What Challenges Does Generative Ai Face With Respect To Data
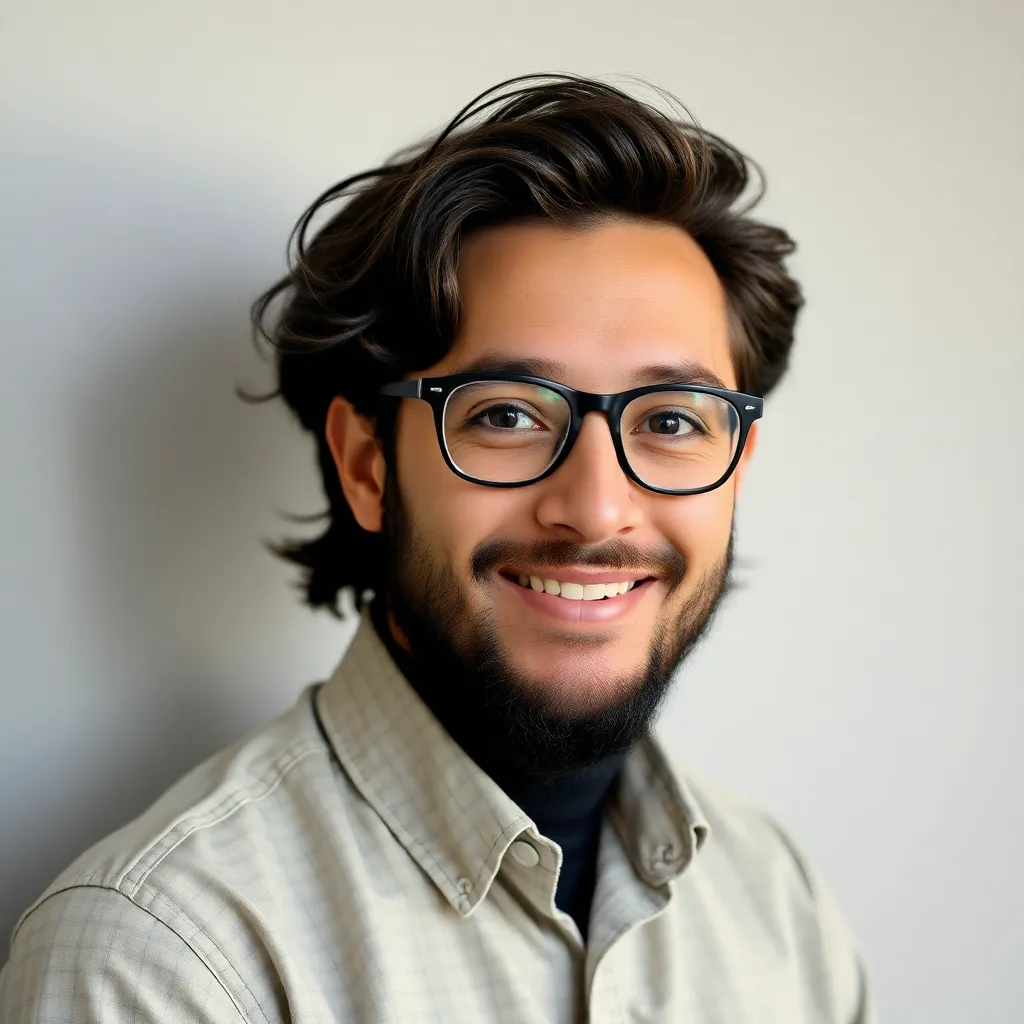
Holbox
Mar 11, 2025 · 5 min read

Table of Contents
- What Challenges Does Generative Ai Face With Respect To Data
- Table of Contents
- What Challenges Does Generative AI Face with Respect to Data?
- The Data Hunger of Generative AI Models
- 1. Data Acquisition and Cost:
- 2. Data Bias and Fairness:
- 3. Data Scarcity in Specific Domains:
- Data Quality and Representation:
- 4. Data Noise and Inconsistency:
- 5. Data Imbalance and Class Distribution:
- 6. Data Representation and Generalization:
- Ethical and Legal Considerations:
- 7. Copyright and Intellectual Property:
- 8. Privacy and Data Security:
- 9. Transparency and Explainability:
- 10. Misinformation and Malicious Use:
- Mitigating the Challenges:
- Conclusion:
- Latest Posts
- Related Post
What Challenges Does Generative AI Face with Respect to Data?
Generative AI, with its capacity to create novel content ranging from text and images to music and code, is rapidly transforming numerous industries. However, this transformative power is inextricably linked to the data it's trained on. The challenges generative AI faces with respect to data are multifaceted and significant, impacting its reliability, ethical implications, and overall potential. This article delves deep into these challenges, exploring their complexities and potential solutions.
The Data Hunger of Generative AI Models
Generative AI models, particularly large language models (LLMs) and generative adversarial networks (GANs), are notorious for their insatiable appetite for data. Training these models requires massive datasets, often terabytes or even petabytes in size. This data hunger presents several key challenges:
1. Data Acquisition and Cost:
Acquiring such vast datasets is a significant undertaking. It often involves:
- Web scraping: Gathering data from the internet is a common practice, but it raises legal and ethical concerns related to copyright infringement and privacy violations. Ensuring data legality and ethical sourcing is crucial but complex.
- Data licensing: Purchasing licensed datasets can be prohibitively expensive, especially for smaller organizations or researchers. The cost can quickly escalate with the size and quality of the required data.
- Data cleaning and preprocessing: Raw data is rarely usable directly. It often requires extensive cleaning, preprocessing, and formatting to eliminate errors, inconsistencies, and biases before it can be used to train a model. This process is time-consuming and labor-intensive.
2. Data Bias and Fairness:
One of the most pressing challenges is data bias. The data used to train generative AI models often reflects existing societal biases, prejudices, and stereotypes present in the source material. This can lead to AI systems that perpetuate and even amplify these biases, resulting in unfair or discriminatory outputs. For instance, a language model trained on a dataset with gender-biased language might generate text that reinforces harmful stereotypes about gender roles. Addressing data bias requires careful curation and preprocessing, but complete elimination remains a significant hurdle.
3. Data Scarcity in Specific Domains:
While data abundance is a challenge in general, a lack of sufficient data is a separate problem for specific domains. Training effective generative AI models for niche applications often faces the hurdle of insufficient relevant data. This is particularly true for specialized fields with limited publicly available information or where data collection is difficult or expensive. For example, creating a generative AI model for diagnosing rare diseases might be hampered by the limited availability of medical images and patient data.
Data Quality and Representation:
Beyond sheer quantity, the quality and representational aspects of data profoundly impact generative AI performance.
4. Data Noise and Inconsistency:
Real-world data is inherently messy. It contains noise, errors, inconsistencies, and inaccuracies. This noisy data can negatively affect the training process, leading to unreliable and inaccurate models. Robust data cleaning and preprocessing techniques are crucial to mitigate the impact of noise, but completely eliminating it is rarely feasible.
5. Data Imbalance and Class Distribution:
Data imbalance, where certain classes or categories are significantly underrepresented compared to others, is a common problem. This can lead to biased models that perform poorly on the underrepresented classes. For example, a facial recognition system trained on a dataset with predominantly light-skinned individuals might perform poorly on darker-skinned individuals. Addressing data imbalance often requires techniques such as oversampling, undersampling, or data augmentation.
6. Data Representation and Generalization:
The way data is represented and structured significantly impacts a model's ability to generalize to new, unseen data. Poor data representation can limit a model's capacity to learn meaningful patterns and relationships, hindering its performance on unseen examples. Careful feature engineering and selection are crucial for ensuring adequate data representation.
Ethical and Legal Considerations:
The data used to train generative AI models raises numerous ethical and legal concerns:
7. Copyright and Intellectual Property:
Using copyrighted material to train generative AI models raises legal questions about copyright infringement. Determining the fair use of copyrighted data for training purposes is a complex and ongoing legal debate. The lines become especially blurred with the creation of derivative works.
8. Privacy and Data Security:
Many datasets used for training generative AI models contain sensitive personal information. Protecting the privacy and security of this data is paramount. Techniques like data anonymization and differential privacy are crucial for mitigating privacy risks, but they are not foolproof and can impact the model's performance.
9. Transparency and Explainability:
The lack of transparency in how generative AI models are trained and what data they utilize raises concerns about accountability and responsibility. Understanding how a model arrives at a particular output is crucial for building trust and ensuring fairness. However, many generative AI models are "black boxes," making it difficult to understand their decision-making processes.
10. Misinformation and Malicious Use:
Generative AI models can be used to create highly realistic but false content, including fake news, deepfakes, and other forms of misinformation. This poses a significant threat to society, potentially undermining trust in information sources and exacerbating social divisions.
Mitigating the Challenges:
Addressing the challenges posed by data in generative AI requires a multi-pronged approach:
- Developing robust data cleaning and preprocessing techniques: Improving algorithms and methods for handling noisy, inconsistent, and biased data is crucial.
- Creating larger, more representative datasets: Investing in data collection and curation efforts, particularly for underrepresented groups and domains, is essential. This also includes incentivizing data sharing and collaboration.
- Developing more robust methods for detecting and mitigating bias: Researchers are actively developing techniques to identify and reduce bias in training data and model outputs.
- Improving data privacy and security measures: Developing and implementing more sophisticated privacy-preserving techniques is necessary to protect sensitive personal information.
- Promoting transparency and explainability in AI models: Researchers are working on making AI models more interpretable and understandable, allowing for better accountability and trust.
- Establishing ethical guidelines and regulations: Clear guidelines and regulations are needed to govern the use of data in generative AI, addressing issues like copyright, privacy, and responsible use.
Conclusion:
The challenges generative AI faces with respect to data are significant but not insurmountable. By addressing these challenges through technological advancements, ethical considerations, and robust regulatory frameworks, we can harness the immense potential of generative AI while mitigating its risks. The future of generative AI hinges on our ability to responsibly manage and utilize the data that fuels its power. Continued research and collaboration across disciplines are vital to navigate this complex landscape and ensure that generative AI benefits society as a whole.
Latest Posts
Related Post
Thank you for visiting our website which covers about What Challenges Does Generative Ai Face With Respect To Data . We hope the information provided has been useful to you. Feel free to contact us if you have any questions or need further assistance. See you next time and don't miss to bookmark.