Which One Of These Best Illustrates Time Series Analysis
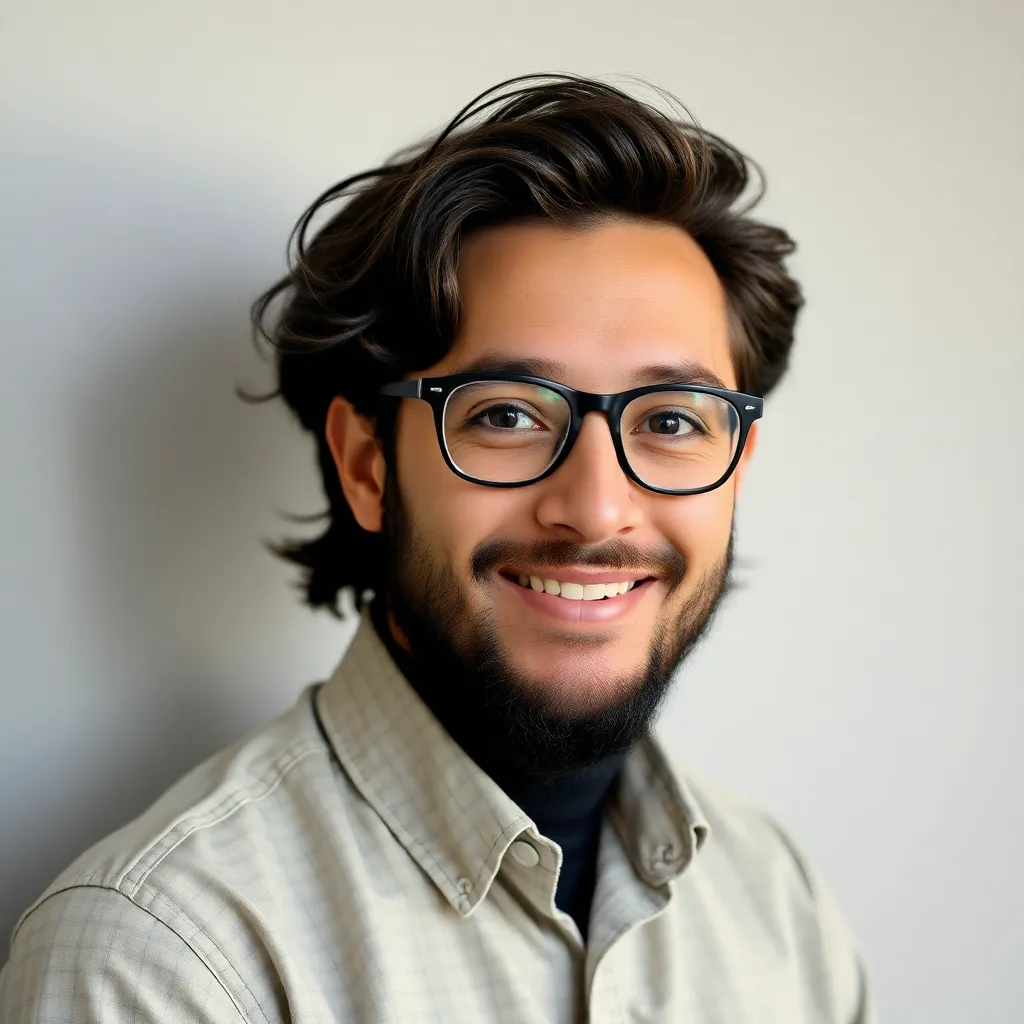
Holbox
May 07, 2025 · 6 min read
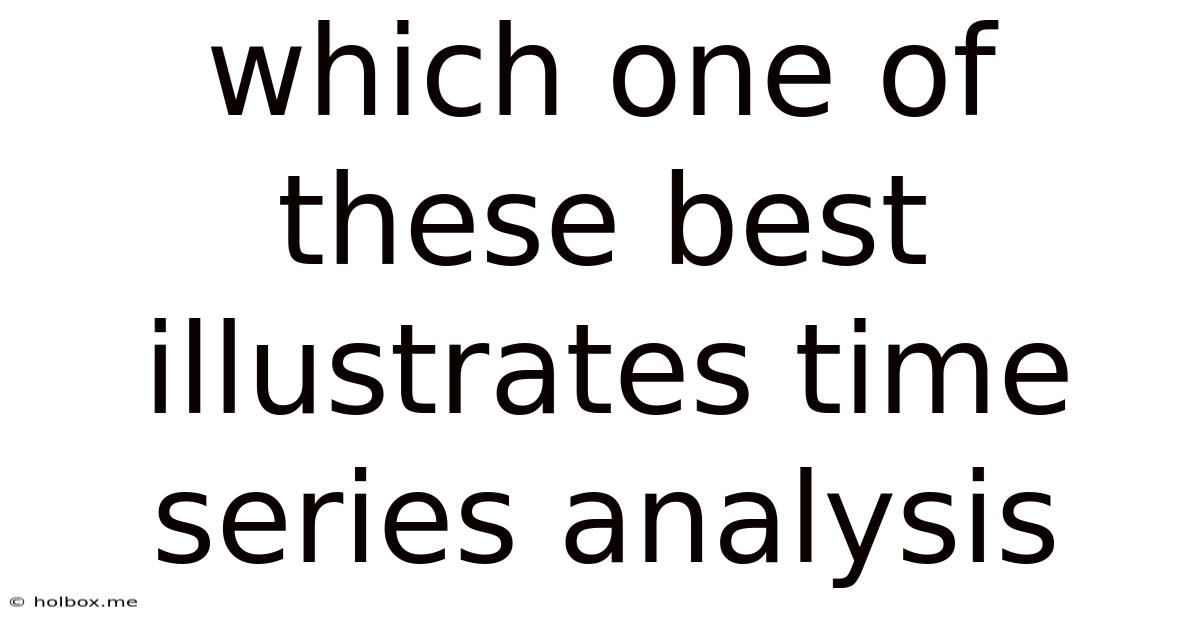
Table of Contents
- Which One Of These Best Illustrates Time Series Analysis
- Table of Contents
- Which One of These Best Illustrates Time Series Analysis?
- Understanding the Essence of Time Series Analysis
- Examples: Which Best Illustrates Time Series Analysis?
- Distinguishing Time Series from Other Statistical Methods
- Advanced Time Series Concepts
- Conclusion
- Latest Posts
- Related Post
Which One of These Best Illustrates Time Series Analysis?
Time series analysis is a powerful statistical technique used to analyze data points collected over time. Understanding which scenarios best exemplify this method is crucial for its effective application. This article delves into various examples, comparing and contrasting them to definitively illustrate what constitutes a prime candidate for time series analysis. We'll explore the key characteristics that make a dataset suitable for this type of analysis and dispel common misconceptions.
Understanding the Essence of Time Series Analysis
Before diving into specific examples, let's establish a foundational understanding. Time series analysis focuses on identifying patterns, trends, and seasonality within data ordered chronologically. The core idea is that the data point at a given time is correlated with data points at preceding and succeeding times. This temporal dependence distinguishes time series analysis from other statistical methods.
Key characteristics of a dataset suitable for time series analysis include:
- Ordered Data: The data must be chronologically ordered. Randomly sequenced data cannot be analyzed using time series methods.
- Temporal Dependence: Successive data points should exhibit correlation or interdependence. The value at one point in time influences (or is influenced by) values at other points.
- Identification of Patterns: The ultimate goal is to uncover underlying patterns, trends (long-term movements), seasonality (regular, short-term fluctuations), and cyclical patterns (longer-term fluctuations).
Examples: Which Best Illustrates Time Series Analysis?
Let's consider several scenarios and assess their suitability for time series analysis.
Scenario 1: Daily Stock Prices of a Specific Company
This is a prime example of a time series. Daily stock prices inherently possess the characteristics we discussed:
- Ordered Data: Prices are recorded daily, creating a clear chronological sequence.
- Temporal Dependence: Today's stock price is highly correlated with yesterday's price, and often influenced by prices over longer periods. Investors consider past performance to inform their decisions.
- Identifiable Patterns: Analysis might reveal upward or downward trends, seasonal fluctuations (e.g., increased activity around quarterly earnings reports), or cyclical patterns reflecting broader economic cycles.
Therefore, daily stock prices strongly illustrate time series analysis. Techniques like ARIMA, exponential smoothing, and GARCH models are frequently used to forecast future stock prices based on past performance.
Scenario 2: Number of Customers Visiting a Store Each Day for a Year
This scenario is also an excellent illustration of time series analysis. The data inherently exhibits:
- Ordered Data: Customer counts are recorded daily, providing a chronological sequence.
- Temporal Dependence: Customer visits on a particular day might be influenced by factors like the day of the week, promotions running, and weather conditions—all of which have a time-dependent element.
- Identifiable Patterns: Analyzing the data might reveal higher customer traffic on weekends, seasonal peaks during holidays, or trends reflecting the store's overall popularity or decline.
Time series methods can help predict future customer footfall, aiding inventory management and staffing decisions.
Scenario 3: The Weights of 100 Individuals at a Specific Point in Time
This scenario is not a good candidate for time series analysis. While the weights are quantifiable data, there's no temporal element. The data represents a snapshot at a single point in time. There's no inherent chronological order or temporal dependence. Although you might analyze the distribution of weights using statistical methods, time series analysis is inappropriate here.
Scenario 4: The Temperature at a Specific Location Every Hour for a Month
This is a strong example of a time series. Hourly temperature readings satisfy all the requirements:
- Ordered Data: Temperatures are recorded every hour, creating a time-ordered sequence.
- Temporal Dependence: Today's temperature is correlated with yesterday's and the previous hours' temperatures, and there are also longer-term seasonal patterns.
- Identifiable Patterns: Analysis can reveal diurnal (daily) variations, seasonal changes across the month, and even long-term trends related to climate change (if the data spans longer periods).
Time series models can effectively forecast future temperatures based on historical data.
Scenario 5: Survey Responses on Customer Satisfaction (with timestamps)
This is a potentially suitable scenario, but its suitability depends on how the data is handled. If the timestamps represent when each response was submitted and the goal is to analyze changes in customer satisfaction over time, then time series analysis could be relevant. However, if the timestamps are merely indicators of when the survey was administered and are not relevant to the core analysis of the satisfaction responses, then a simple statistical analysis of the responses themselves might be sufficient.
The critical factor here is the research question. If the research question centers around the evolution of customer satisfaction over time, time series analysis could be highly valuable.
Scenario 6: The Number of Cars Passing a Certain Point on a Highway Every Hour for a Week
This is another excellent illustration of a time series. Similar to Scenario 4, this dataset is naturally ordered and exhibits temporal dependence and patterns. The number of cars in a given hour will likely be correlated with the number of cars in the preceding and succeeding hours. Analysis could reveal rush hour peaks, daily fluctuations, and perhaps even weekly trends.
Distinguishing Time Series from Other Statistical Methods
It's important to distinguish time series analysis from other statistical methods. While other methods might analyze similar datasets, the focus on temporal dependence and the utilization of specialized models differentiate time series analysis.
For instance, you could analyze the average daily customer count (Scenario 2) using descriptive statistics. However, this approach would ignore the crucial temporal relationships and patterns. Time series analysis goes beyond simple averages, exploring the dynamics and interdependencies over time.
Advanced Time Series Concepts
The examples above primarily focus on relatively straightforward time series. More complex scenarios might involve:
- Multiple Time Series: Analyzing multiple related time series simultaneously, such as stock prices of different companies in the same sector.
- Multivariate Time Series: Considering multiple variables simultaneously, like temperature, humidity, and wind speed.
- Non-stationary Time Series: Series that exhibit trends or seasonality that change over time—requiring more complex modeling techniques.
- Irregularly Spaced Time Series: Data points that aren't collected at regular intervals.
Conclusion
In conclusion, scenarios involving chronologically ordered data exhibiting temporal dependence, such as daily stock prices, hourly temperatures, and daily customer counts, are prime examples illustrating time series analysis. The key lies in identifying the temporal element and its influence on the data's behavior. By carefully evaluating the characteristics of a dataset, you can determine whether time series analysis is the most appropriate and powerful method for uncovering hidden insights and making accurate predictions. Understanding these nuances is critical for effectively employing this valuable statistical technique.
Latest Posts
Related Post
Thank you for visiting our website which covers about Which One Of These Best Illustrates Time Series Analysis . We hope the information provided has been useful to you. Feel free to contact us if you have any questions or need further assistance. See you next time and don't miss to bookmark.