Which Of The Following Is A Measure Of Central Tendency
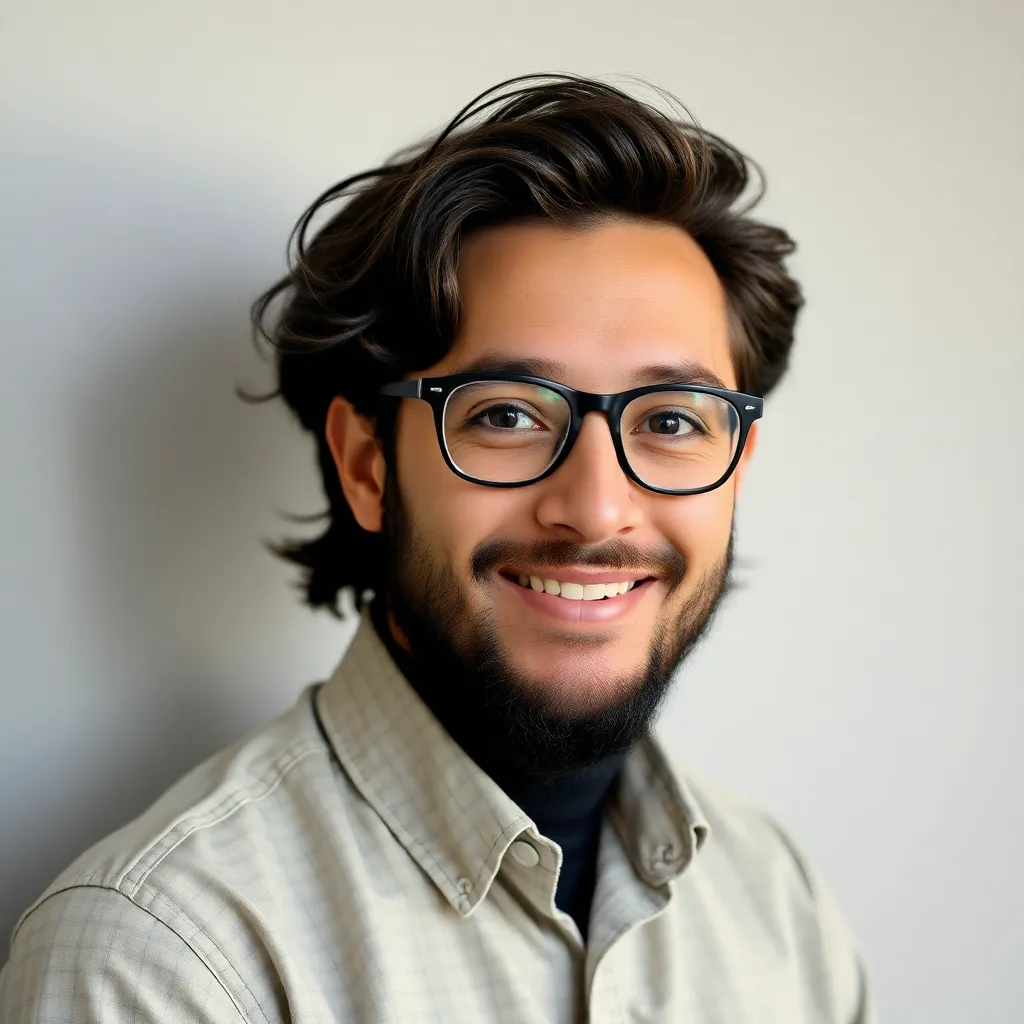
Holbox
May 10, 2025 · 6 min read
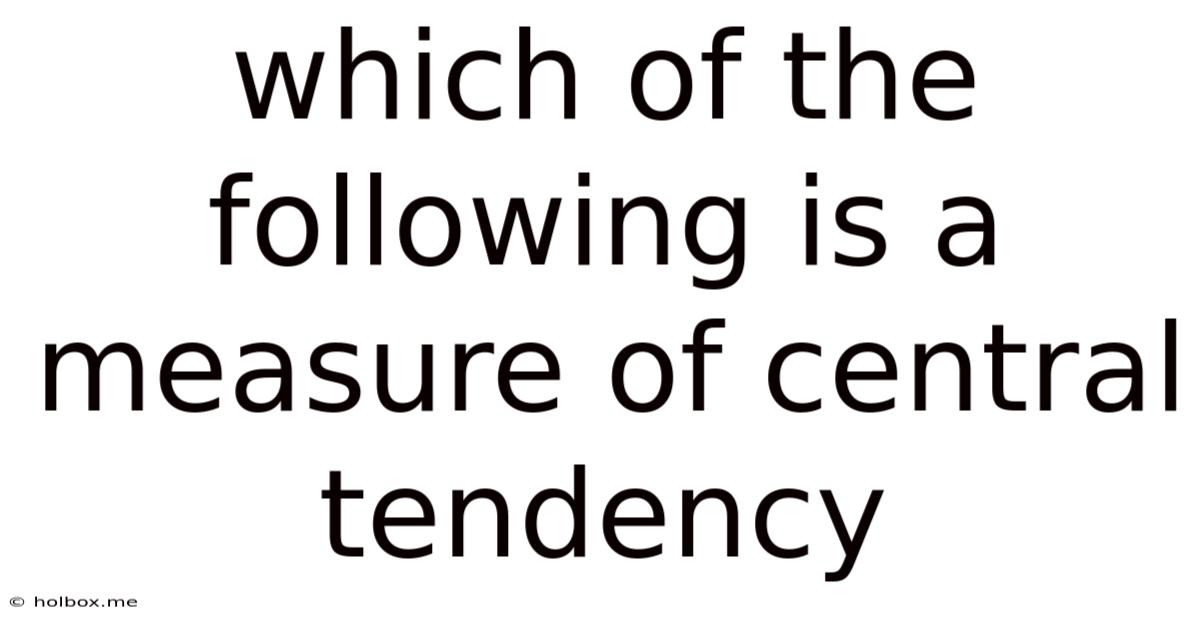
Table of Contents
- Which Of The Following Is A Measure Of Central Tendency
- Table of Contents
- Which of the Following is a Measure of Central Tendency? A Deep Dive into Mean, Median, and Mode
- What is Central Tendency?
- The Three Main Measures of Central Tendency
- 1. The Mean (Average)
- 2. The Median
- 3. The Mode
- Choosing the Right Measure
- Beyond the Basics: Understanding Data Distributions
- Advanced Considerations: Trimmed Mean and Winsorized Mean
- Conclusion: A Powerful Tool for Data Interpretation
- Latest Posts
- Related Post
Which of the Following is a Measure of Central Tendency? A Deep Dive into Mean, Median, and Mode
Understanding central tendency is crucial in statistics. It helps us summarize and interpret data by identifying the "center" or typical value of a dataset. But what exactly is central tendency, and which measures best represent it? This comprehensive guide explores the three primary measures – mean, median, and mode – explaining their calculations, applications, and limitations. We'll also delve into situations where one measure might be preferred over another, equipping you with the knowledge to choose the most appropriate measure for your data analysis.
What is Central Tendency?
Central tendency refers to the single value that attempts to describe a dataset by identifying the central position within that set of data. It's a way to condense a large amount of information into a single, representative number. This single value can then be used for comparison, interpretation, and further analysis. Think of it as finding the "average" or "typical" value. However, the method used to find this "average" depends on the type of data and the desired information.
The Three Main Measures of Central Tendency
Three primary measures capture central tendency: the mean, the median, and the mode. Each offers a unique perspective on the data, making understanding their nuances essential.
1. The Mean (Average)
The mean is the most commonly used measure of central tendency. It's calculated by summing all the values in a dataset and then dividing by the number of values. This is also known as the arithmetic mean.
Formula:
Mean = Σx / n
Where:
- Σx represents the sum of all values in the dataset.
- n represents the number of values in the dataset.
Example:
Consider the dataset: 2, 4, 6, 8, 10
Mean = (2 + 4 + 6 + 8 + 10) / 5 = 6
Advantages of using the Mean:
- Familiar and easily understood: Most people are comfortable with the concept of an average.
- Simple to calculate: The calculation is straightforward.
- Uses all data points: It considers every value in the dataset.
- Good for symmetrical distributions: It accurately represents the center of a symmetrical distribution.
Disadvantages of using the Mean:
- Sensitive to outliers: Extreme values (outliers) can significantly skew the mean, making it a poor representation of the central tendency. For instance, if we add the value 100 to the previous dataset, the mean jumps to 24, which is not representative of most of the data points.
- Not suitable for skewed distributions: In datasets with skewed distributions (where data is heavily concentrated on one side), the mean may not accurately reflect the typical value.
- Cannot be used with categorical data: The mean is only applicable to numerical data.
2. The Median
The median is the middle value in a dataset when the data is ordered from least to greatest. If the dataset has an even number of values, the median is the average of the two middle values.
Example:
- Odd number of values: Dataset: 2, 4, 6, 8, 10. The median is 6.
- Even number of values: Dataset: 2, 4, 6, 8. The median is (4 + 6) / 2 = 5.
Advantages of using the Median:
- Robust to outliers: Outliers have less impact on the median than on the mean. In the example above, adding 100 to the dataset would still leave the median at 6 (or close to 6 if the dataset is larger) better reflecting the central tendency.
- Suitable for skewed distributions: The median is a better measure of central tendency for skewed distributions.
- Can be used with ordinal data: The median can be used with data that has a clear order (e.g., rankings).
Disadvantages of using the Median:
- Ignores some data points: It only considers the middle value(s), disregarding the magnitude of other values.
- Can be less precise than the mean: For symmetrical distributions, the mean provides more precise information about the center.
- More complex calculation for large datasets: Sorting large datasets can be time-consuming.
3. The Mode
The mode is the value that appears most frequently in a dataset. A dataset can have one mode (unimodal), two modes (bimodal), or multiple modes (multimodal). If all values occur with the same frequency, there is no mode.
Example:
Dataset: 2, 4, 4, 6, 8, 8, 8, 10. The mode is 8.
Advantages of using the Mode:
- Simple to identify: The mode is easily found by inspecting the dataset.
- Unaffected by outliers: Extreme values don't influence the mode.
- Can be used with categorical data: The mode can be applied to both numerical and categorical data (e.g., finding the most popular color).
Disadvantages of using the Mode:
- May not be unique: A dataset can have multiple modes or no mode at all.
- Ignores most data points: It only considers the most frequent value, neglecting other values in the dataset.
- Less informative than mean and median: It only provides information about the most frequent value, not the central tendency overall.
Choosing the Right Measure
The best measure of central tendency depends on the specific characteristics of the dataset and the research question.
- Symmetrical distribution with no outliers: The mean is usually the best choice.
- Skewed distribution or presence of outliers: The median is generally preferred as it is more resistant to outliers and provides a more robust measure of central tendency.
- Categorical data or identification of the most frequent value: The mode is the appropriate measure.
Beyond the Basics: Understanding Data Distributions
The choice of central tendency measure is also heavily influenced by the distribution of your data. Understanding if your data is normally distributed (bell-shaped curve), skewed (leaning to one side), or otherwise patterned is critical.
- Normal Distribution: In a normal distribution, the mean, median, and mode are all approximately equal.
- Positively Skewed Distribution: In a positively skewed distribution, the mean is greater than the median, and the median is greater than the mode. This is because the tail of the distribution extends to the right. The median is usually a better representation of the central tendency in this case.
- Negatively Skewed Distribution: In a negatively skewed distribution, the mean is less than the median, and the median is less than the mode. The tail extends to the left. Again, the median is often preferred.
Visualizing your data using histograms or box plots can help determine the shape of the distribution and aid in selecting the most appropriate measure of central tendency.
Advanced Considerations: Trimmed Mean and Winsorized Mean
For datasets with outliers that significantly skew the mean, alternative measures like the trimmed mean and Winsorized mean can be used.
- Trimmed Mean: A certain percentage of the highest and lowest values are removed from the dataset before calculating the mean. This reduces the influence of outliers.
- Winsorized Mean: The highest and lowest values are replaced with less extreme values (often the highest and lowest values within the remaining percentage) before calculating the mean.
These methods provide a compromise between the sensitivity of the mean to outliers and the robustness of the median.
Conclusion: A Powerful Tool for Data Interpretation
Understanding the nuances of mean, median, and mode is fundamental to effective data analysis. While the mean is often the first measure that comes to mind, choosing the appropriate measure depends on several factors, including the distribution of the data and the presence of outliers. By carefully considering the strengths and weaknesses of each measure, you can accurately represent the central tendency of your data and draw meaningful conclusions. Remember to always visualize your data to gain a better understanding of its distribution before selecting a measure of central tendency. The correct choice will greatly improve the accuracy and reliability of your analysis and conclusions.
Latest Posts
Related Post
Thank you for visiting our website which covers about Which Of The Following Is A Measure Of Central Tendency . We hope the information provided has been useful to you. Feel free to contact us if you have any questions or need further assistance. See you next time and don't miss to bookmark.