Using Logic To Compare Samples With Different Sources Of Variation
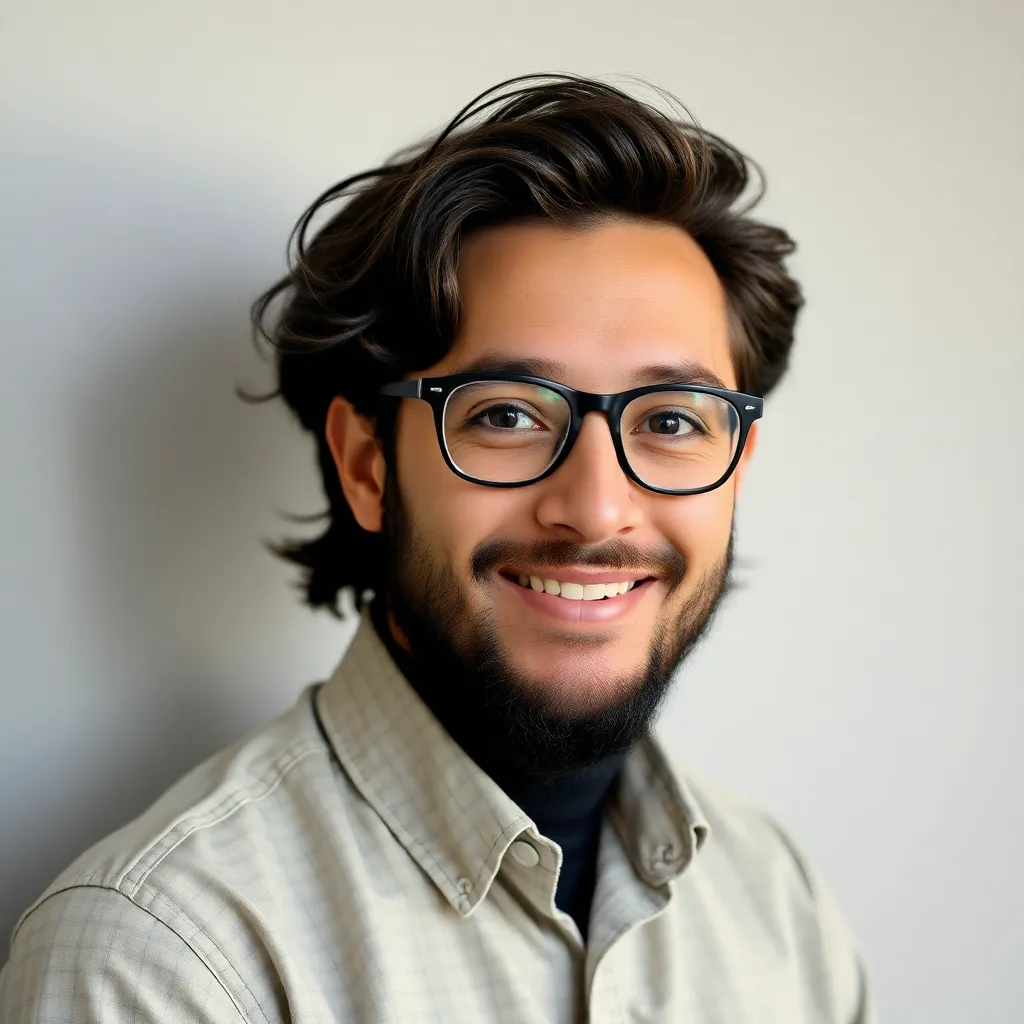
Holbox
May 08, 2025 · 6 min read
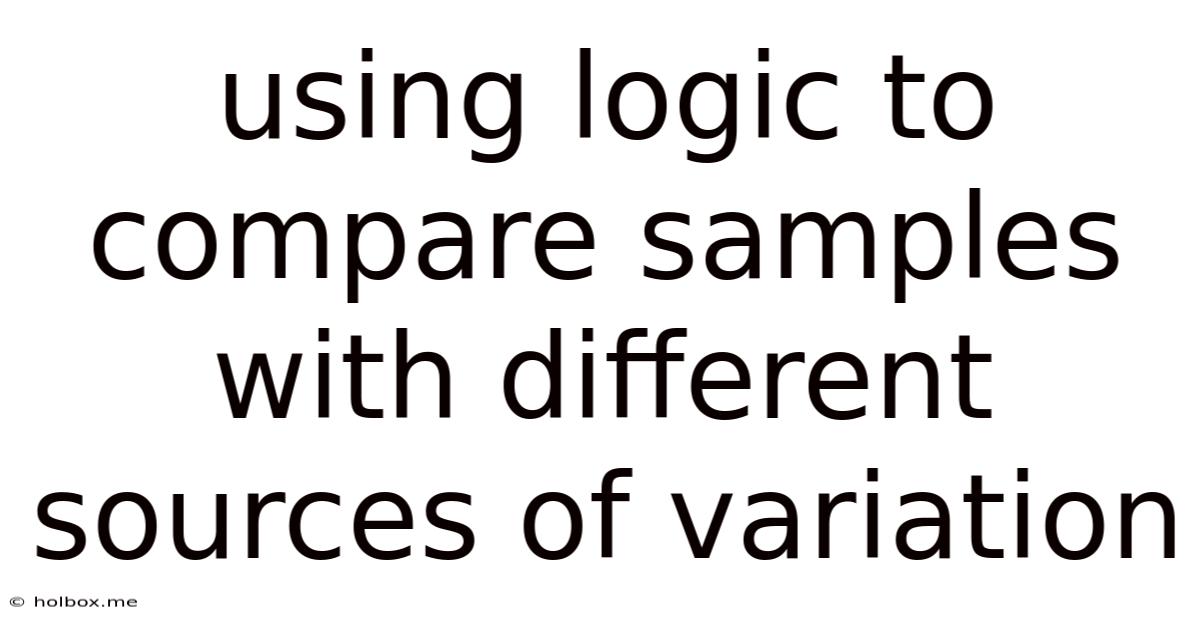
Table of Contents
- Using Logic To Compare Samples With Different Sources Of Variation
- Table of Contents
- Using Logic to Compare Samples with Different Sources of Variation
- Understanding Sources of Variation
- 1. Random Variation (Error):
- 2. Systematic Variation:
- 3. Interaction Effects:
- Statistical Techniques for Comparing Samples with Different Sources of Variation
- 1. Analysis of Variance (ANOVA):
- 2. Analysis of Covariance (ANCOVA):
- 3. Regression Analysis:
- 4. Mixed-effects Models:
- 5. Non-parametric Methods:
- Logical Considerations and Interpretation of Results
- Example: Comparing Crop Yields Across Different Regions
- Conclusion
- Latest Posts
- Related Post
Using Logic to Compare Samples with Different Sources of Variation
Comparing samples from different sources, each with its own unique variations, presents a significant challenge in many fields, from scientific research to business analytics. Simply comparing averages or raw data can be misleading and fail to reveal meaningful insights. This article delves into the logical frameworks and statistical techniques crucial for effectively comparing such samples, highlighting the importance of accounting for different sources of variation. We'll explore how to identify, isolate, and interpret these variations to reach reliable conclusions.
Understanding Sources of Variation
Before comparing samples, it's crucial to identify the potential sources of variation within and between them. These variations can be broadly categorized as:
1. Random Variation (Error):
This is inherent in any measurement process. It represents the unpredictable fluctuations that arise from numerous minor, uncontrolled factors. Random variation is typically assumed to be normally distributed, meaning it follows a bell curve. While we can't eliminate random variation entirely, we can minimize its impact through careful experimental design and appropriate statistical analysis.
2. Systematic Variation:
This type of variation results from known or identifiable factors that consistently influence the measurements. For example, different batches of materials used in a manufacturing process, different experimental conditions, or differences in the skill level of the individuals collecting the data, can all contribute to systematic variation. Understanding and accounting for these systematic variations is critical for making valid comparisons.
3. Interaction Effects:
This often overlooked source arises when the effect of one factor depends on the level of another factor. For example, the effect of fertilizer type on plant growth might vary depending on the soil type. Ignoring interaction effects can lead to incorrect conclusions.
Statistical Techniques for Comparing Samples with Different Sources of Variation
Several statistical methods are designed to address the challenges posed by multiple sources of variation. The appropriate technique depends on the specific research question, the nature of the data, and the number of variables involved.
1. Analysis of Variance (ANOVA):
ANOVA is a powerful technique for comparing the means of three or more groups. It partitions the total variation in the data into different sources of variation, allowing us to determine whether the differences between group means are statistically significant or simply due to random variation. ANOVA is particularly useful when dealing with systematic variation arising from different treatment groups or experimental conditions.
One-way ANOVA: Used when there is only one factor influencing the variation.
Two-way ANOVA: Used when there are two factors influencing the variation, allowing for the investigation of main effects and interaction effects.
Repeated measures ANOVA: Used when the same subjects are measured multiple times under different conditions.
2. Analysis of Covariance (ANCOVA):
ANCOVA extends ANOVA by incorporating one or more continuous covariates. This is particularly useful when we want to control for the influence of a confounding variable that might affect the dependent variable. For instance, when comparing plant growth under different fertilizer treatments, ANCOVA can control for initial plant size. By adjusting for the covariate, we can obtain a more accurate assessment of the fertilizer's effect.
3. Regression Analysis:
Regression analysis models the relationship between a dependent variable and one or more independent variables. It can be used to identify and quantify the impact of different factors on the outcome. This is especially valuable when dealing with continuous data and multiple sources of variation. For example, we could use regression to model the relationship between crop yield (dependent variable) and rainfall, fertilizer type (independent variables).
4. Mixed-effects Models:
These models are particularly well-suited for data with nested or hierarchical structures, where observations are clustered within groups. For instance, if we're comparing student performance across different schools, the students are nested within schools. Mixed-effects models account for the correlation between observations within the same group, providing more accurate estimates of the effects of interest. They handle random variation at multiple levels effectively.
5. Non-parametric Methods:
When assumptions of normality or equal variances are violated, non-parametric methods provide robust alternatives to ANOVA or t-tests. These methods don't rely on specific distributional assumptions, making them useful when dealing with skewed data or outliers. Examples include the Kruskal-Wallis test (analogous to one-way ANOVA) and the Friedman test (for repeated measures).
Logical Considerations and Interpretation of Results
The statistical analysis itself is only part of the process. Careful logical reasoning is essential throughout:
-
Clearly Define the Research Question: What are you trying to compare? What are the specific sources of variation you're interested in? A well-defined question guides the entire analysis.
-
Appropriate Sample Size: Ensure sufficient sample size for each group to obtain reliable results. Power analysis can help determine the necessary sample size to detect meaningful differences.
-
Data Cleaning and Preprocessing: Examine your data for outliers, missing values, and errors. Appropriate handling of these issues is crucial for accurate analysis.
-
Visualizations: Graphs and charts (box plots, scatter plots) are invaluable tools for visualizing the data and identifying patterns and potential issues.
-
Interpreting p-values: A statistically significant p-value (typically below 0.05) indicates that the observed differences are unlikely to be due to random chance. However, statistical significance doesn't necessarily imply practical significance. Consider the effect size and the context of the results.
-
Addressing Limitations: Acknowledge any limitations of the study design or analysis. This enhances the credibility of your conclusions.
Example: Comparing Crop Yields Across Different Regions
Imagine a study comparing crop yields across three different regions, each characterized by distinct soil types and rainfall patterns. Here, the sources of variation include:
- Regional Variation: Systematic differences in soil type and rainfall between regions.
- Random Variation: Unpredictable fluctuations due to weather events, pests, etc.
- Interaction Effect: The interaction between soil type and rainfall might influence crop yield differently in each region.
To analyze this data, we might use a two-way ANOVA, with region and soil type as factors. This would allow us to assess the main effects of region and soil type, as well as their interaction effect on crop yield. Visualizations, such as box plots, would help compare the distributions of yields across regions and soil types. The results would be interpreted considering the specific context of each region's environmental conditions.
Conclusion
Comparing samples with different sources of variation necessitates a robust, multi-faceted approach. The process begins with carefully identifying potential sources of variation and proceeds with employing appropriate statistical techniques such as ANOVA, ANCOVA, regression, or mixed-effects models, depending on the nature of the data and the research question. Critically, strong logical reasoning throughout the process, from experimental design to interpretation of results, is indispensable. By thoughtfully combining statistical methods with logical considerations, we can effectively navigate the complexities of comparing samples and uncover meaningful insights from data exhibiting diverse sources of variation. Remember to always consider the limitations of your methods and present your findings transparently to ensure the robustness and credibility of your conclusions. The combination of sound statistical methodology and logical interpretation is crucial for accurate and meaningful results in any comparative analysis.
Latest Posts
Related Post
Thank you for visiting our website which covers about Using Logic To Compare Samples With Different Sources Of Variation . We hope the information provided has been useful to you. Feel free to contact us if you have any questions or need further assistance. See you next time and don't miss to bookmark.