With A Probabilistic Model Increasing The Service Level
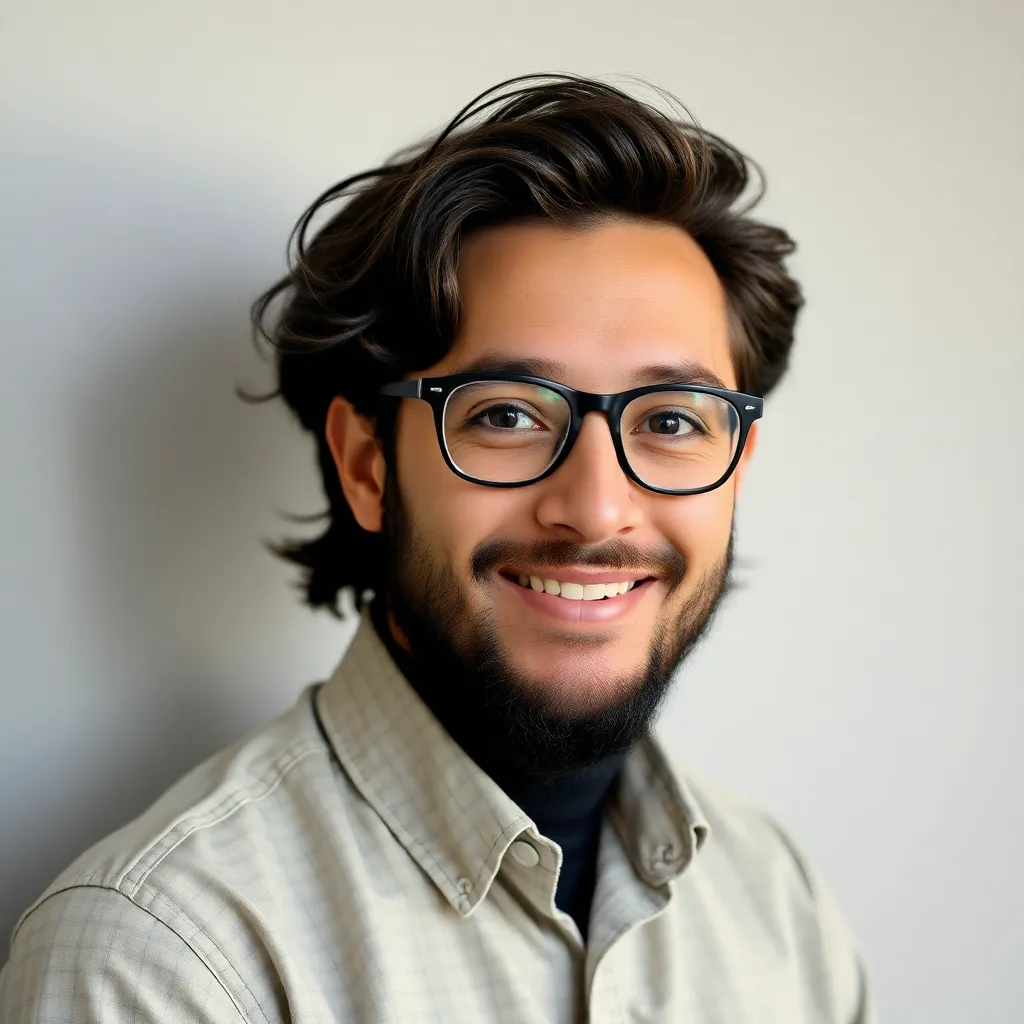
Holbox
May 11, 2025 · 6 min read
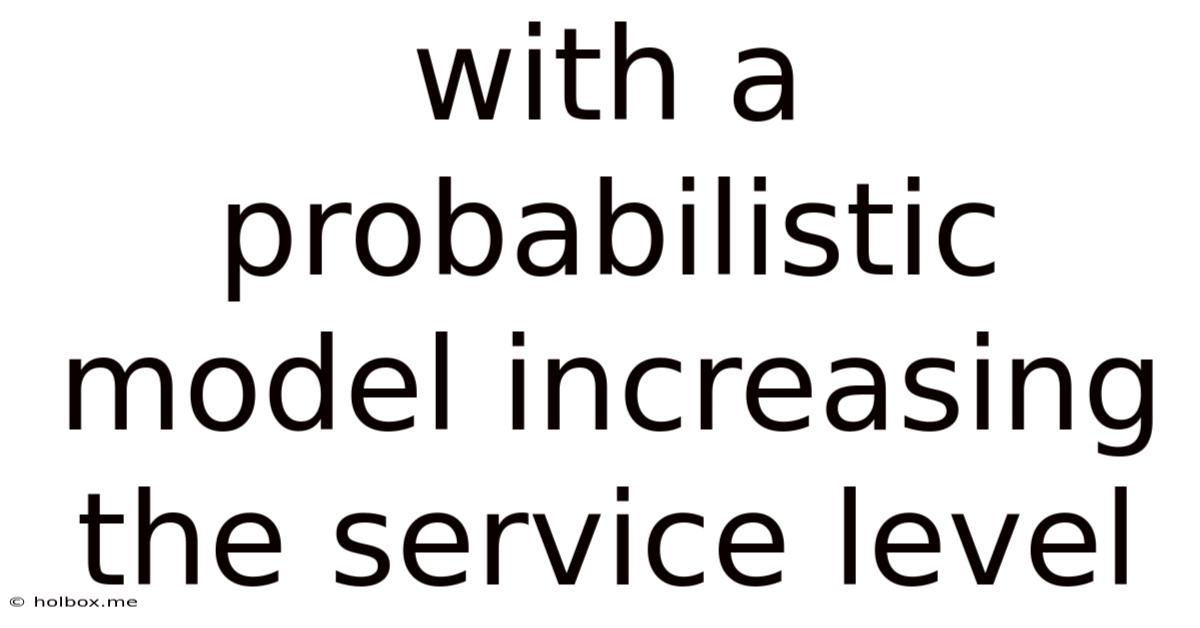
Table of Contents
- With A Probabilistic Model Increasing The Service Level
- Table of Contents
- Increasing Service Level with a Probabilistic Model
- Understanding Service Level and Its Challenges
- The Power of Probabilistic Modeling
- Types of Probabilistic Models for Service Level Improvement
- 1. Queuing Models
- 2. Markov Chains
- 3. Bayesian Networks
- 4. Monte Carlo Simulation
- Implementing Probabilistic Models: A Step-by-Step Guide
- Case Studies: Real-World Applications
- Conclusion: Embracing Uncertainty for Improved Service
- Latest Posts
- Latest Posts
- Related Post
Increasing Service Level with a Probabilistic Model
Improving service levels is a crucial goal for any business, particularly those operating in competitive markets or dealing with sensitive customer expectations. A probabilistic model offers a powerful approach to achieve this, allowing for more accurate forecasting, proactive resource allocation, and informed decision-making. This approach moves beyond simple averages and embraces the inherent uncertainty in many service-related processes. This article will delve into the application of probabilistic models in enhancing service levels, examining various techniques, advantages, and considerations.
Understanding Service Level and Its Challenges
Before diving into probabilistic models, it's vital to define service level. In essence, it represents the agreed-upon performance target between a service provider and its customer (internal or external). This could be measured in various ways, including:
- Order fulfillment rate: The percentage of orders successfully processed and delivered.
- Resolution time: The time taken to resolve customer issues or inquiries.
- Uptime: The percentage of time a system or service is operational.
- Customer satisfaction: Measured through surveys or feedback mechanisms.
Achieving consistently high service levels presents several challenges:
- Variability: Customer demand, system performance, and unforeseen events can introduce significant variability, making accurate forecasting difficult.
- Resource constraints: Limited resources (personnel, budget, equipment) often restrict the ability to meet peak demand or handle unexpected surges.
- Lack of visibility: Without real-time monitoring and data analysis, it's challenging to identify bottlenecks and areas for improvement.
- Data limitations: Insufficient or unreliable data can hinder the development of effective predictive models.
The Power of Probabilistic Modeling
Probabilistic models embrace the inherent uncertainty in service-related processes. They go beyond deterministic approaches that assume perfect predictability, instead providing a framework for dealing with variability and risk. Key advantages include:
- Improved forecasting accuracy: Probabilistic forecasts provide a range of possible outcomes, along with their associated probabilities, giving a much more realistic view of future demand. This is crucial for proactive resource allocation.
- Risk management: By quantifying the probability of various scenarios (e.g., exceeding capacity, falling short of targets), businesses can proactively mitigate risks and develop contingency plans.
- Optimized resource allocation: Knowing the probability of various demand levels allows for more effective resource allocation, avoiding both over-provisioning (wasteful expenditure) and under-provisioning (compromising service levels).
- Data-driven decision-making: Probabilistic models are data-driven, allowing for informed decisions based on evidence rather than intuition.
- Continuous improvement: The iterative nature of model development enables continuous monitoring, refinement, and optimization, leading to ongoing service level improvements.
Types of Probabilistic Models for Service Level Improvement
Several probabilistic models are applicable to improving service levels. The choice of model depends on the specific context, data availability, and complexity of the system:
1. Queuing Models
Queuing models are particularly relevant when dealing with waiting times and service capacity. They analyze the flow of customers or requests through a system, considering arrival rates, service times, and the number of servers. Key metrics include average waiting time, queue length, and server utilization. Examples include M/M/1 (Markovian arrival process, Markovian service process, single server), M/M/c (multiple servers), and more complex models incorporating variations in arrival or service times.
Application: Analyzing call center performance, optimizing hospital emergency room staffing, or managing online order fulfillment.
2. Markov Chains
Markov chains are useful for modeling systems that transition between different states over time. The probability of moving from one state to another depends only on the current state, not the past history. This makes them suitable for modeling systems with discrete states and time steps.
Application: Modeling customer churn (transitioning between loyal and churned states), predicting equipment failure (transitioning between operational and failed states), or tracking the progress of a service request through different stages.
3. Bayesian Networks
Bayesian networks are powerful tools for representing and reasoning under uncertainty. They model the relationships between multiple variables, using probabilities to quantify the influence of one variable on another. This allows for incorporating prior knowledge and updating beliefs as new data become available.
Application: Diagnosing system failures, predicting customer behavior, or optimizing preventive maintenance schedules based on equipment age and usage patterns.
4. Monte Carlo Simulation
Monte Carlo simulation is a versatile technique that involves repeatedly sampling from probability distributions to simulate the behavior of a system. This allows for generating a large number of possible outcomes, providing a statistical distribution of results. This is especially useful when dealing with complex systems with many interacting variables.
Application: Forecasting future demand, evaluating the impact of different resource allocation strategies, or assessing the risk of service disruptions.
Implementing Probabilistic Models: A Step-by-Step Guide
Implementing a probabilistic model to enhance service levels involves several key steps:
- Data Collection and Preparation: Gather relevant data, including historical service performance, customer demand patterns, and resource utilization. Clean and prepare the data for analysis. Ensure data quality is high to avoid bias in model outputs.
- Model Selection: Choose the appropriate probabilistic model based on the nature of the system, the available data, and the specific objectives. Consider the trade-off between model complexity and accuracy.
- Model Calibration and Validation: Estimate the model parameters using the available data. Validate the model's accuracy and predictive power using appropriate statistical methods. Ensure the model generalizes well to unseen data.
- Scenario Analysis: Use the calibrated model to simulate different scenarios, such as changes in demand, resource availability, or operational processes. Assess the impact of these scenarios on service levels.
- Decision Support: Use the model's outputs to inform decision-making, such as resource allocation, capacity planning, or service improvement initiatives.
- Monitoring and Refinement: Continuously monitor the model's performance and update it as new data become available or as the system evolves.
Case Studies: Real-World Applications
Numerous real-world examples demonstrate the effectiveness of probabilistic models in improving service levels:
- Call Centers: Queuing models can predict call volumes and optimize staffing levels to minimize waiting times and ensure efficient service.
- Supply Chain Management: Probabilistic forecasting methods can anticipate fluctuations in demand and optimize inventory levels, preventing stockouts and minimizing storage costs.
- Healthcare: Probabilistic models can assist in allocating resources (doctors, nurses, equipment) to effectively handle patient flow and optimize hospital operations.
- IT Operations: Predictive maintenance models can identify the probability of equipment failures and schedule preventive maintenance to minimize downtime.
Conclusion: Embracing Uncertainty for Improved Service
Probabilistic models offer a powerful and versatile approach to improving service levels. By embracing the inherent uncertainty in service-related processes, these models enable more accurate forecasting, proactive resource allocation, and data-driven decision-making. Through careful data analysis, model selection, and ongoing monitoring, businesses can leverage probabilistic modeling to enhance their service levels, improve customer satisfaction, and gain a competitive advantage in today's dynamic marketplace. Remember that the implementation requires a collaborative effort between data scientists, operational managers, and customer-facing teams to ensure the model's insights are effectively translated into tangible improvements. The continuous improvement cycle inherent in this approach will ensure long-term benefits and adaptability to changing market conditions.
Latest Posts
Latest Posts
-
300 Km Is How Many Miles
May 19, 2025
-
How Many Inches Is 19 Cm
May 19, 2025
-
50 Cm In Inches And Feet
May 19, 2025
-
What Is 18 Pounds In Kg
May 19, 2025
-
What Is 52 Cm In Inches
May 19, 2025
Related Post
Thank you for visiting our website which covers about With A Probabilistic Model Increasing The Service Level . We hope the information provided has been useful to you. Feel free to contact us if you have any questions or need further assistance. See you next time and don't miss to bookmark.