Use The Bootstrap Distributions In Figure 1
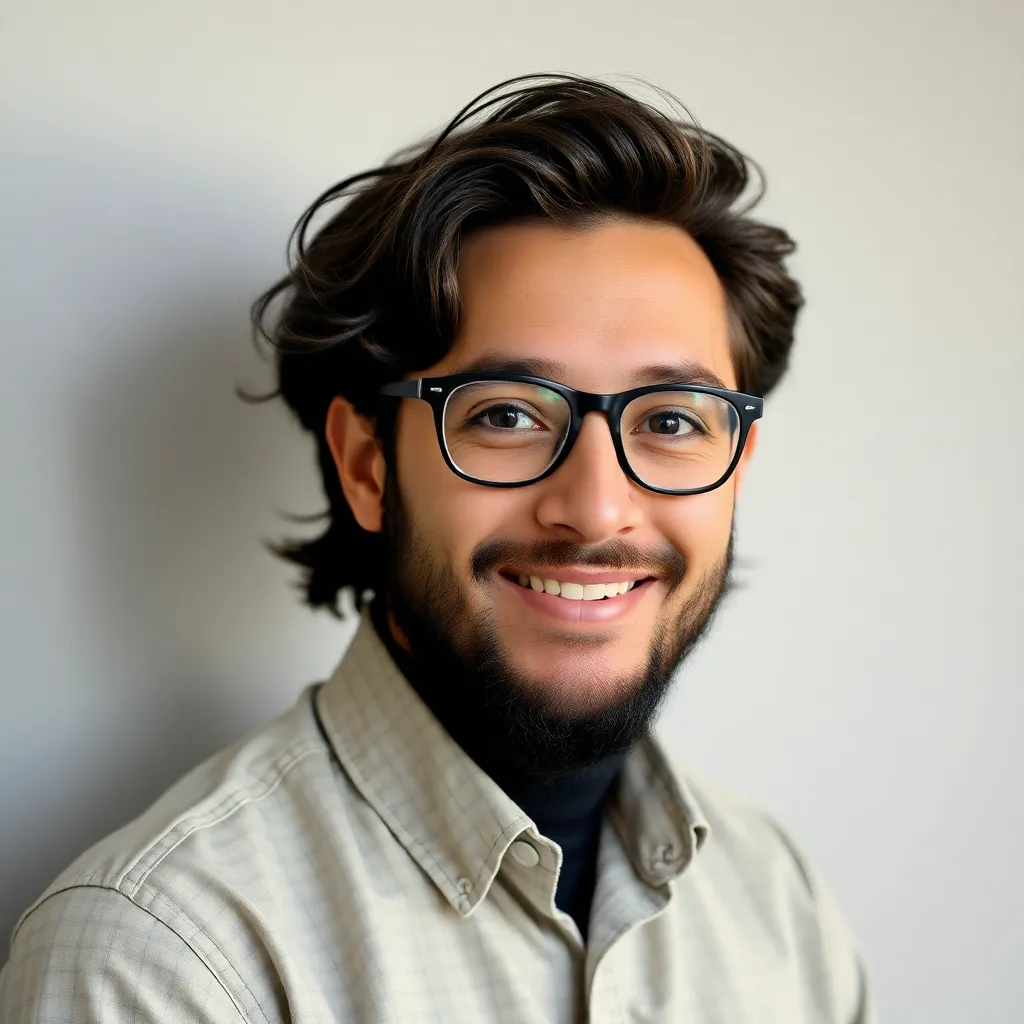
Holbox
May 09, 2025 · 5 min read
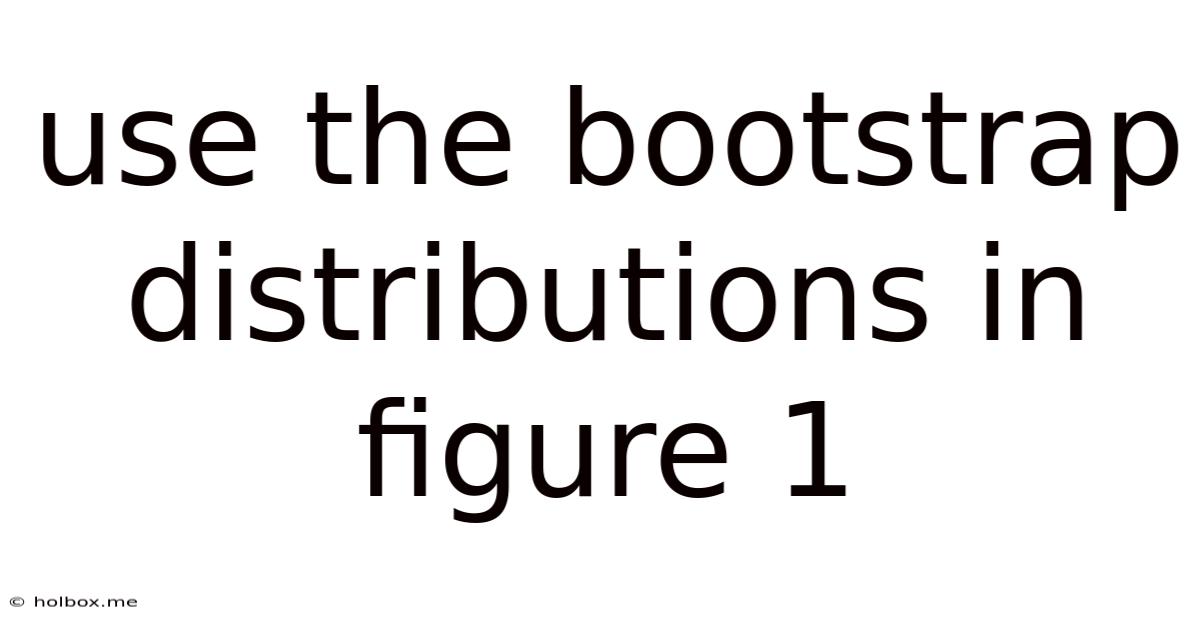
Table of Contents
Understanding and Utilizing Bootstrap Distributions: A Deep Dive into Figure 1 (Illustrative Example)
This article explores the concept of bootstrap distributions, illustrating their application and interpretation using a hypothetical "Figure 1" (as a concrete example, I will create a scenario and corresponding data). We will delve into the practical uses of bootstrap distributions in statistical inference, focusing on confidence intervals and hypothesis testing. The principles discussed here are broadly applicable across various statistical problems.
Understanding Bootstrap Resampling
Before diving into the interpretation of a bootstrap distribution, it's crucial to understand the underlying concept of bootstrap resampling. The bootstrap method is a powerful resampling technique used to estimate the sampling distribution of a statistic. It works by repeatedly drawing random samples with replacement from the original dataset. Each resampled dataset is the same size as the original. The statistic of interest (e.g., mean, median, standard deviation) is calculated for each resampled dataset. The collection of these calculated statistics forms the bootstrap distribution.
Why Use Bootstrap Resampling?
Traditional statistical inference often relies on assumptions about the underlying population distribution (e.g., normality). The bootstrap method is particularly useful when:
- Assumptions about the population distribution are violated: The bootstrap doesn't require assumptions about the population distribution. It works well even with skewed or non-normal data.
- The sampling distribution of a statistic is complex or unknown: The bootstrap provides an empirical estimate of the sampling distribution, regardless of its complexity.
- Analytical solutions are intractable: For complex statistics, the bootstrap offers a computationally efficient way to approximate their sampling distribution.
Illustrative Example: Analyzing Customer Satisfaction Scores (Our Hypothetical "Figure 1")
Let's imagine "Figure 1" represents a histogram of a bootstrap distribution. This histogram is generated from a dataset of customer satisfaction scores (ranging from 1 to 10) for a new product. We have a sample of n=50 customer satisfaction scores. The original sample mean is 7.2. We are interested in estimating the 95% confidence interval for the population mean customer satisfaction score.
(Hypothetical) Figure 1: Bootstrap Distribution of Mean Customer Satisfaction Scores
(Imagine a histogram here showing a roughly bell-shaped distribution centered around 7.2, with the x-axis representing the mean customer satisfaction score and the y-axis representing the frequency of that mean in the bootstrap samples. The histogram would show the distribution of mean scores from many bootstrap resamples.)
Interpreting the Bootstrap Distribution in "Figure 1"
The histogram in "Figure 1" displays the distribution of the mean customer satisfaction scores obtained from numerous bootstrap resamples (let's say 1000 resamples). Several key aspects of this distribution are informative:
-
Center: The center of the distribution (approximately 7.2 in this example) provides an estimate of the population mean. This estimate is usually very close to the mean of the original sample.
-
Spread: The spread or variability of the distribution (represented by its standard deviation, often called the bootstrap standard error) reflects the uncertainty in our estimate of the population mean. A wider spread indicates more uncertainty.
-
Shape: The shape of the distribution provides insights into the sampling distribution of the mean. While often approximately normal (especially with larger sample sizes), it can be skewed or exhibit other characteristics depending on the original data.
Constructing Confidence Intervals using the Bootstrap Distribution
The bootstrap distribution in "Figure 1" allows us to construct a confidence interval for the population mean. For a 95% confidence interval, we need to find the 2.5th percentile and the 97.5th percentile of the bootstrap distribution. These percentiles represent the lower and upper bounds of the confidence interval.
For our example, let's assume the 2.5th percentile of the bootstrap distribution is 6.8 and the 97.5th percentile is 7.6. This means we can be 95% confident that the true population mean customer satisfaction score lies between 6.8 and 7.6.
Hypothesis Testing with Bootstrap Distributions
The bootstrap can also be used for hypothesis testing. Let's say we want to test the null hypothesis that the population mean customer satisfaction score is 7.0 against the alternative hypothesis that it is different from 7.0.
We can use the bootstrap distribution to perform a permutation test. First, we center the bootstrap distribution around the null hypothesis value (7.0). We then calculate the proportion of bootstrap means that are as extreme or more extreme than the observed sample mean (7.2). This proportion represents the p-value. If the p-value is below a pre-defined significance level (e.g., 0.05), we reject the null hypothesis.
Advantages of the Bootstrap Method
-
Non-parametric: The bootstrap method doesn't assume any specific distribution for the data. This makes it applicable to a wide variety of datasets, including those that are not normally distributed.
-
Flexibility: It can be used to estimate the sampling distribution of almost any statistic, not just the mean.
-
Simplicity: While the underlying theory can be complex, the implementation of the bootstrap is relatively straightforward, particularly with statistical software packages.
Limitations of the Bootstrap Method
-
Computational Intensity: Generating a large number of bootstrap resamples can be computationally intensive, especially with large datasets or complex statistics.
-
Bias: The bootstrap estimates can be biased, particularly with small sample sizes or when the statistic of interest is highly sensitive to outliers.
-
Interpretation: Interpreting the bootstrap distribution and its implications requires a good understanding of statistical concepts.
Further Applications and Extensions
Beyond confidence intervals and hypothesis testing, bootstrap methods can be extended to:
-
Estimating bias and variance: The bootstrap provides a way to estimate the bias and variance of an estimator.
-
Estimating prediction intervals: Bootstrap resampling can be used to construct prediction intervals for future observations.
-
Analyzing complex models: Bootstrap methods can be applied to assess the uncertainty in the estimates of parameters in complex statistical models.
Conclusion
The bootstrap is a powerful and versatile tool for statistical inference. It allows us to make reliable inferences about population parameters even when the underlying assumptions of traditional methods are violated. By examining the bootstrap distribution (like our hypothetical "Figure 1"), we can gain valuable insights into the uncertainty surrounding our estimates and make more informed decisions based on the data. The flexibility and relative simplicity of the bootstrap make it a valuable asset in a wide range of statistical applications. Remember that understanding the context of your data and the limitations of the bootstrap method is crucial for accurate and meaningful interpretation of the results. Always consider employing appropriate visualization techniques, like the histogram illustrated in the hypothetical "Figure 1," to effectively communicate your findings.
Latest Posts
Latest Posts
-
How Many Hours In 5 Days
May 18, 2025
-
How Many Pounds Is 900 G
May 18, 2025
-
5 Days Is How Many Hours
May 18, 2025
-
What Is 30 Percent Of 25
May 18, 2025
-
How Many Weeks Is 10 Days
May 18, 2025
Related Post
Thank you for visiting our website which covers about Use The Bootstrap Distributions In Figure 1 . We hope the information provided has been useful to you. Feel free to contact us if you have any questions or need further assistance. See you next time and don't miss to bookmark.