What Best Accounts For The Observation
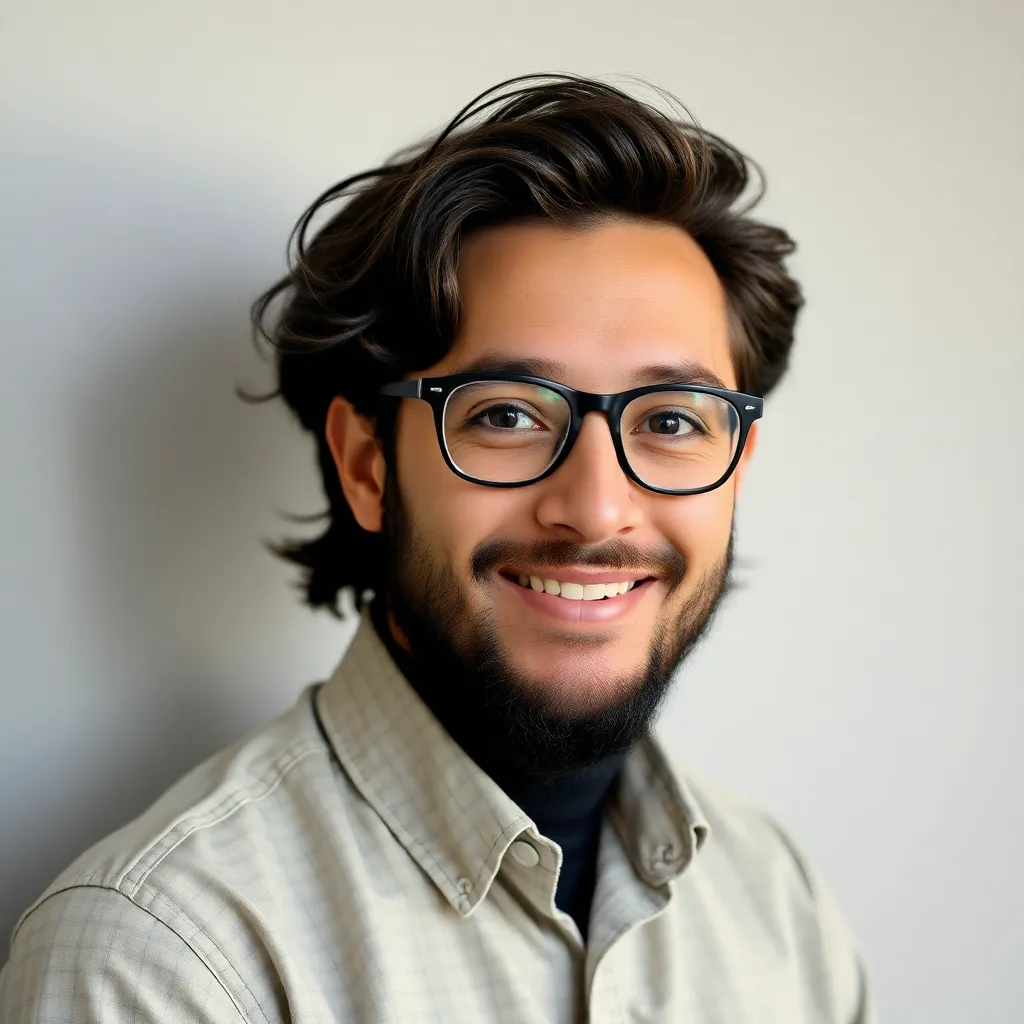
Holbox
Apr 05, 2025 · 6 min read

Table of Contents
- What Best Accounts For The Observation
- Table of Contents
- What Best Accounts for the Observation? A Deep Dive into Causal Inference
- Understanding Correlation vs. Causation
- The Role of Controlled Experiments
- The Challenges of Observational Studies
- The Importance of Mechanistic Understanding
- Addressing Alternative Explanations
- The Role of Temporal Precedence
- Bayesian Reasoning and Prior Knowledge
- The Limitations of Statistical Significance
- Case Studies: Illustrating the Challenges
- Conclusion: The Ongoing Pursuit of Causal Understanding
- Latest Posts
- Latest Posts
- Related Post
What Best Accounts for the Observation? A Deep Dive into Causal Inference
The question, "What best accounts for the observation?" lies at the heart of scientific inquiry, data analysis, and critical thinking. It's a question that demands rigorous investigation, careful consideration of alternative explanations, and a robust understanding of causal inference. This article delves into the various methods and considerations involved in answering this crucial question, exploring the nuances of correlation versus causation, the importance of controlled experiments, and the limitations of observational studies.
Understanding Correlation vs. Causation
The first crucial step in determining what best accounts for an observation is to differentiate between correlation and causation. Simply because two things happen together doesn't mean one causes the other. Correlation refers to a statistical relationship between two variables—when one changes, the other tends to change as well. However, causation implies that one variable directly influences another; a change in one variable causes a change in the other.
For example, ice cream sales and crime rates might be positively correlated: both tend to increase during the summer months. However, this doesn't mean that eating ice cream causes crime, or vice versa. The underlying cause is likely a third factor—the warmer weather—that influences both ice cream sales and crime rates independently. This third factor is known as a confounder and highlights the importance of carefully considering all potential influences when investigating causal relationships.
The Role of Controlled Experiments
The gold standard for establishing causality is the controlled experiment. In a controlled experiment, researchers manipulate one variable (the independent variable) and measure its effect on another variable (the dependent variable), while holding all other factors constant. This control allows researchers to isolate the effect of the independent variable and determine whether it truly causes a change in the dependent variable.
Randomized controlled trials (RCTs) are a particularly powerful type of controlled experiment. In RCTs, participants are randomly assigned to either an experimental group (which receives the treatment or intervention) or a control group (which does not). This randomization helps ensure that the groups are comparable at the outset, minimizing the influence of confounding variables. The results of a well-designed RCT provide strong evidence of a causal relationship.
The Challenges of Observational Studies
Often, conducting a controlled experiment is impractical or unethical. In such cases, researchers rely on observational studies, which involve observing and measuring variables without manipulating them. Observational studies can provide valuable insights, but they are inherently more susceptible to confounding variables and biases, making it more challenging to establish causality.
Several techniques can help mitigate these challenges in observational studies:
- Matching: Researchers can match individuals in the study based on relevant characteristics to create more comparable groups. This reduces the impact of confounding variables.
- Stratification: Researchers can analyze data within subgroups (strata) defined by different levels of confounding variables. This helps to control for the influence of these variables.
- Regression analysis: Statistical techniques like regression analysis can help to adjust for the effects of confounding variables, allowing researchers to estimate the independent effect of the variable of interest.
Despite these methods, observational studies can never definitively prove causation. Even with careful analysis, the possibility of unmeasured or unknown confounders remains. Therefore, the conclusions drawn from observational studies should be interpreted with caution.
The Importance of Mechanistic Understanding
Beyond statistical analysis, a strong understanding of the underlying mechanisms is crucial for establishing causality. Simply showing a statistical association isn't enough; researchers need to understand how the proposed cause influences the effect. This involves identifying the biological, physical, or social processes that link the cause and effect.
For example, if a study shows a correlation between smoking and lung cancer, a mechanistic understanding would involve explaining how the chemicals in cigarette smoke damage lung tissue and lead to the development of cancer. This mechanistic explanation strengthens the causal claim significantly.
Addressing Alternative Explanations
A critical aspect of determining what best accounts for an observation involves systematically considering and ruling out alternative explanations. This involves:
- Identifying potential confounders: Carefully considering all variables that could potentially influence both the proposed cause and effect.
- Testing alternative hypotheses: Developing and testing hypotheses that propose different causal mechanisms.
- Evaluating the plausibility of each explanation: Considering the biological, physical, or social plausibility of each proposed explanation.
The Role of Temporal Precedence
Another key element in establishing causality is temporal precedence: the cause must precede the effect in time. If the purported effect occurs before the purported cause, a causal relationship is impossible. This seemingly obvious point is often overlooked, particularly in observational studies where determining the precise temporal sequence of events can be challenging.
Bayesian Reasoning and Prior Knowledge
Bayesian reasoning provides a formal framework for updating beliefs based on new evidence. Prior knowledge about the plausibility of different causal mechanisms can significantly influence the interpretation of data. Strong prior knowledge, combined with compelling evidence, can lead to stronger conclusions about causality. However, it is important to acknowledge any biases in prior beliefs and avoid letting them unduly influence the interpretation of data.
The Limitations of Statistical Significance
While statistical significance (typically represented by a p-value) is often used to assess the strength of evidence, it's crucial to avoid placing too much emphasis on it. A statistically significant result doesn't automatically imply causality, especially in the presence of potential confounders or weak mechanistic understanding. Furthermore, a statistically non-significant result doesn't necessarily mean a lack of a causal relationship – it might simply reflect a lack of power to detect a real effect.
Case Studies: Illustrating the Challenges
Let's consider a few illustrative examples to solidify these concepts:
Example 1: Coffee Consumption and Heart Disease: Observational studies have shown a correlation between coffee consumption and a reduced risk of heart disease. However, it's impossible to definitively conclude that coffee causes this reduction without controlling for other factors, such as diet, exercise, and socioeconomic status, which might influence both coffee consumption and heart health. A potential confounder could be that people who drink coffee are also more likely to engage in healthy lifestyle choices.
Example 2: Vaccine Safety: Observational studies linking vaccines to adverse events are often prone to bias and confounding. For example, a temporal association between a vaccine and an illness doesn't necessarily mean the vaccine caused the illness. The illness might have been coincidental or caused by other factors. RCTs are crucial for determining the true causal relationship between vaccines and adverse events.
Example 3: Social Media Use and Depression: Observational studies have shown correlations between social media use and depression. However, determining causality is difficult. It's possible that social media use contributes to depression, but it's also possible that depressed individuals are more likely to use social media as a coping mechanism or that other factors, such as social isolation or pre-existing mental health conditions, influence both social media use and depression.
Conclusion: The Ongoing Pursuit of Causal Understanding
Determining what best accounts for an observation is a complex and multifaceted process. While controlled experiments offer the strongest evidence of causality, they are not always feasible. Observational studies, while providing valuable insights, are susceptible to bias and confounding. To draw robust conclusions, researchers must combine rigorous statistical analysis with a deep understanding of the underlying mechanisms, careful consideration of alternative explanations, and an awareness of the limitations of different research designs. The ongoing pursuit of causal understanding requires a combination of critical thinking, innovative research methodologies, and a healthy dose of skepticism. Only through such a holistic approach can we move beyond mere correlation and gain a clearer understanding of the causal relationships that shape our world.
Latest Posts
Latest Posts
-
Which Of The Following Statements Are True Regarding Corporations
Apr 09, 2025
-
Businesses Must Monitor The Factors In Their Environment To
Apr 09, 2025
-
Is Garlic Powder A Pure Substance Or Mixture
Apr 09, 2025
-
You Ring Up An Item For A Customer
Apr 09, 2025
-
Save The Current Workbook To The Workshops Folder
Apr 09, 2025
Related Post
Thank you for visiting our website which covers about What Best Accounts For The Observation . We hope the information provided has been useful to you. Feel free to contact us if you have any questions or need further assistance. See you next time and don't miss to bookmark.