Which Of The Following Is An Example Of Inductive Reasoning
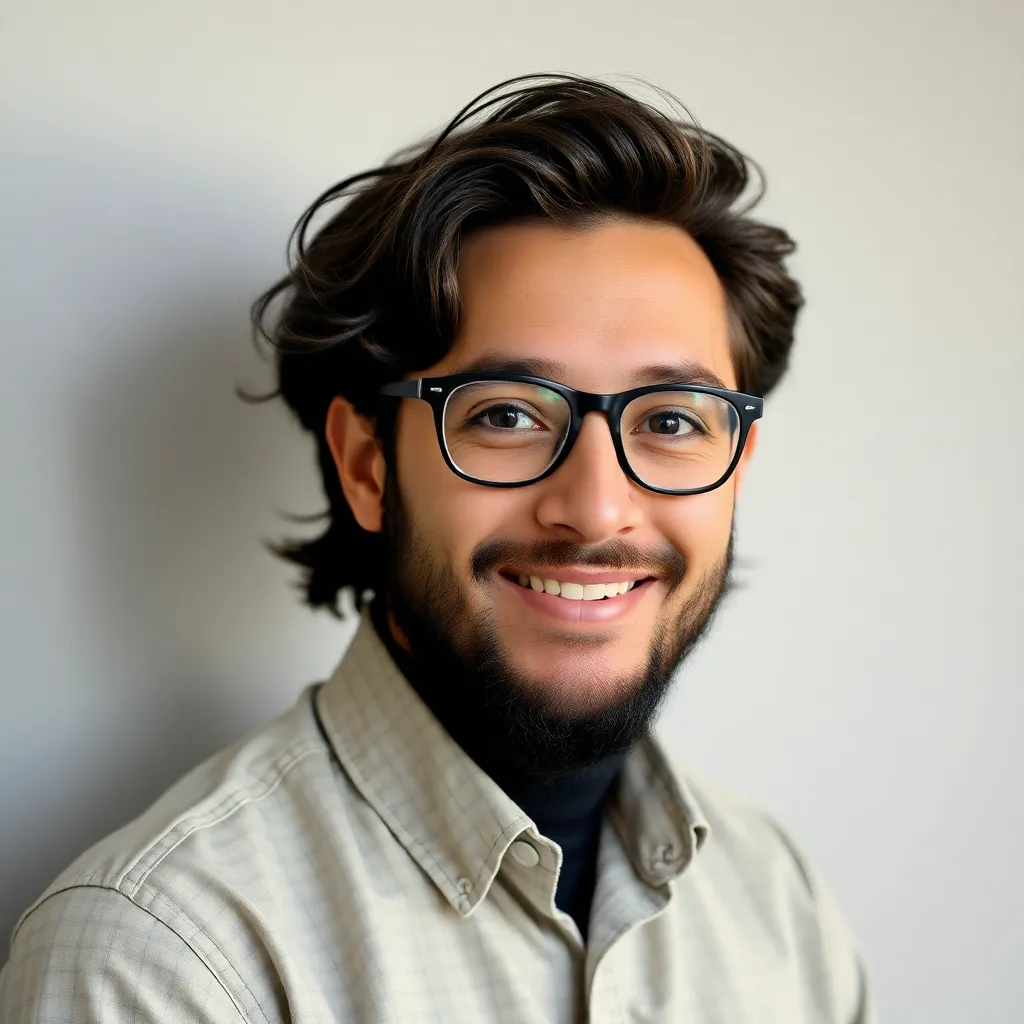
Holbox
May 08, 2025 · 7 min read
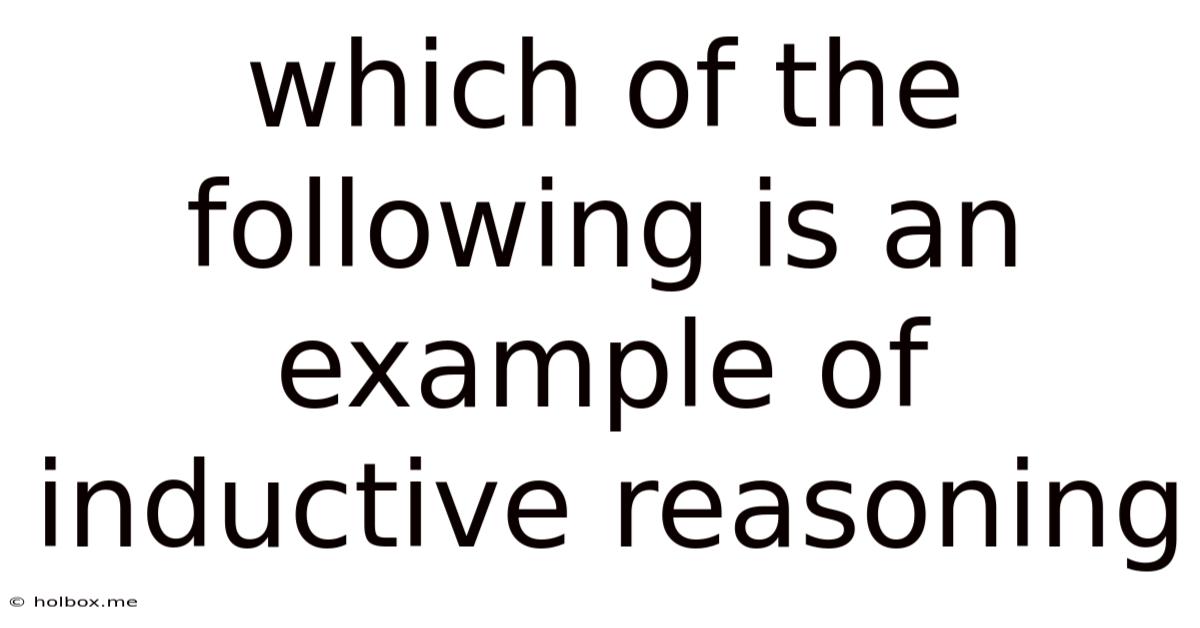
Table of Contents
- Which Of The Following Is An Example Of Inductive Reasoning
- Table of Contents
- Which of the Following is an Example of Inductive Reasoning? Understanding and Applying Inductive Logic
- Defining Inductive Reasoning: From Specifics to Generalities
- The Contrast with Deductive Reasoning: Top-Down vs. Bottom-Up
- Types of Inductive Reasoning: A Diverse Landscape
- 1. Generalization: Drawing Broad Conclusions
- 2. Statistical Induction: Probability and Inference
- 3. Causal Inference: Establishing Cause-and-Effect Relationships
- 4. Analogical Reasoning: Drawing Parallels and Comparisons
- 5. Predictive Reasoning: Forecasting Future Events
- Evaluating Inductive Arguments: Strengths and Weaknesses
- Examples of Inductive Reasoning in Different Contexts
- Science: The Foundation of Scientific Discovery
- Medicine: Diagnosing Illnesses and Developing Treatments
- Everyday Life: Making Decisions and Solving Problems
- Common Pitfalls in Inductive Reasoning: Avoiding Errors
- Strengthening Inductive Arguments: Best Practices
- Conclusion: The Power and Limitations of Inductive Reasoning
- Latest Posts
- Latest Posts
- Related Post
Which of the Following is an Example of Inductive Reasoning? Understanding and Applying Inductive Logic
Inductive reasoning is a cornerstone of scientific inquiry, everyday decision-making, and creative problem-solving. Unlike deductive reasoning, which moves from general principles to specific conclusions, inductive reasoning starts with specific observations and moves toward broader generalizations. This process is often probabilistic rather than certain; the conclusions are likely, but not guaranteed. Understanding inductive reasoning is crucial for critical thinking and effective communication. This article will delve into the nature of inductive reasoning, differentiating it from deductive reasoning, exploring its various types, and providing examples to solidify understanding. We'll also address common pitfalls and strategies for strengthening inductive arguments.
Defining Inductive Reasoning: From Specifics to Generalities
Inductive reasoning, at its core, is the process of drawing general conclusions from specific observations. It's a bottom-up approach, where evidence gathered from individual instances leads to the formulation of a hypothesis or theory. The strength of an inductive argument depends heavily on the quality and quantity of evidence presented. A robust inductive argument will provide ample, relevant, and representative data to support its conclusion.
The Contrast with Deductive Reasoning: Top-Down vs. Bottom-Up
To fully grasp inductive reasoning, it's essential to contrast it with its counterpart: deductive reasoning. Deductive reasoning is a top-down approach; it begins with established general principles (premises) and deduces specific conclusions. If the premises are true and the logic is sound, the conclusion must also be true. For example:
- Premise 1: All men are mortal.
- Premise 2: Socrates is a man.
- Conclusion: Therefore, Socrates is mortal.
This is a deductive argument; the conclusion is guaranteed given the truth of the premises. Inductive reasoning, on the other hand, does not offer such certainty. The conclusion is probable, based on the available evidence, but it could be false even if the premises are true.
Types of Inductive Reasoning: A Diverse Landscape
Inductive reasoning isn't a monolithic process; it encompasses several distinct types, each with its own characteristics and applications:
1. Generalization: Drawing Broad Conclusions
Generalization is the most common form of inductive reasoning. It involves observing a sample and inferring that the same pattern will hold true for the larger population. For example:
- Observation: Every swan I've ever seen is white.
- Conclusion: Therefore, all swans are white.
This is a classic example of generalization. While the premise might seem true based on limited observations, it's famously false (black swans exist). This highlights the inherent uncertainty in inductive reasoning. The strength of a generalization depends on the size and representativeness of the sample. A larger, more diverse sample is more likely to yield a reliable generalization.
2. Statistical Induction: Probability and Inference
Statistical induction uses statistical data to draw conclusions about a population. It relies on probabilities and the laws of chance. For example:
- Data: 90% of students who study regularly pass the exam.
- Conclusion: Therefore, there's a high probability that a student who studies regularly will pass the exam.
This type of inductive reasoning uses statistical data to make predictions about future events. The certainty of the conclusion depends on the accuracy and reliability of the statistical data.
3. Causal Inference: Establishing Cause-and-Effect Relationships
Causal inference seeks to establish cause-and-effect relationships between events. It's a complex process that requires careful consideration of correlation versus causation. For example:
- Observation: Every time I water the plant, it grows taller.
- Conclusion: Therefore, watering the plant causes it to grow taller.
While correlation (a relationship between two variables) is observed, it doesn't necessarily imply causation. Other factors could be influencing the plant's growth. Stronger causal inferences require controlling for confounding variables and establishing a clear temporal sequence (cause precedes effect).
4. Analogical Reasoning: Drawing Parallels and Comparisons
Analogical reasoning involves drawing conclusions based on similarities between different situations or objects. It's often used in creative problem-solving and scientific modeling. For example:
- Premise: The human heart is like a pump.
- Conclusion: Therefore, understanding how a pump works can help us understand how the heart works.
Analogical reasoning is heuristic; it offers a plausible explanation but doesn't guarantee its truth. The strength of an analogical argument depends on the relevance and extent of the similarities between the compared entities.
5. Predictive Reasoning: Forecasting Future Events
Predictive reasoning uses past observations to predict future outcomes. It's widely used in forecasting, weather prediction, and market analysis. For example:
- Observation: The stock market has historically risen after periods of low interest rates.
- Conclusion: Therefore, it's likely the stock market will rise following the current period of low interest rates.
Predictive reasoning relies on identifying patterns and trends in past data to make informed guesses about the future. However, unpredictable events can render these predictions inaccurate.
Evaluating Inductive Arguments: Strengths and Weaknesses
Not all inductive arguments are created equal. The strength of an inductive argument is determined by several factors:
- Quantity of Evidence: More evidence generally strengthens an inductive argument.
- Quality of Evidence: Reliable, relevant, and representative evidence is crucial.
- Absence of Counterexamples: The lack of contradictory evidence strengthens the argument.
- Consistency with Other Knowledge: The argument should align with existing knowledge.
Examples of Inductive Reasoning in Different Contexts
To further clarify, let’s explore specific examples of inductive reasoning across diverse fields:
Science: The Foundation of Scientific Discovery
Inductive reasoning is the engine of scientific discovery. Scientists gather data through observation and experimentation, then formulate hypotheses and theories based on patterns in the data. The discovery of the laws of motion by Isaac Newton is a prime example. He observed the movement of celestial bodies and terrestrial objects and induced the laws of motion that govern their behavior. This process is constantly refined as new evidence emerges.
Medicine: Diagnosing Illnesses and Developing Treatments
Doctors use inductive reasoning to diagnose illnesses. Based on symptoms, medical history, and test results, they formulate a diagnosis. A patient presenting with fever, cough, and fatigue might be diagnosed with influenza, based on the doctor's experience and knowledge of common illnesses.
Everyday Life: Making Decisions and Solving Problems
We use inductive reasoning countless times daily. Deciding whether to bring an umbrella based on the weather forecast, inferring a friend's mood from their facial expression, or choosing a restaurant based on online reviews are all examples of everyday inductive reasoning.
Common Pitfalls in Inductive Reasoning: Avoiding Errors
Several pitfalls can lead to weak or flawed inductive arguments:
- Hasty Generalization: Drawing a broad conclusion from insufficient evidence.
- Confirmation Bias: Seeking only evidence that confirms existing beliefs and ignoring contradictory evidence.
- False Analogy: Comparing dissimilar things and drawing inappropriate conclusions.
- Post Hoc Ergo Propter Hoc: Assuming that because one event follows another, the first event caused the second.
Strengthening Inductive Arguments: Best Practices
To enhance the strength and validity of inductive arguments, consider the following strategies:
- Increase Sample Size: Use a larger and more diverse sample to make generalizations.
- Improve Sample Representativeness: Ensure the sample accurately reflects the larger population.
- Control for Confounding Variables: Identify and control factors that might influence the results.
- Seek Diverse Perspectives: Consider alternative explanations and viewpoints.
- Acknowledge Uncertainty: Recognize the inherent probabilistic nature of inductive conclusions.
Conclusion: The Power and Limitations of Inductive Reasoning
Inductive reasoning is a powerful tool for exploring the world around us, building knowledge, and making informed decisions. Its ability to generate hypotheses and generalizations is essential for scientific advancement, problem-solving, and everyday life. However, it's crucial to understand its limitations. Inductive conclusions are probabilistic rather than certain, and the strength of an argument depends on the quality and quantity of evidence. By understanding the nature of inductive reasoning, its various types, and common pitfalls, we can enhance our critical thinking skills and build stronger, more persuasive arguments. The ability to effectively utilize inductive reasoning is a skill that can be honed through practice and mindful application.
Latest Posts
Latest Posts
-
What Is 74 Degrees Celsius In Fahrenheit
May 18, 2025
-
How Many Ounces Is 156 Ml
May 18, 2025
-
90 Square Meters In Square Feet
May 18, 2025
-
How Many Ounces Is 900 Ml
May 18, 2025
-
How Many Days Is 96 Hours
May 18, 2025
Related Post
Thank you for visiting our website which covers about Which Of The Following Is An Example Of Inductive Reasoning . We hope the information provided has been useful to you. Feel free to contact us if you have any questions or need further assistance. See you next time and don't miss to bookmark.