Which Of The Following Is Not A Principle Of Probability
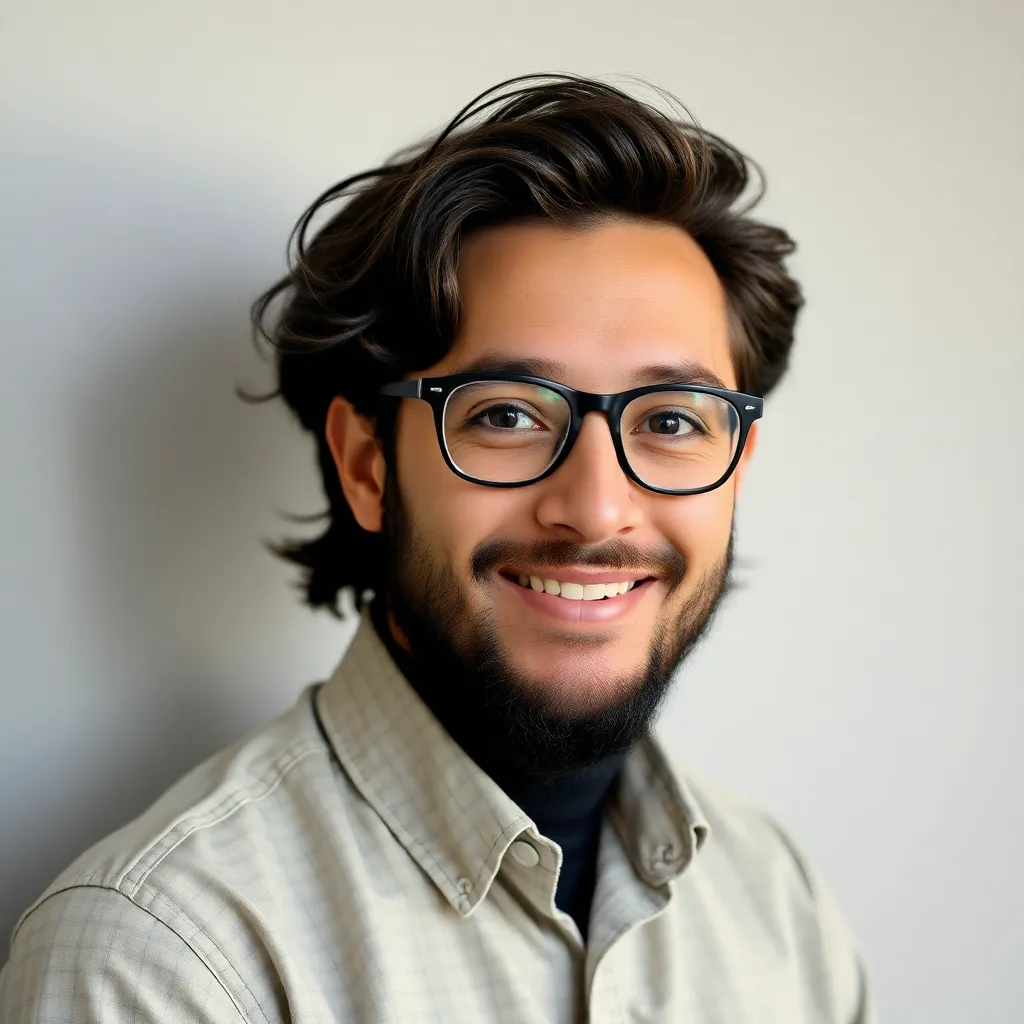
Holbox
May 09, 2025 · 6 min read
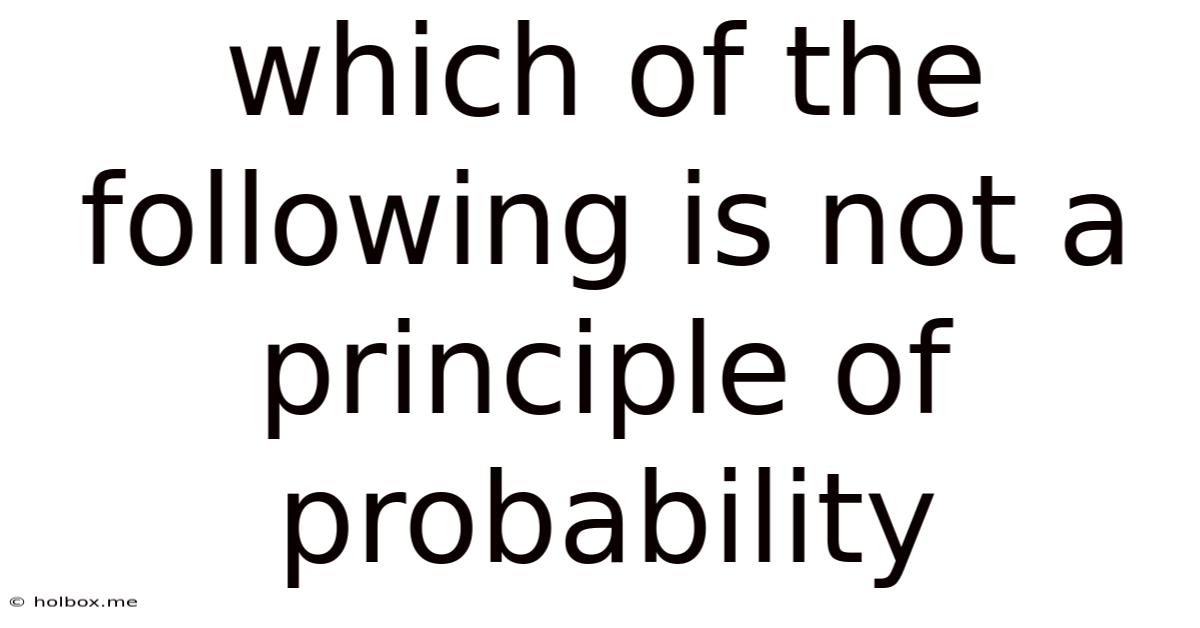
Table of Contents
- Which Of The Following Is Not A Principle Of Probability
- Table of Contents
- Which of the following is NOT a Principle of Probability?
- The Cornerstones of Probability Theory
- 1. The Range of Probability: 0 to 1
- 2. The Law of Large Numbers
- 3. Addition Rule for Mutually Exclusive Events
- 4. Multiplication Rule for Independent Events
- 5. Conditional Probability
- The Imposter: Deterministic Outcomes
- Distinguishing Probability from Determinism: A Deeper Dive
- Common Misconceptions
- Conclusion: Understanding the Foundation
- Latest Posts
- Latest Posts
- Related Post
Which of the following is NOT a Principle of Probability?
Probability, at its core, governs the likelihood of events occurring. Understanding its fundamental principles is crucial in various fields, from statistics and gambling to finance and science. While numerous concepts contribute to a comprehensive understanding of probability, certain core principles form its foundation. This article delves into these fundamental principles, highlighting one crucial concept that does not represent a principle of probability. We will explore these concepts through detailed explanations and relevant examples. By the end, you'll have a solid grasp of probability's building blocks and the common misconceptions surrounding them.
The Cornerstones of Probability Theory
Before identifying the outlier, let's establish the key principles that define probability:
1. The Range of Probability: 0 to 1
This foundational principle dictates that the probability of any event (denoted as P(A) where A represents the event) must fall between 0 and 1, inclusive. A probability of 0 indicates impossibility, meaning the event cannot occur. Conversely, a probability of 1 signifies certainty – the event is guaranteed to happen. Any probability value outside this range is invalid.
Example: The probability of rolling a 7 on a standard six-sided die is 0 (impossible), while the probability of rolling a number less than 7 is 1 (certain). A probability of 1.5 or -0.2 would be incorrect.
2. The Law of Large Numbers
This principle states that as the number of trials or observations increases, the observed frequency of an event will converge towards its theoretical probability. In simpler terms, the more you repeat an experiment, the closer the results will get to the expected outcome based on the probability.
Example: If you flip a fair coin, the probability of getting heads is 0.5. While a few flips might show a disproportionate number of heads or tails, over many thousands of flips, the proportion of heads will approach 50%.
3. Addition Rule for Mutually Exclusive Events
This rule applies when events are mutually exclusive, meaning they cannot occur simultaneously. The probability of either event A or event B happening is the sum of their individual probabilities.
Formula: P(A or B) = P(A) + P(B) (for mutually exclusive events)
Example: The probability of rolling a 1 or a 6 on a six-sided die is P(1) + P(6) = 1/6 + 1/6 = 1/3. These events are mutually exclusive because you cannot roll both a 1 and a 6 at the same time on a single roll.
4. Multiplication Rule for Independent Events
This rule is used when events are independent, meaning the occurrence of one event does not affect the probability of the other. The probability of both events A and B happening is the product of their individual probabilities.
Formula: P(A and B) = P(A) * P(B) (for independent events)
Example: The probability of flipping a head on a coin and then rolling a 3 on a die is (1/2) * (1/6) = 1/12. These events are independent; the outcome of the coin flip doesn't influence the die roll.
5. Conditional Probability
Conditional probability considers the probability of an event given that another event has already occurred. It introduces the concept of dependence between events.
Formula: P(A|B) = P(A and B) / P(B) (probability of A given B)
Example: Let's say we have a bag with 5 red marbles and 5 blue marbles. The probability of drawing a red marble (A) given that you've already drawn a red marble (B) and haven't replaced it is different from the initial probability of drawing a red marble. The probability changes due to the dependence between the events.
The Imposter: Deterministic Outcomes
Now, let's address the concept that isn't a principle of probability: Deterministic Outcomes.
Determinism, in contrast to probability, suggests that events are predetermined and inevitable. There's no element of chance or randomness involved. A deterministic outcome has a probability of 1 (certainty) before the event occurs. It's not a principle governing probabilistic events; instead, it represents the absence of probability.
Examples of Deterministic Outcomes:
- Sunrise: Assuming normal conditions, the sun will rise tomorrow. The probability is 1 – it's a certainty. This is not a probabilistic event; it's a predictable, deterministic event.
- Mathematical Equations: The result of a simple mathematical calculation (e.g., 2 + 2 = 4) is predetermined and not probabilistic.
- Pre-programmed Actions: A robot following a set of instructions will perform those actions predictably; the outcome is deterministic.
The core principles of probability deal with uncertainty and the quantification of likelihood. Deterministic outcomes, on the other hand, eliminate uncertainty – the outcome is already known. They don't fall under the umbrella of probability theory. Attempting to apply probabilistic principles to a deterministic scenario is redundant.
Distinguishing Probability from Determinism: A Deeper Dive
The difference between probability and determinism becomes even clearer when we consider the following:
-
Randomness: Probability fundamentally relies on randomness. Events are subject to chance and unpredictable variations. Determinism excludes randomness. The future state is completely determined by the present state.
-
Uncertainty: Probability quantifies uncertainty. We might not know the exact outcome of an event, but we can assign a probability based on available information. Determinism eliminates uncertainty; the outcome is known with absolute certainty.
-
Prediction: In probability, we make predictions based on the likelihood of events. These predictions involve a degree of uncertainty. In determinism, predictions are certain and accurate, given complete knowledge of the initial conditions.
-
Modeling: Probabilistic models are designed to capture the uncertainty and variability inherent in many real-world phenomena. Deterministic models represent systems where there is no inherent randomness; the outcome is completely predictable.
-
Applications: Probability is used extensively in areas like statistics, risk assessment, machine learning, quantum mechanics (despite apparent randomness at a micro level, the underlying equations are often deterministic), and weather forecasting (although weather is chaotic, models try to capture underlying deterministic processes). Deterministic models are prevalent in classical mechanics, computer science (especially in algorithmic analysis), and many engineering applications where precise control and prediction are crucial.
Common Misconceptions
It's easy to confuse probability with related concepts. Let's address some common misconceptions:
-
Confusing Probability with Frequency: While the law of large numbers links probability to observed frequencies, probability itself is not defined by the frequency of occurrences in a limited number of trials. It represents the theoretical likelihood of an event, regardless of observed frequencies.
-
Assuming Independence When It Doesn't Exist: Many situations involve dependent events, where the outcome of one event influences the probability of another. Failing to account for dependence leads to inaccurate probability calculations.
-
Ignoring Conditional Probability: Forgetting to consider the impact of prior information (conditional probability) can severely distort probability estimations.
Conclusion: Understanding the Foundation
Probability is a powerful tool for understanding and quantifying uncertainty. Mastering its core principles—the range of probability, the law of large numbers, the addition and multiplication rules, and conditional probability—is crucial for accurate analysis and decision-making across a wide range of fields. Remember, deterministic outcomes, characterized by their certainty, stand apart from probabilistic events, which inherently involve uncertainty and the quantification of likelihood. By clearly distinguishing between these concepts, we can more effectively apply the principles of probability and avoid common pitfalls in our analyses.
Latest Posts
Latest Posts
-
What Is 77 Kg In Pounds
May 19, 2025
-
How Many Minutes Is 600 Seconds
May 19, 2025
-
How Much Is 165 Kg In Pounds
May 19, 2025
-
How Many Days Are In 60 Years
May 19, 2025
-
How Many Oz Is 700 Ml
May 19, 2025
Related Post
Thank you for visiting our website which covers about Which Of The Following Is Not A Principle Of Probability . We hope the information provided has been useful to you. Feel free to contact us if you have any questions or need further assistance. See you next time and don't miss to bookmark.