Use The Graph To Estimate The Dissociation Constant
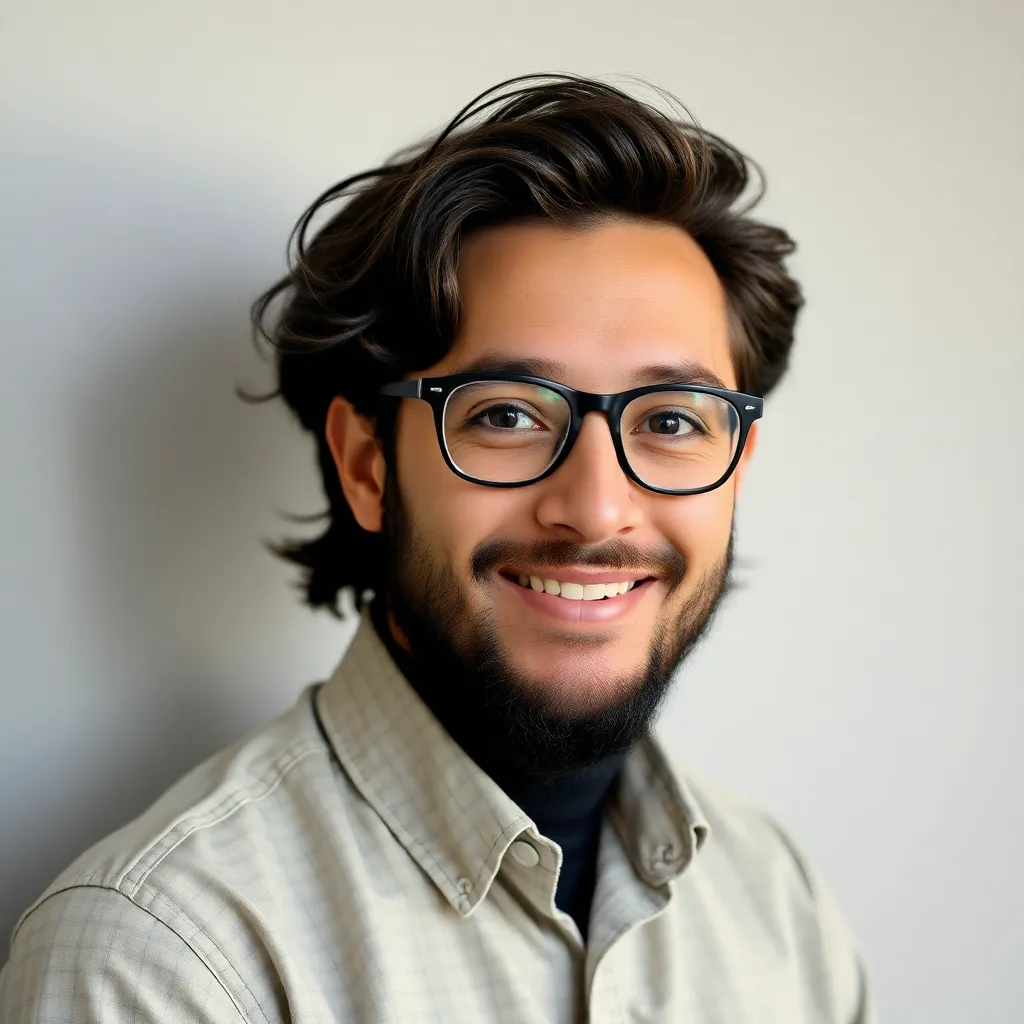
Holbox
May 09, 2025 · 6 min read
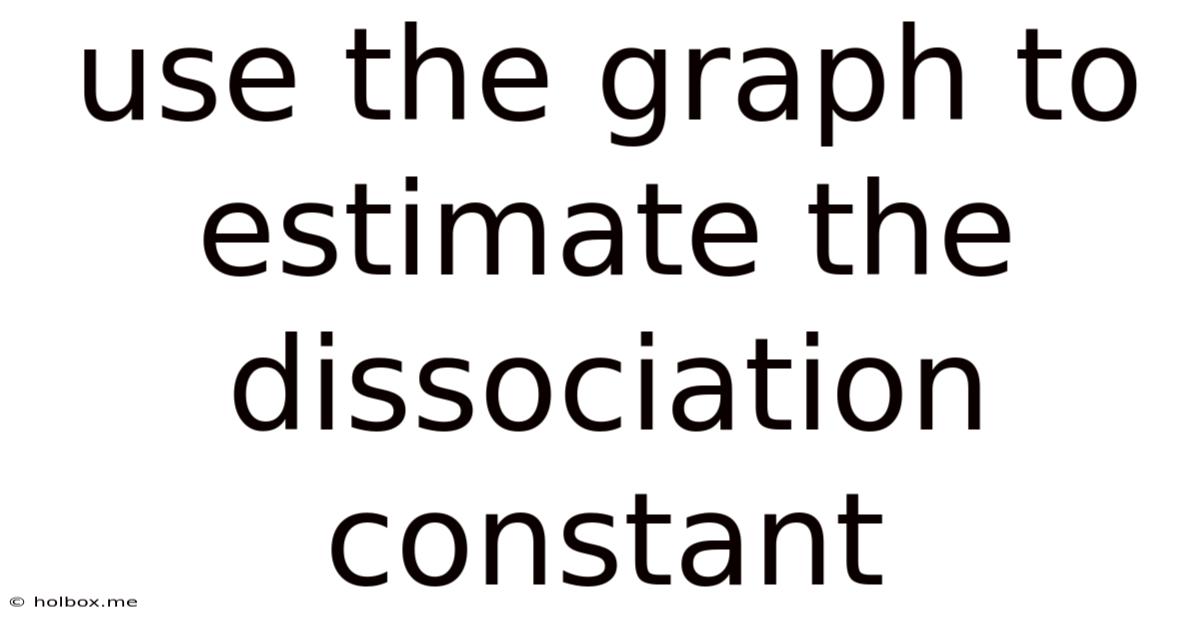
Table of Contents
- Use The Graph To Estimate The Dissociation Constant
- Table of Contents
- Using Graphs to Estimate the Dissociation Constant (Kd)
- Understanding the Dissociation Constant (Kd)
- Graphical Methods for Estimating Kd
- 1. Direct Binding Plot (Saturation Binding Curve)
- 2. Scatchard Plot
- 3. Lineweaver-Burk Plot (Double Reciprocal Plot)
- 4. Hanes-Woolf Plot
- Choosing the Right Graphical Method
- Importance of Non-Linear Regression
- Practical Considerations and Limitations
- Conclusion
- Latest Posts
- Latest Posts
- Related Post
Using Graphs to Estimate the Dissociation Constant (Kd)
Determining the dissociation constant (Kd) is crucial in various fields, including biochemistry, pharmacology, and environmental science. Kd represents the equilibrium constant for the dissociation of a complex into its constituent components. While precise Kd values often require sophisticated techniques, a graphical approach using experimental data offers a valuable, accessible estimate. This article explores several graphical methods for estimating Kd, highlighting their strengths, limitations, and practical applications.
Understanding the Dissociation Constant (Kd)
Before delving into graphical estimations, let's solidify our understanding of Kd. For a reversible binding reaction, such as the interaction between a ligand (L) and a receptor (R):
R + L ⇌ RL
The dissociation constant (Kd) is defined as:
Kd = [R][L] / [RL]
where [R], [L], and [RL] represent the equilibrium concentrations of the free receptor, free ligand, and the receptor-ligand complex, respectively. A lower Kd value indicates higher affinity, meaning the complex is more stable and less likely to dissociate. Conversely, a higher Kd value signifies lower affinity.
Graphical Methods for Estimating Kd
Several graphical representations allow for the estimation of Kd from experimental data. The choice of method often depends on the type of data collected and the experimental setup.
1. Direct Binding Plot (Saturation Binding Curve)
This is the most straightforward method. It involves plotting the amount of bound ligand ([RL]) against the concentration of free ligand ([L]). The resulting curve is a rectangular hyperbola. While visually intuitive, extracting Kd directly from this curve is not trivial.
-
Procedure: Experimental data typically involves measuring the amount of bound ligand at different free ligand concentrations. These data points are plotted.
-
Estimation of Kd: The Kd is approximately half the concentration of free ligand ([L]) at which half of the receptor sites are occupied (i.e., half-maximal binding). This point is often referred to as the half-saturation point. Accurate determination requires fitting the data to a non-linear regression model, such as the Hill equation, to obtain a precise Kd value.
-
Strengths: Simple concept, visually clear representation of binding affinity.
-
Weaknesses: Accurate Kd determination necessitates curve fitting, which can be complex, and prone to error if the data is noisy or the binding isn't strictly following a simple 1:1 model.
2. Scatchard Plot
The Scatchard plot linearizes the binding data, simplifying Kd estimation. However, it's sensitive to experimental error, particularly at high ligand concentrations.
-
Procedure: The Scatchard plot is generated by plotting the ratio of bound ligand to free ligand ([RL]/[L]) against the concentration of bound ligand ([RL]).
-
Estimation of Kd: The plot produces a straight line with a slope of -1/Kd and an x-intercept representing the total number of binding sites ([Rtotal]). The negative reciprocal of the slope provides an estimate for Kd.
-
Strengths: Linear relationship simplifies estimation, provides an estimate of the total number of binding sites.
-
Weaknesses: Highly sensitive to experimental error, particularly at high and low [RL]/[L] values. Assumes a single class of binding sites and non-cooperative binding. Outliers can significantly skew the results.
3. Lineweaver-Burk Plot (Double Reciprocal Plot)
Often used in enzyme kinetics, this method can be adapted for receptor-ligand binding studies.
-
Procedure: A double reciprocal plot is constructed by plotting the reciprocal of bound ligand (1/[RL]) against the reciprocal of free ligand (1/[L]).
-
Estimation of Kd: This yields a straight line with a y-intercept of 1/[Rtotal] and a slope of Kd/[Rtotal]. Kd can be estimated from the slope and y-intercept.
-
Strengths: Linearizes the data, simplifies estimation from the plot's parameters.
-
Weaknesses: Similar to the Scatchard plot, it's highly sensitive to experimental error, particularly at low concentrations of ligand. Amplifies the effect of errors at low ligand concentrations.
4. Hanes-Woolf Plot
The Hanes-Woolf plot is an alternative linearization method offering improved robustness compared to the Lineweaver-Burk plot.
-
Procedure: It involves plotting [L]/[RL] against [L].
-
Estimation of Kd: The plot produces a straight line with a slope of 1 and a y-intercept of Kd. Thus, the y-intercept directly provides the Kd value.
-
Strengths: Less sensitive to errors compared to the Lineweaver-Burk plot, simpler interpretation.
-
Weaknesses: Still susceptible to error propagation, particularly at low ligand concentrations. The assumption of a single class of binding sites still applies.
Choosing the Right Graphical Method
The selection of the most suitable graphical method depends on several factors:
-
Data Quality: If the data is noisy or contains outliers, methods like the Scatchard and Lineweaver-Burk plots might be less reliable. In such cases, non-linear regression or the Hanes-Woolf plot might be preferred.
-
Number of Binding Sites: If multiple binding sites with different affinities are suspected, these graphical methods are inappropriate. More complex models would be required.
-
Cooperativity: These methods are typically valid only when the binding process is non-cooperative. If cooperativity is present, different models should be applied.
-
Software Availability: Non-linear regression analysis requires specialized software, which may not always be readily available. The linearization methods, however, can be done using basic graphing tools.
Importance of Non-Linear Regression
While graphical methods offer a quick estimate, non-linear regression analysis is the gold standard for Kd determination. It fits the raw binding data to an appropriate binding equation (often the Hill equation or a related model), providing statistically robust Kd values along with confidence intervals. This approach minimizes the impact of experimental error and allows for rigorous statistical analysis.
Practical Considerations and Limitations
Remember these crucial points:
-
Data quality is paramount. Accurate and precise experimental measurements are essential for reliable Kd estimation using any method.
-
Control experiments are vital. Appropriate controls should be included to account for non-specific binding or other experimental artifacts.
-
Assumptions of the models. The methods discussed here rely on several assumptions (e.g., equilibrium conditions, single binding site, non-cooperative binding). Deviations from these assumptions can lead to inaccurate Kd estimates.
-
Interpreting results carefully. Graphical methods provide estimates, not precise values. The results should always be interpreted with due consideration to the limitations of the method employed and the uncertainty associated with the estimate.
Conclusion
Estimating the dissociation constant (Kd) is an essential task across diverse scientific disciplines. While graphical methods offer convenient visual representations and approximate Kd values, they have inherent limitations. For accurate and reliable Kd determination, non-linear regression analysis applied to high-quality experimental data remains the preferred approach. Choosing the appropriate method necessitates careful consideration of data quality, experimental design, and the potential limitations of each technique. The selection of an accurate and suitable method ensures a robust estimate of Kd and fosters a deeper understanding of molecular interactions.
Latest Posts
Latest Posts
-
How Many Ounces In 1000 Ml
May 19, 2025
-
How Tall Is 184 Cm In Feet
May 19, 2025
-
How Many Ml Is 48 Ounces
May 19, 2025
-
How Much Is 45k Per Hour
May 19, 2025
-
How Many Days Is 900 Hours
May 19, 2025
Related Post
Thank you for visiting our website which covers about Use The Graph To Estimate The Dissociation Constant . We hope the information provided has been useful to you. Feel free to contact us if you have any questions or need further assistance. See you next time and don't miss to bookmark.