What Is The Difference Between Univariate Data And Bivariate Data
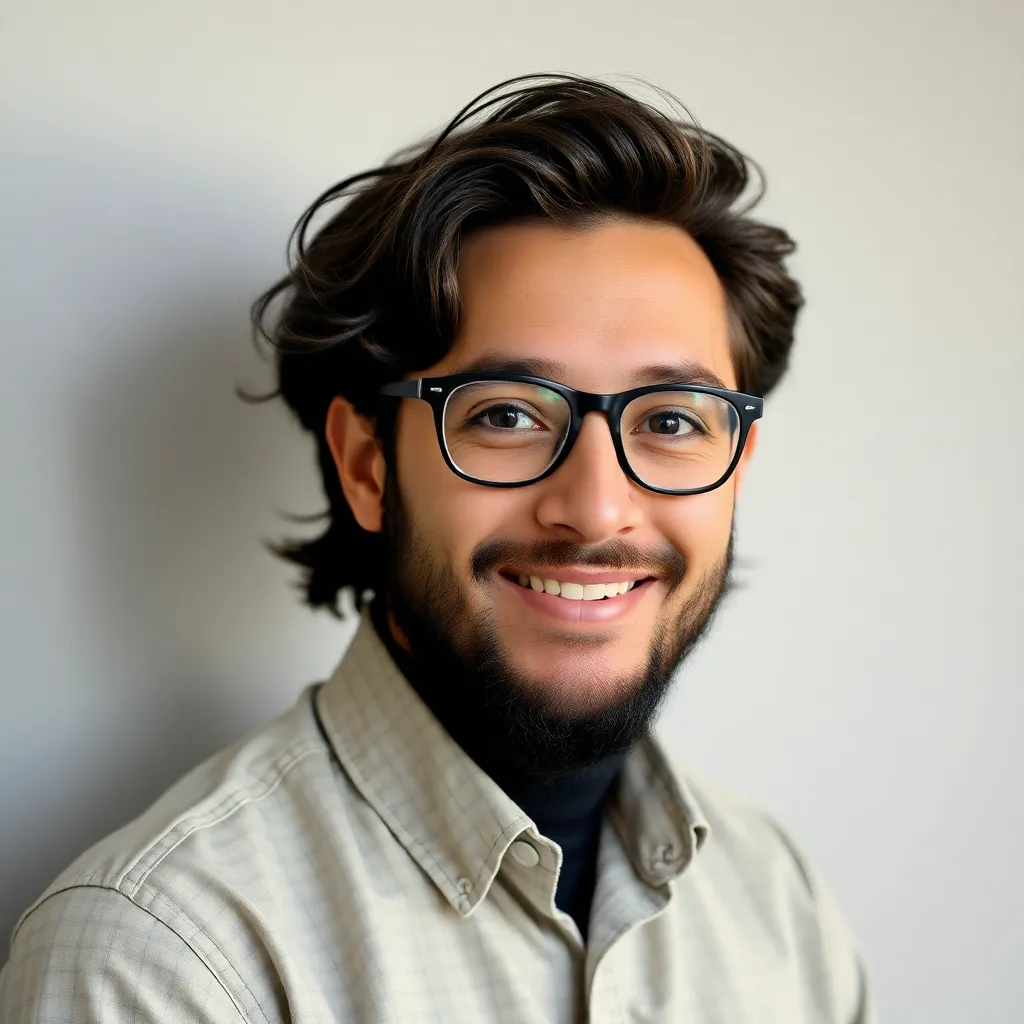
Holbox
May 10, 2025 · 7 min read
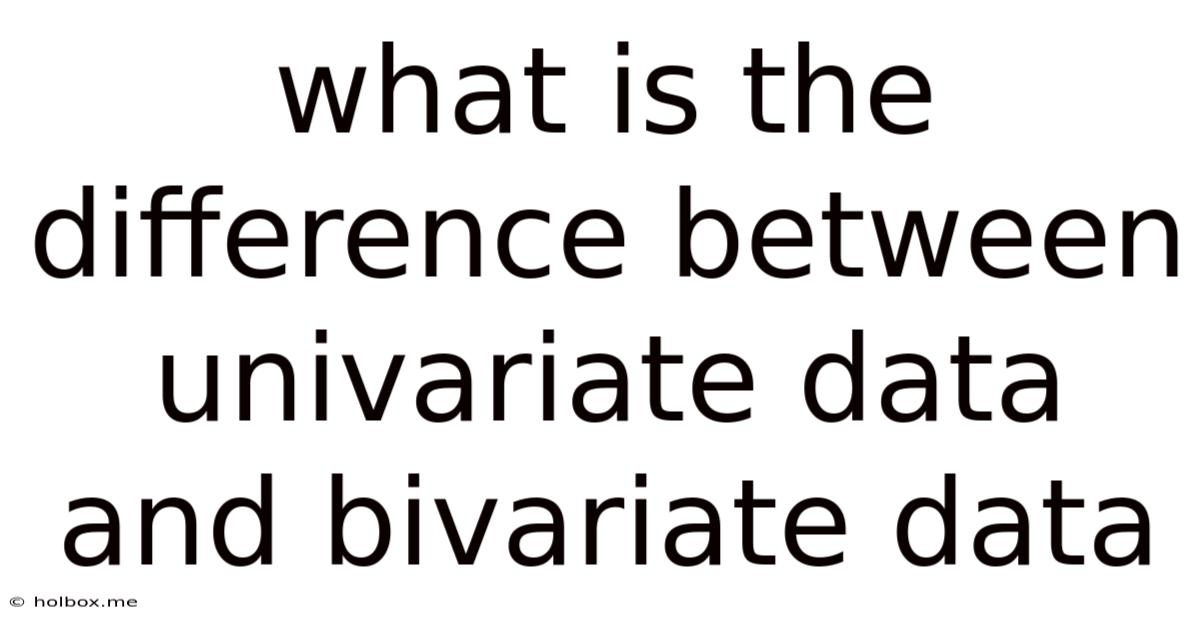
Table of Contents
- What Is The Difference Between Univariate Data And Bivariate Data
- Table of Contents
- Delving into Data: Unveiling the Differences Between Univariate and Bivariate Data
- What is Univariate Data?
- Analyzing Univariate Data
- Visualizing Univariate Data
- What is Bivariate Data?
- Analyzing Bivariate Data
- Visualizing Bivariate Data
- Key Differences Between Univariate and Bivariate Data
- Practical Applications
- Advanced Concepts and Considerations
- Conclusion
- Latest Posts
- Related Post
Delving into Data: Unveiling the Differences Between Univariate and Bivariate Data
Understanding the nuances of data analysis is crucial for anyone working with information, whether you're a seasoned data scientist or a curious student. This article dives deep into the fundamental difference between two core data types: univariate data and bivariate data. We'll explore their definitions, characteristics, analytical approaches, and practical applications, providing a comprehensive guide to help you confidently navigate the world of data.
What is Univariate Data?
Univariate data is the simplest form of data. It involves only one variable. This single variable is measured or observed, and the analysis focuses solely on understanding its distribution, central tendency, and dispersion. Think of it as examining a single aspect of something. Instead of looking at multiple things at once, we zoom in on just one.
Examples of Univariate Data:
- Height of students in a class: The data set would contain only the height of each student, with no other variables considered.
- Daily temperature in a city: The data would consist of the temperature recorded each day, without considering other factors like rainfall or humidity.
- Number of cars sold by a dealership each month: This dataset only tracks the number of cars sold monthly.
- The age of participants in a survey: This would be a list of ages without any other associated information.
- Scores on a single exam: This involves only the scores obtained by students on a particular exam.
Analyzing Univariate Data
Analyzing univariate data primarily involves descriptive statistics. We look at measures such as:
- Measures of Central Tendency: These describe the "middle" of the data. Common measures include the mean (average), median (middle value), and mode (most frequent value).
- Measures of Dispersion: These describe the spread or variability of the data. Key measures include the range (difference between the highest and lowest values), variance, and standard deviation (measures of how far the data points are from the mean).
- Frequency Distribution: This shows how often each value (or range of values) appears in the data set. It can be represented graphically using histograms, bar charts, or frequency polygons.
Visualizing Univariate Data
Visualizations are key to understanding univariate data. Common visual representations include:
- Histograms: Show the frequency distribution of a continuous variable.
- Bar Charts: Display the frequency distribution of a categorical variable.
- Pie Charts: Illustrate the proportion of each category within the whole.
- Box Plots: Show the distribution of data, including median, quartiles, and outliers.
- Stem-and-Leaf Plots: A simple way to display the distribution of numerical data, particularly useful for smaller datasets.
What is Bivariate Data?
Bivariate data, in contrast to univariate data, involves two variables. The analysis focuses on understanding the relationship between these two variables. We're no longer just looking at a single characteristic, but how two characteristics relate to each other. This relationship can be explored to identify correlations, patterns, and dependencies.
Examples of Bivariate Data:
- Height and weight of students: We're now considering two variables for each student.
- Temperature and ice cream sales: This explores the relationship between daily temperature and the number of ice cream cones sold.
- Age and income: Examining the relationship between the age of individuals and their annual income.
- Hours studied and exam score: This dataset links the number of study hours to the score obtained in an exam.
- Advertising spend and sales revenue: This investigates the correlation between the amount spent on advertising and the resulting sales revenue.
Analyzing Bivariate Data
Analyzing bivariate data involves exploring the relationship between the two variables. This often involves:
- Scatter Plots: These graphically display the relationship between two continuous variables. The pattern of points on the scatter plot can reveal positive, negative, or no correlation.
- Correlation Coefficient: A numerical measure (typically denoted as 'r') that quantifies the strength and direction of the linear relationship between two variables. A correlation coefficient of +1 indicates a perfect positive correlation, -1 indicates a perfect negative correlation, and 0 indicates no linear correlation. It's crucial to remember that correlation does not imply causation.
- Regression Analysis: This statistical technique allows us to model the relationship between two variables, predicting the value of one variable based on the value of the other. Linear regression is commonly used for modeling linear relationships, while other regression techniques exist for non-linear relationships.
- Contingency Tables: Used to analyze the relationship between two categorical variables. They show the frequency distribution of the variables and how they interact with each other.
Visualizing Bivariate Data
Visualizations are critical for understanding bivariate data. The most common visualization is the scatter plot, which effectively shows the relationship between two continuous variables. Other techniques include:
- Line graphs: Particularly useful when one variable represents time.
- Bar charts with grouped bars: Useful for comparing two categorical variables.
- Heatmaps: Can be used to visualize bivariate data, especially when dealing with large datasets.
Key Differences Between Univariate and Bivariate Data
Feature | Univariate Data | Bivariate Data |
---|---|---|
Number of Variables | One | Two |
Focus of Analysis | Distribution, central tendency, dispersion | Relationship between two variables |
Analytical Methods | Descriptive statistics, frequency distributions | Correlation, regression, contingency tables |
Visualizations | Histograms, bar charts, pie charts, box plots | Scatter plots, line graphs, grouped bar charts |
Data Type | Can be continuous or categorical | Can involve both continuous and categorical data |
Objective | Describe the characteristics of a single variable | Explore the relationship between two variables |
Practical Applications
Understanding the distinction between univariate and bivariate data is vital across numerous fields.
Univariate Data Applications:
- Market Research: Analyzing customer satisfaction scores to understand overall customer sentiment.
- Healthcare: Studying the distribution of patient ages to determine the age demographics served by a hospital.
- Finance: Analyzing the daily fluctuations in stock prices to understand market trends.
- Education: Evaluating the distribution of student grades on a specific test to assess overall class performance.
Bivariate Data Applications:
- Marketing: Analyzing the relationship between advertising spend and sales to optimize marketing campaigns.
- Healthcare: Studying the relationship between lifestyle factors (e.g., smoking) and the risk of developing certain diseases.
- Finance: Analyzing the relationship between interest rates and inflation to understand macroeconomic trends.
- Environmental Science: Studying the relationship between carbon dioxide levels and global temperatures to understand climate change.
- Social Sciences: Exploring correlations between socioeconomic status and educational attainment.
Advanced Concepts and Considerations
While this article provides a foundational understanding, the analysis of univariate and bivariate data can become significantly more complex. Here are some advanced aspects:
- Multivariate Data: Extends the concept of bivariate data to include three or more variables. Analyzing multivariate data involves more sophisticated techniques like multiple regression and factor analysis.
- Non-linear Relationships: While linear regression is often used to model bivariate relationships, many real-world relationships are non-linear. Techniques like polynomial regression and non-parametric methods are needed to model these relationships.
- Causation vs. Correlation: A strong correlation between two variables doesn't necessarily imply a causal relationship. Careful consideration is needed to avoid making incorrect causal inferences.
- Data Cleaning and Preprocessing: Before analyzing data, it's crucial to clean and preprocess it to handle missing values, outliers, and inconsistencies.
Conclusion
The difference between univariate and bivariate data lies in the number of variables being analyzed and the nature of the analysis. Univariate data focuses on describing a single variable, while bivariate data explores the relationship between two variables. Understanding these differences is crucial for effectively analyzing data and drawing meaningful conclusions across various domains. Choosing the right analytical and visualization techniques based on the data type and research question is vital for obtaining accurate and insightful results. By mastering these fundamental concepts, you'll be well-equipped to unlock the power of data and make informed decisions based on evidence.
Latest Posts
Related Post
Thank you for visiting our website which covers about What Is The Difference Between Univariate Data And Bivariate Data . We hope the information provided has been useful to you. Feel free to contact us if you have any questions or need further assistance. See you next time and don't miss to bookmark.