What Challenges Does Generative Face With Respect To Data
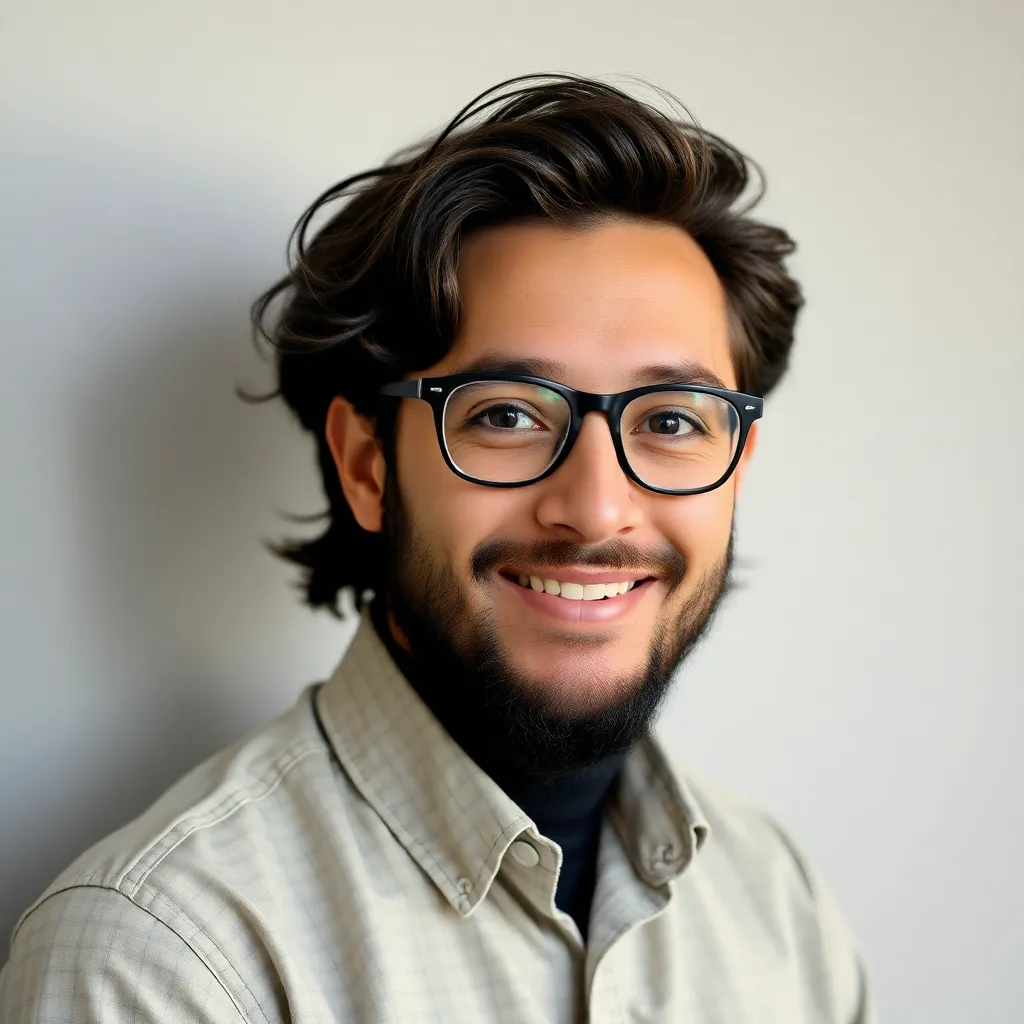
Holbox
Mar 26, 2025 · 6 min read
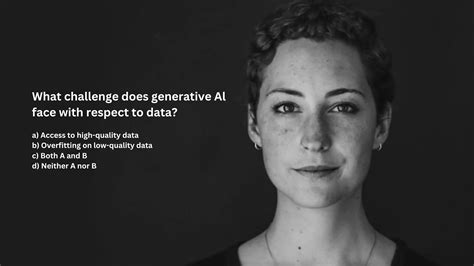
Table of Contents
- What Challenges Does Generative Face With Respect To Data
- Table of Contents
- What Challenges Does Generative AI Face With Respect to Data?
- The Data Hunger of Generative AI Models
- 1. Data Acquisition and Availability:
- 2. Data Quality and Cleaning:
- Ethical and Societal Challenges Related to Data
- 1. Copyright and Intellectual Property:
- 2. Privacy and Data Security:
- 3. Bias and Discrimination:
- Mitigating the Data Challenges in Generative AI
- 1. Data Augmentation and Synthesis:
- 2. Improved Data Cleaning and Pre-processing Techniques:
- 3. Ethical Data Governance and Responsible AI Practices:
- 4. Collaboration and Data Sharing:
- Conclusion: Navigating the Complex Landscape of Data in Generative AI
- Latest Posts
- Latest Posts
- Related Post
What Challenges Does Generative AI Face With Respect to Data?
Generative AI, with its capacity to produce novel content ranging from text and images to code and music, is rapidly transforming numerous industries. However, this transformative power is inextricably linked to the data it's trained on. The challenges generative AI faces with respect to data are multifaceted and significant, impacting not only the quality and reliability of its output but also its ethical implications and broader societal impact. This article delves deep into these challenges, exploring their nuances and potential solutions.
The Data Hunger of Generative AI Models
Generative AI models, particularly large language models (LLMs) and diffusion models, are notoriously data-hungry. They require massive datasets to learn the intricate patterns and relationships within the data, enabling them to generate coherent and relevant outputs. This insatiable appetite for data presents several key challenges:
1. Data Acquisition and Availability:
- Scale: Obtaining datasets of sufficient size and diversity is a major hurdle. The sheer volume of data needed to train state-of-the-art models can be overwhelming, requiring significant computational resources and infrastructure.
- Accessibility: Many valuable datasets are not publicly accessible, either due to proprietary restrictions or privacy concerns. This limits the ability of researchers and developers to train and improve their models.
- Data Bias: The availability of data often reflects existing societal biases, leading to skewed datasets that can perpetuate and amplify harmful stereotypes in the generated content. This is a particularly acute problem when dealing with sensitive topics like gender, race, and ethnicity.
2. Data Quality and Cleaning:
- Noise and Inconsistency: Real-world datasets are rarely clean and consistent. They often contain errors, inconsistencies, and irrelevant information that can negatively impact model performance. Cleaning and pre-processing this data can be incredibly time-consuming and labor-intensive.
- Data Sparsity: For certain domains or tasks, sufficient high-quality data might be simply unavailable. This lack of data can severely limit the model's ability to generate meaningful outputs in those specific areas.
- Data Representation: The way data is structured and represented can significantly influence model performance. Choosing appropriate data formats and representations is crucial for efficient training and accurate results.
Ethical and Societal Challenges Related to Data
Beyond the technical hurdles, the data used to train generative AI models raises serious ethical and societal concerns:
1. Copyright and Intellectual Property:
- Training Data Ownership: Many training datasets comprise copyrighted material, raising questions about the legality of using this data to train generative models. The potential for infringement on intellectual property rights is a significant concern for both developers and copyright holders.
- Attribution and Licensing: Determining appropriate attribution and licensing for generated content remains a complex issue. The models often blend and transform their input data in unpredictable ways, making it difficult to trace the origins of specific elements.
2. Privacy and Data Security:
- Sensitive Information Leakage: Generative models trained on personal data can inadvertently leak sensitive information, violating privacy and potentially causing harm. This is particularly problematic when dealing with medical records, financial data, or other sensitive personal information.
- Data Breaches: The massive datasets used to train generative AI models represent attractive targets for cyberattacks. Breaches could compromise sensitive information and cause significant damage.
- Data Anonymization Challenges: Effective anonymization techniques are crucial to protect privacy. However, even anonymized data can be re-identified under certain circumstances, highlighting the ongoing challenges in balancing data utility with privacy preservation.
3. Bias and Discrimination:
- Amplification of Existing Biases: As mentioned earlier, biased training data can lead to models that perpetuate and amplify harmful stereotypes, potentially causing significant social harm. This bias can manifest in various ways, including gender bias, racial bias, and other forms of discrimination.
- Lack of Representation: Underrepresentation of certain groups in the training data can lead to models that are less accurate and reliable when generating content related to those underrepresented groups. This can exacerbate existing inequalities and marginalization.
- Unintended Consequences: Even with careful data curation, unintended biases can still emerge during the training process. Identifying and mitigating these biases requires ongoing monitoring and evaluation.
Mitigating the Data Challenges in Generative AI
Addressing these challenges requires a multi-pronged approach involving technical innovations, ethical considerations, and policy interventions:
1. Data Augmentation and Synthesis:
- Synthetic Data Generation: Creating synthetic data that mimics the characteristics of real-world data can help address data scarcity and privacy concerns. This can be achieved using various techniques such as generative adversarial networks (GANs) and variational autoencoders (VAEs).
- Data Augmentation Techniques: Applying data augmentation techniques, such as adding noise, cropping, or rotating images, can increase the size and diversity of training datasets.
2. Improved Data Cleaning and Pre-processing Techniques:
- Automated Data Cleaning Tools: Developing robust and efficient tools for automated data cleaning and pre-processing can significantly reduce the time and effort required for data preparation.
- Improved Data Quality Control: Implementing rigorous data quality control measures throughout the data lifecycle can help minimize errors and inconsistencies.
3. Ethical Data Governance and Responsible AI Practices:
- Data Provenance and Transparency: Tracking the origin and usage of data throughout the AI lifecycle is crucial for ensuring responsible AI practices. Transparency in data sourcing and usage is vital for building trust and accountability.
- Bias Detection and Mitigation Techniques: Developing and implementing effective bias detection and mitigation techniques is essential for preventing models from perpetuating harmful stereotypes.
- Ethical Guidelines and Regulations: Establishing clear ethical guidelines and regulations for the development and deployment of generative AI models can help promote responsible innovation and mitigate potential harms.
4. Collaboration and Data Sharing:
- Open-Source Datasets: Promoting the development and sharing of open-source datasets can encourage collaboration and accelerate research progress. However, careful consideration must be given to data privacy and licensing issues.
- Data Sharing Initiatives: Establishing data sharing initiatives between researchers, developers, and organizations can help overcome the limitations of data scarcity and accessibility.
Conclusion: Navigating the Complex Landscape of Data in Generative AI
The challenges posed by data in generative AI are significant and complex. They require a holistic approach that addresses technical, ethical, and societal concerns. By investing in robust data management techniques, promoting responsible AI practices, and fostering collaboration, we can harness the immense potential of generative AI while mitigating its risks. The future of generative AI hinges on our ability to navigate this complex landscape responsibly, ensuring that these powerful technologies benefit humanity as a whole. Ongoing research, transparent development processes, and robust regulatory frameworks are essential for navigating the ethical and technical complexities of data within this rapidly evolving field. Only through careful consideration and proactive action can we ensure that generative AI realizes its full potential without compromising our values or jeopardizing societal well-being.
Latest Posts
Latest Posts
-
The Unit Sales Of Unmanned Aerial View
Mar 30, 2025
-
In Making Short Term Business Decisions What Should You Do
Mar 30, 2025
-
Select The Medical Term That Means A Type Of Fungus
Mar 30, 2025
-
Dethatching Is A Decreasingly Common Cultural Practice
Mar 30, 2025
-
Which Of The Following Statements About Internal Control Is Correct
Mar 30, 2025
Related Post
Thank you for visiting our website which covers about What Challenges Does Generative Face With Respect To Data . We hope the information provided has been useful to you. Feel free to contact us if you have any questions or need further assistance. See you next time and don't miss to bookmark.