside -1 to +1 are Impossible
The reason why values outside the range of -1 to +1 are impossible stems from the mathematical formula used to calculate the correlation coefficient. The formula involves the covariance of the two variables and their standard deviations. The mathematical properties of these quantities restrict the possible values of 'r' to the interval [-1, 1].
Let's break this down further. The formula for Pearson's r is:
r = Σ[(xi - x̄)(yi - ȳ)] / √[Σ(xi - x̄)² * Σ(yi - ȳ)²]
where:
- xi and yi are individual data points for variables x and y respectively.
- x̄ and ȳ are the means of variables x and y respectively.
- Σ denotes the sum of the values.
The numerator represents the covariance, measuring the direction and strength of the linear relationship between x and y. The denominator involves the standard deviations of x and y, scaling the covariance to a standardized value between -1 and 1. The Cauchy-Schwarz inequality in mathematics proves that the absolute value of the covariance is always less than or equal to the product of the standard deviations. This inequality directly leads to the constraint that |r| ≤ 1.
Common Misconceptions about the Correlation Coefficient
Several misconceptions surround the interpretation of correlation coefficients:
-
Ignoring Non-linear Relationships: A correlation coefficient of 0 only indicates the absence of a linear relationship. A strong non-linear relationship might exist, but 'r' won't capture it. Consider a parabolic relationship; 'r' could be close to zero despite a strong relationship.
-
Causation vs. Correlation: This is the most critical misconception. Correlation does not equal causation. A high correlation between two variables doesn't necessarily mean one causes the other. A confounding variable might be influencing both.
-
Extrapolating Beyond the Data Range: The correlation coefficient describes the relationship within the observed data range. Extrapolating beyond this range can be misleading and inaccurate. The relationship might change outside the observed range.
-
Misinterpreting Weak Correlations: A weak correlation (r close to 0) doesn't necessarily imply a lack of relationship. It could indicate a weak linear relationship or the presence of a strong non-linear relationship.
Examples of Impossible r Values and Their Implications
Let's consider some examples to illustrate why certain values are impossible:
-
r = 1.5: This is impossible. The correlation coefficient can never exceed 1, even in cases with a perfect positive relationship.
-
r = -2: This is also impossible. The correlation coefficient can't be less than -1, even if the variables have a perfect negative relationship.
These impossible values highlight the importance of understanding the limitations of the correlation coefficient. Always examine scatter plots to visually assess the relationship between variables and avoid overinterpreting the numerical value of 'r'.
Interpreting Different Ranges of r Values
While the exact interpretation depends on the context and field of study, general guidelines exist for interpreting the strength of a correlation based on the magnitude of 'r':
-
|r| ≥ 0.8: Strong correlation. Indicates a substantial linear relationship.
-
0.6 ≤ |r| < 0.8: Moderate correlation. Indicates a noticeable linear relationship.
-
0.4 ≤ |r| < 0.6: Weak correlation. Indicates a weak linear relationship.
-
|r| < 0.4: Very weak or no correlation. Indicates a negligible linear relationship.
Remember that these are guidelines and the practical significance of a correlation coefficient depends heavily on the context of the study.
Beyond Pearson's r: Other Correlation Measures
While Pearson's r is widely used, it's not suitable for all data types. Other correlation measures exist, such as:
-
Spearman's rank correlation coefficient: Used for ordinal data or when the relationship isn't linear. It measures the monotonic relationship between variables.
-
Kendall's tau: Another non-parametric correlation measure suitable for ordinal data and non-linear relationships.
The choice of correlation measure depends on the nature of the data and the type of relationship being investigated.
Conclusion: Mastering Correlation Coefficients for Accurate Data Analysis
Understanding the possible values of the correlation coefficient, 'r', is crucial for accurate data analysis and interpretation. Remember that 'r' can only range from -1 to +1, inclusive. Values outside this range are impossible due to the mathematical constraints of the formula. It's essential to avoid common misconceptions, like confusing correlation with causation or ignoring non-linear relationships. Always visually inspect scatter plots and consider the context of your study when interpreting the correlation coefficient. Mastering these concepts will greatly enhance your ability to analyze data effectively and draw meaningful conclusions. By understanding the limitations and nuances of correlation coefficients, you can make better-informed decisions based on data-driven insights. Remember to always consider the limitations and context before making any conclusions based solely on the correlation coefficient.
Latest Posts
Latest Posts
-
Job Enrichment Differs From Job Rotation In That Job Enrichment
Apr 05, 2025
-
A Food Company Hydrogenated A Barrel Of Fat The Treatment
Apr 05, 2025
-
According To The Efficient Market Hypothesis
Apr 05, 2025
-
Comparisons Of Financial Data Made Within A Company Are Called
Apr 05, 2025
-
The Components Of Global Market Assessment Include
Apr 05, 2025
Related Post
Thank you for visiting our website which covers about Which Of The Following Is Not A Possible R Value . We hope the information provided has been useful to you. Feel free to contact us if you have any questions or need further assistance. See you next time and don't miss to bookmark.