Which Statement Is Supported By The Data Table
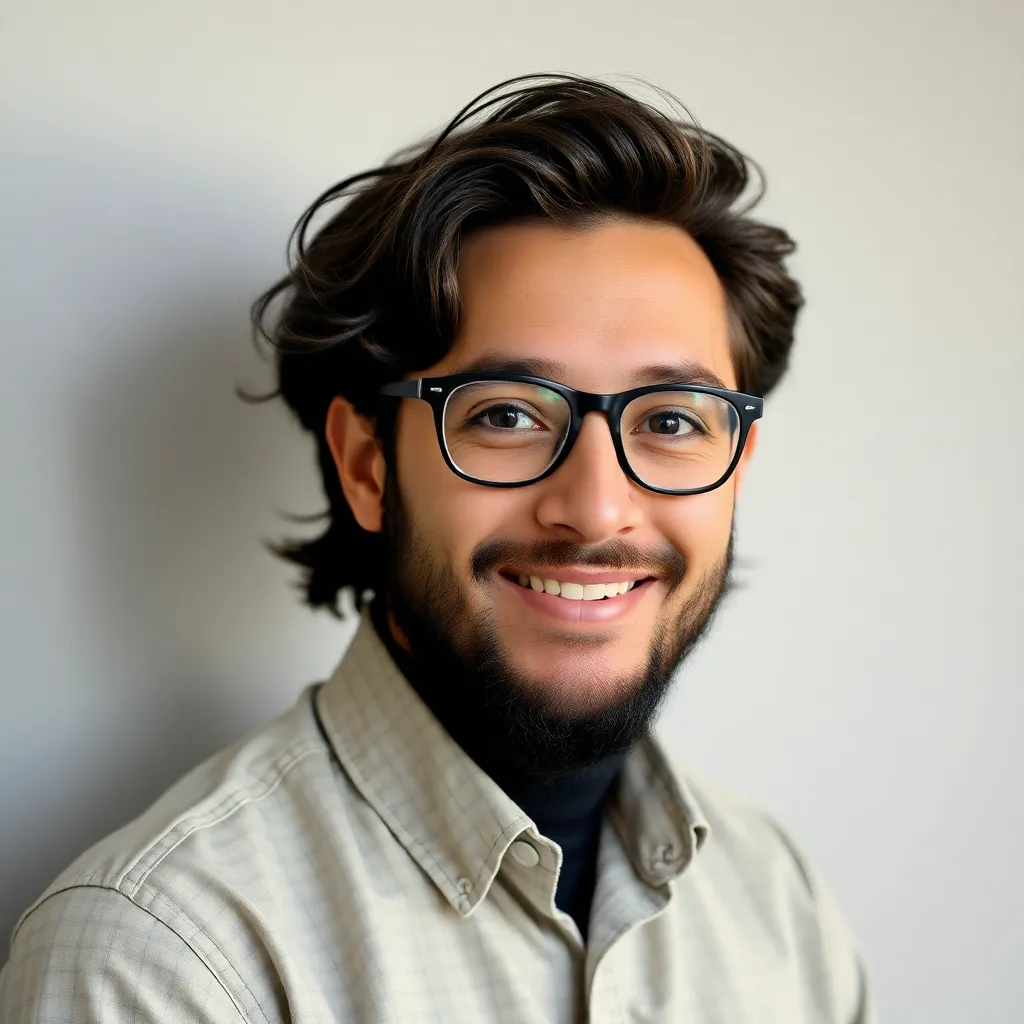
Holbox
May 07, 2025 · 6 min read
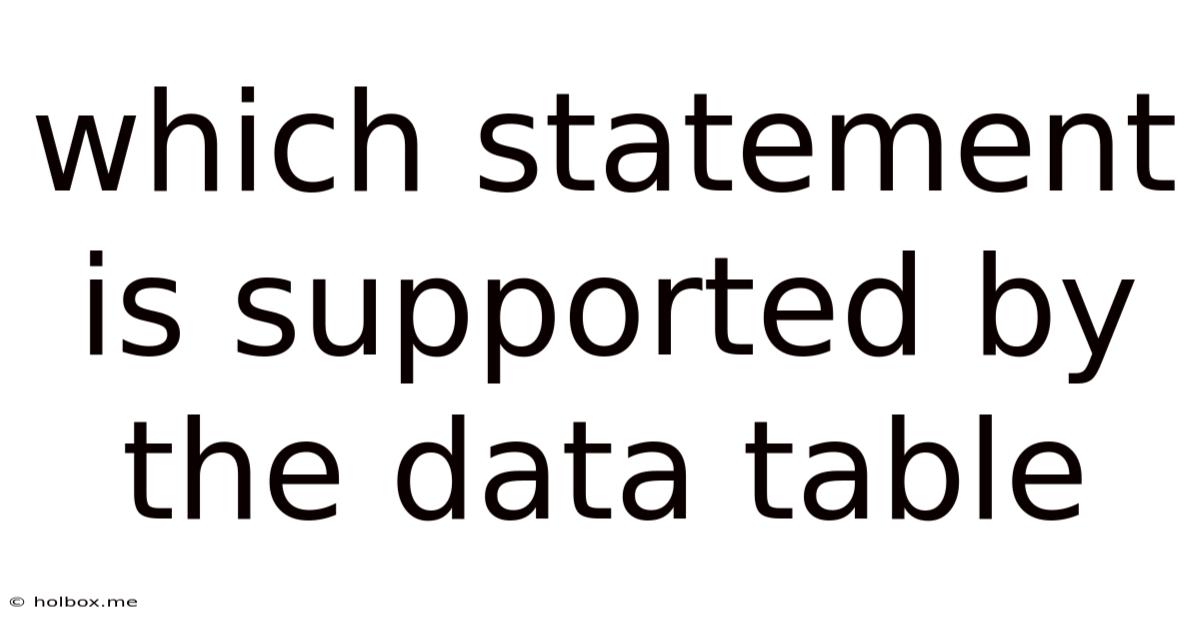
Table of Contents
- Which Statement Is Supported By The Data Table
- Table of Contents
- Which Statement is Supported by the Data Table: A Comprehensive Guide to Data Analysis and Interpretation
- 1. Identify the Variables and Their Types
- 2. Examine Data Distribution and Trends
- 3. Compare and Contrast Different Groups
- 4. Formulate Hypotheses and Test Them
- 5. Draw Conclusions Based on Evidence
- Latest Posts
- Related Post
Which Statement is Supported by the Data Table: A Comprehensive Guide to Data Analysis and Interpretation
Analyzing data tables is a crucial skill across numerous fields, from scientific research and business analytics to journalism and everyday decision-making. Understanding how to interpret data and identify which statements are supported by the evidence presented is paramount. This comprehensive guide will explore various techniques and considerations for effectively analyzing data tables and drawing accurate conclusions.
Understanding Data Tables: Structure and Components
Before diving into analysis, it's essential to understand the basic structure of a data table. A typical data table consists of:
- Rows: These represent individual observations or data points.
- Columns: These represent different variables or characteristics being measured.
- Headers: These clearly label each column, indicating the variable it represents.
- Data Cells: These contain the actual values for each observation and variable.
A well-constructed data table is crucial for clear and accurate analysis. Inconsistent formatting, missing data, or unclear labels can significantly hinder the interpretation process.
Key Steps in Analyzing Data Tables
Analyzing a data table involves a systematic approach to ensure accurate conclusions. The following steps are fundamental:
1. Identify the Variables and Their Types
Begin by carefully examining the table headers to identify the variables involved. Determine whether the variables are categorical (e.g., color, gender, location) or numerical (e.g., age, height, income). Understanding the variable types will influence the analytical techniques you employ.
2. Examine Data Distribution and Trends
Once you've identified the variables, look for patterns and trends within the data. This might involve:
- Calculating summary statistics: For numerical variables, calculate measures like mean, median, mode, standard deviation, and range to understand the central tendency and spread of the data.
- Visualizing the data: Create charts and graphs (histograms, bar charts, scatter plots) to visualize the data distribution and identify any trends or outliers. Visualizations often reveal patterns that are not immediately apparent in the raw data.
- Identifying outliers: Outliers are data points that are significantly different from the rest of the data. These might represent errors in data collection or genuine anomalies. Consider whether to exclude them from your analysis, or investigate the reason for their unusual values.
3. Compare and Contrast Different Groups
If the table contains data for different groups (e.g., different age groups, different treatment groups), compare and contrast these groups. This might involve calculating summary statistics for each group and performing statistical tests (e.g., t-tests, ANOVA) to determine if there are statistically significant differences between the groups.
4. Formulate Hypotheses and Test Them
Based on your observations, formulate specific hypotheses about relationships between variables. For example, you might hypothesize that there is a correlation between age and income. Then, use appropriate statistical tests to determine if your hypotheses are supported by the data. Remember that correlation does not imply causation.
5. Draw Conclusions Based on Evidence
Finally, draw conclusions based on your analysis. Crucially, ensure that your conclusions are directly supported by the data presented in the table. Avoid making generalizations or interpretations that are not justified by the evidence.
Common Errors in Data Table Interpretation
Several common errors can lead to inaccurate conclusions when analyzing data tables:
- Ignoring context: Always consider the context in which the data was collected. The meaning of the data can change dramatically depending on the context.
- Misinterpreting correlations: Correlation does not imply causation. Just because two variables are correlated does not mean that one causes the other. There might be a third, confounding variable at play.
- Overgeneralizing: Avoid making broad generalizations based on limited data. The data in the table may only represent a specific sample, and your conclusions might not apply to the broader population.
- Ignoring sampling bias: Consider whether the sample represented in the table is representative of the population you are interested in. Sampling bias can lead to inaccurate conclusions.
- Failing to account for uncertainty: Remember that data analysis always involves some degree of uncertainty. Consider the margins of error and confidence intervals when interpreting the results.
- Confirmation bias: Avoid seeking out only evidence that supports your preconceived notions. Consider all the evidence, including evidence that contradicts your hypotheses.
Examples of Statements and Data Table Analysis
Let's illustrate these principles with some examples. Imagine a data table showing the number of hours studied and the exam scores of 10 students.
Data Table Example:
Student | Hours Studied | Exam Score |
---|---|---|
1 | 5 | 75 |
2 | 10 | 90 |
3 | 2 | 50 |
4 | 8 | 85 |
5 | 12 | 95 |
6 | 3 | 60 |
7 | 7 | 80 |
8 | 15 | 98 |
9 | 4 | 65 |
10 | 9 | 88 |
Statements to Evaluate:
- Statement A: Students who study more hours tend to get higher exam scores.
- Statement B: Studying 10 hours guarantees a score above 90.
- Statement C: There is a perfect correlation between hours studied and exam score.
- Statement D: All students who studied less than 5 hours scored below 70.
Analysis:
-
Statement A: This statement is generally supported by the data. A visual inspection and calculation of the correlation coefficient would likely reveal a positive correlation between hours studied and exam score. However, it's crucial to avoid claiming causality. Other factors could contribute to exam performance.
-
Statement B: This statement is not supported. While a student who studied 10 hours did score 90, this doesn't guarantee that all students studying 10 hours will achieve the same result.
-
Statement C: This statement is not supported. The data shows a positive correlation, but it's far from perfect. There's variability in exam scores even for similar study times.
-
Statement D: This statement is not fully supported. While most students studying less than 5 hours scored below 70, there is at least one exception (Student 1).
Conclusion:
Carefully examining the data table, visualizing the data, and understanding statistical concepts are essential for drawing accurate and meaningful conclusions. Always ensure that your statements are directly supported by the evidence and avoid overgeneralizations or interpretations that aren't justified by the data. Critical thinking and a nuanced understanding of data analysis are key to avoiding pitfalls and extracting valuable insights from data tables. This systematic approach will lead to more accurate interpretations and reliable conclusions across various applications. Remember to always consider the limitations of your data and avoid making claims beyond what the data supports. By following these guidelines, you can significantly improve your data analysis skills and confidently draw accurate conclusions based on the evidence presented in data tables.
Latest Posts
Related Post
Thank you for visiting our website which covers about Which Statement Is Supported By The Data Table . We hope the information provided has been useful to you. Feel free to contact us if you have any questions or need further assistance. See you next time and don't miss to bookmark.