Which Of The Following Is Incorrectly Matched
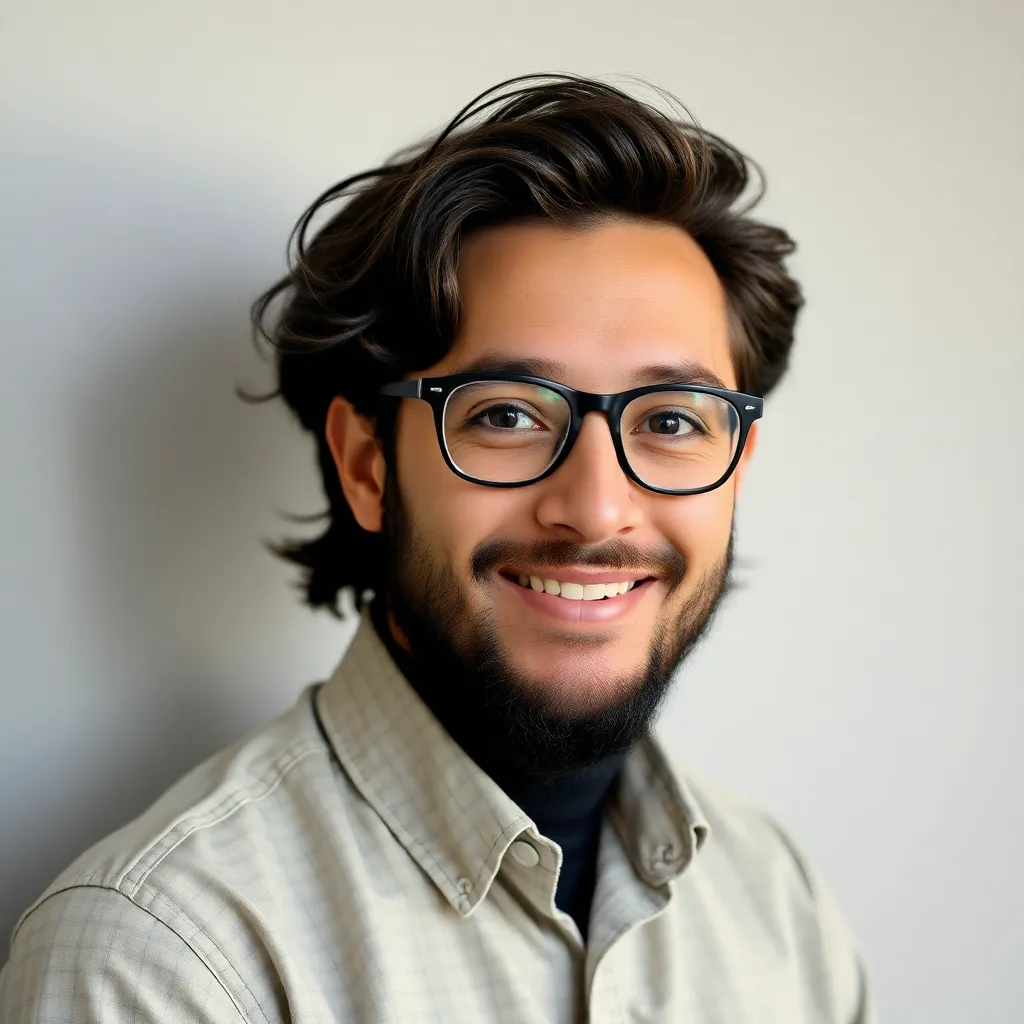
Holbox
Apr 07, 2025 · 6 min read

Table of Contents
- Which Of The Following Is Incorrectly Matched
- Table of Contents
- Which of the Following is Incorrectly Matched? A Deep Dive into Identifying Errors in Paired Data
- Understanding Different Types of Mismatches
- Strategies for Identifying Incorrectly Matched Pairs
- Practical Examples and Case Studies
- Minimizing Mismatches: Proactive Strategies
- Conclusion
- Latest Posts
- Latest Posts
- Related Post
Which of the Following is Incorrectly Matched? A Deep Dive into Identifying Errors in Paired Data
Identifying incorrectly matched pairs is a crucial skill across numerous fields, from data analysis and scientific research to educational assessment and even everyday problem-solving. This article will explore the intricacies of this task, providing practical strategies and examples to help you confidently pinpoint discrepancies in paired data sets. We'll move beyond simple recognition to understanding the why behind mismatches, equipping you to not only identify errors but also to prevent them in the future.
This seemingly simple task – identifying incorrectly matched pairs – underpins much more complex processes. Consider these scenarios:
- Data Analysis: Incorrectly matched data points can skew statistical analysis, leading to inaccurate conclusions and flawed decision-making. Imagine a study comparing customer demographics with purchasing habits. A mismatch in customer IDs would distort the relationships identified.
- Scientific Research: In experimental settings, mismatched samples (e.g., incorrect labeling of control and experimental groups) can invalidate the entire experiment. This is especially critical in fields like medicine and pharmaceuticals.
- Educational Assessment: Incorrectly paired student responses with their identifying information can lead to inaccurate grading and assessment of learning outcomes.
- Database Management: In databases, incorrect pairings (e.g., linking a customer to the wrong order) can cause significant operational problems and financial losses.
Understanding Different Types of Mismatches
Before we delve into identification strategies, let's categorize the types of mismatches you might encounter:
1. Simple Mismatches: These are straightforward errors where two items intended to be paired are incorrectly linked. For example:
- Name-Address Mismatch: A database listing "John Doe" with the incorrect address.
- Order-Customer Mismatch: An online order mistakenly assigned to the wrong customer account.
- Image-Caption Mismatch: An image of a cat incorrectly captioned as a dog.
2. Complex Mismatches: These involve multiple factors and require more sophisticated analysis to identify. For instance:
- Temporal Mismatches: Data points recorded at the wrong time or in the wrong order. This is common in time-series data.
- Spatial Mismatches: Data points incorrectly associated with a specific location. Geographical information systems (GIS) often deal with this type of mismatch.
- Logical Mismatches: Data pairs that violate established rules or relationships. For example, a database entry showing a person under the age of 16 owning a car.
3. Systemic Mismatches: These are recurring errors stemming from a flaw in the data collection or processing system. Identifying systemic mismatches requires a thorough examination of the entire process to pinpoint the root cause. This might involve reviewing data entry procedures, software bugs, or inconsistencies in data standards.
Strategies for Identifying Incorrectly Matched Pairs
The best strategy for identifying mismatches depends on the nature and volume of data. Let’s explore several approaches:
1. Visual Inspection: This is the simplest method, ideal for smaller datasets. Carefully examining each pair allows for the direct identification of obvious errors. However, it's time-consuming and prone to human error for larger datasets.
2. Data Sorting and Filtering: Sorting data by relevant fields (e.g., sorting customer orders by date) can reveal inconsistencies or anomalies that indicate mismatches. Filtering based on specific criteria (e.g., filtering for orders with unusually high values) can also highlight potential errors.
3. Data Validation Rules: Establishing rules and constraints for data entry can prevent many mismatches from occurring in the first place. For instance, a rule could ensure that a customer's age is always a positive integer. Data validation checks can identify violations of these rules.
4. Data Deduplication: This technique identifies and removes duplicate records, which can often be indicators of mismatches. For instance, duplicate customer entries with slightly different spellings of their name might suggest a data entry error.
5. Statistical Analysis: For larger datasets, statistical methods can be used to detect outliers or inconsistencies that might indicate mismatches. These methods can identify data points that deviate significantly from the overall pattern. For example, anomaly detection algorithms can help identify unusual purchasing behaviors that might indicate a mismatch in customer data.
6. Machine Learning Techniques: Advanced machine learning algorithms, particularly those used in anomaly detection and classification, can be very effective in identifying mismatches, especially in complex datasets. These algorithms can learn patterns in correctly matched data and flag instances that deviate from those patterns. This is especially useful for large datasets and identifying subtle inconsistencies.
7. Cross-Referencing: Comparing the data with other sources of information can help identify errors. For example, verifying customer information by checking against a separate customer database can help pinpoint mismatches.
Practical Examples and Case Studies
Let's consider a few practical scenarios:
Example 1: Student Test Scores and IDs
Imagine a spreadsheet of student test scores where each row represents a student, with columns for student ID, name, and score. A visual inspection or sorting by student ID might reveal that two students have the same ID, suggesting a duplication or mismatch. Similarly, discrepancies between the name and ID could indicate an incorrectly assigned score.
Example 2: E-commerce Order Processing
An e-commerce platform needs to ensure accurate order fulfillment. Mismatches between customer IDs and order details can lead to incorrect shipping addresses or product deliveries. Data validation rules, such as checking for valid addresses and ensuring the order total matches the payment received, can help prevent and identify these errors.
Example 3: Medical Research Study
In a medical research study comparing the effectiveness of two treatments, mismatched patient data (e.g., assigning a patient to the wrong treatment group) could bias the results. Careful data entry procedures, double-checking of data, and potentially using unique patient identifiers can minimize such errors.
Minimizing Mismatches: Proactive Strategies
Preventing mismatches is crucial. Here are some proactive strategies:
- Standardized Data Entry Procedures: Establishing clear and consistent procedures for data entry minimizes the risk of human error. This includes using standardized formats and clear instructions.
- Data Validation: Implementing robust data validation rules ensures that data conforms to expected formats and constraints.
- Data Cleaning: Regular data cleaning helps identify and correct inconsistencies and errors in the data.
- Automated Data Checks: Automating data checks, such as cross-referencing data against multiple sources, can help catch mismatches early on.
- Data Version Control: Keeping track of changes made to the data allows for easy rollback if errors are detected.
- Regular Audits: Periodic audits of data quality can help identify systemic issues leading to mismatches.
Conclusion
Identifying incorrectly matched pairs is a critical task with significant implications across various fields. By understanding the different types of mismatches and employing appropriate identification and prevention strategies, individuals and organizations can enhance data quality, improve decision-making, and ultimately minimize the impact of errors. The approach chosen depends on the nature and volume of data, ranging from simple visual inspection to sophisticated machine learning techniques. Proactive measures such as standardized data entry, robust data validation, and regular data audits are essential for preventing these errors from occurring in the first place. By implementing these strategies, you can ensure data accuracy and reliability, forming a solid foundation for informed decisions and accurate analysis.
Latest Posts
Latest Posts
-
For Companies Using Lifo Inventory Is Valued At
Apr 13, 2025
-
Consider The Coils Depicted In The Figure
Apr 13, 2025
-
A Phone App Functions As A Phone
Apr 13, 2025
-
Draw The Correct Bond Line Structure For The Following Compound
Apr 13, 2025
-
A Ladder Is Leaning Against A Vertical Wall
Apr 13, 2025
Related Post
Thank you for visiting our website which covers about Which Of The Following Is Incorrectly Matched . We hope the information provided has been useful to you. Feel free to contact us if you have any questions or need further assistance. See you next time and don't miss to bookmark.