Time Series Data May Exhibit Which Of The Following Behaviors
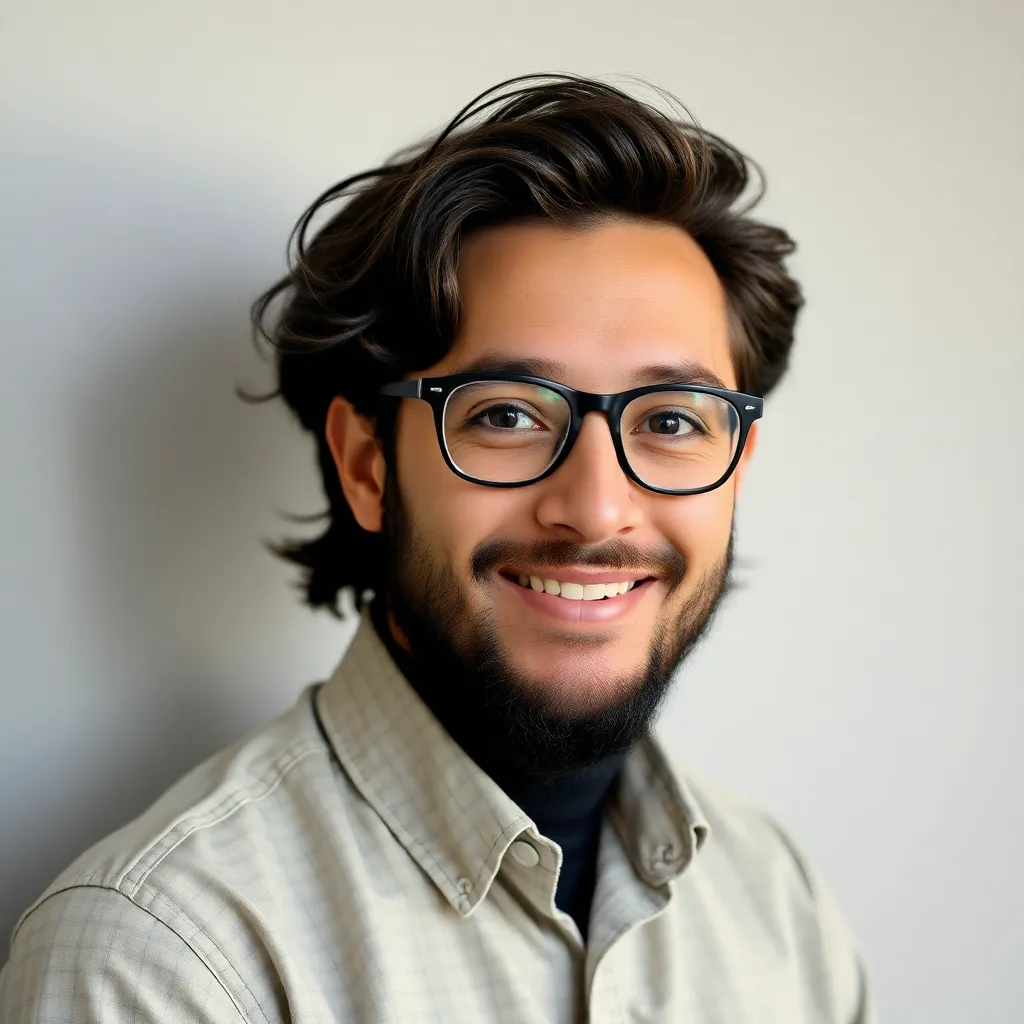
Holbox
Apr 05, 2025 · 6 min read

Table of Contents
- Time Series Data May Exhibit Which Of The Following Behaviors
- Table of Contents
- Time Series Data: Unmasking its Behavioral Quirks
- Key Behaviors of Time Series Data
- 1. Trend: The Long-Term Direction
- 2. Seasonality: Rhythmic Patterns
- 3. Cyclicity: Long-Term Fluctuations
- 4. Randomness (Noise): The Unpredictable Element
- 5. Autocorrelation: The Temporal Dependence
- 6. Heteroscedasticity: Varying Volatility
- 7. Structural Breaks: Abrupt Changes
- Implications for Time Series Analysis
- Advanced Considerations
- 8. Non-linearity: Complex Relationships
- 9. Long Memory: Persistence of Effects
- 10. Multi-variate Time Series: Interdependencies
- Conclusion
- Latest Posts
- Latest Posts
- Related Post
Time Series Data: Unmasking its Behavioral Quirks
Time series data, a sequential collection of data points indexed in time order, is ubiquitous across numerous fields. From stock prices and weather patterns to website traffic and sensor readings, understanding its inherent behaviors is crucial for accurate forecasting and informed decision-making. This article delves deep into the various behavioral quirks exhibited by time series data, exploring their characteristics and implications for analysis.
Key Behaviors of Time Series Data
Time series data, unlike cross-sectional data, possesses a unique temporal dependency. This means that observations are not independent; the value at one point in time is often influenced by previous values. This dependence introduces several characteristic behaviors:
1. Trend: The Long-Term Direction
A trend represents the long-term directional movement of the data. It can be:
- Upward Trend: Data consistently increases over time. Think of the growth of a company's revenue over several years.
- Downward Trend: Data consistently decreases over time. For example, the depletion of a natural resource.
- No Trend (Stationary): The data fluctuates around a constant mean, without a clear upward or downward direction. A stable temperature in a climate-controlled room might exhibit this behavior.
Identifying the trend is a fundamental step in time series analysis. Methods like moving averages and regression techniques can help to estimate and extract the trend component. Understanding the trend is pivotal for forecasting future values. An upward trend suggests future values will likely be higher, while a downward trend suggests the opposite.
2. Seasonality: Rhythmic Patterns
Seasonality refers to cyclical fluctuations that occur at regular intervals. These patterns are often linked to calendar time, such as:
- Daily Seasonality: Variations within a day (e.g., website traffic peaks during working hours).
- Weekly Seasonality: Variations across the days of the week (e.g., higher sales on weekends).
- Monthly Seasonality: Variations across the months (e.g., increased holiday shopping in December).
- Yearly Seasonality: Variations across the years (e.g., higher ice cream sales in summer).
Seasonality introduces predictable patterns that can be exploited for forecasting. Techniques like seasonal decomposition and Fourier analysis can isolate the seasonal component from the data, enabling more accurate predictions. Ignoring seasonality can lead to significantly biased forecasts. For example, forecasting holiday sales without accounting for seasonal peaks would be highly inaccurate.
3. Cyclicity: Long-Term Fluctuations
Cyclicity refers to periodic fluctuations that are longer and less regular than seasonality. Unlike seasonality, which has a fixed period, cycles have variable periods and are often difficult to predict precisely. Examples include:
- Business Cycles: Economic expansions and contractions that occur over several years.
- Climate Cycles: Long-term weather patterns like El Niño and La Niña.
- Technological Cycles: Periods of innovation and disruption in technology sectors.
Identifying cyclical patterns is challenging due to their irregular nature. Methods like spectral analysis can help detect cyclical components, but predicting their timing and magnitude is often uncertain. Understanding cyclicity is crucial for strategic long-term planning. Recognizing a potential economic downturn, for instance, allows businesses to adapt their strategies accordingly.
4. Randomness (Noise): The Unpredictable Element
Randomness, also known as noise, represents the unpredictable fluctuations in the data that cannot be explained by trend, seasonality, or cyclicity. It's the residual variation after accounting for other components. Noise can arise from various sources:
- Measurement Error: Inaccuracies in data collection.
- Unforeseen Events: Unexpected shocks or events that affect the data (e.g., a natural disaster affecting sales).
- Random Variation: Inherent randomness in the underlying process generating the data.
While randomness cannot be predicted, understanding its characteristics is crucial for evaluating the accuracy of forecasts. A high level of noise indicates greater uncertainty in predictions. Techniques like smoothing can help reduce the impact of noise on analysis and forecasting. However, over-smoothing can obscure important underlying patterns.
5. Autocorrelation: The Temporal Dependence
Autocorrelation measures the correlation between data points at different time lags. It quantifies the temporal dependence inherent in time series data. A high autocorrelation indicates strong dependence between consecutive observations, while a low autocorrelation suggests weaker dependence.
Analyzing autocorrelation is essential for understanding the data's dynamics. It helps to identify the presence of trends, seasonality, and cycles. Techniques like the autocorrelation function (ACF) and partial autocorrelation function (PACF) are used to analyze autocorrelation and guide the selection of appropriate forecasting models. Understanding autocorrelation is fundamental for choosing the right model. Models that fail to account for autocorrelation can produce inaccurate and inefficient forecasts.
6. Heteroscedasticity: Varying Volatility
Heteroscedasticity refers to the situation where the variance of the data changes over time. This means that the data's volatility is not constant. Periods of high volatility might be followed by periods of low volatility. For example, stock prices often exhibit heteroscedasticity, with periods of high volatility during market crises and lower volatility during calmer periods.
Heteroscedasticity affects the accuracy of forecasting models. Models that assume constant variance will produce unreliable results if the data is heteroscedastic. Transformations like logarithmic transformations or weighted least squares can be applied to address heteroscedasticity.
7. Structural Breaks: Abrupt Changes
Structural breaks represent abrupt changes in the underlying process generating the data. These changes can be due to various factors, such as policy changes, technological advancements, or unforeseen events. For example, the introduction of a new product might cause a sudden shift in sales data.
Identifying structural breaks is crucial for accurate forecasting. Models that ignore structural breaks will fail to capture the changed dynamics of the data. Techniques like change-point detection methods can help identify the location and magnitude of structural breaks. Ignoring structural breaks can lead to catastrophic forecasting errors.
Implications for Time Series Analysis
Understanding these behaviors is paramount for effective time series analysis. The choice of appropriate analytical techniques depends heavily on the characteristics of the data. For instance:
- Trend analysis informs forecasting models.
- Seasonality adjustments improve forecasting accuracy.
- Autocorrelation analysis guides model selection (e.g., ARIMA models).
- Heteroscedasticity considerations lead to the use of robust techniques.
- Structural break detection necessitates adjustments to the model specification.
Ignoring these behavioral aspects can lead to misleading interpretations and inaccurate predictions. A robust analysis necessitates a thorough examination of the data's characteristics to select appropriate methodologies and avoid potential pitfalls.
Advanced Considerations
Several advanced concepts further enhance our understanding of time series data behavior:
8. Non-linearity: Complex Relationships
Many time series exhibit non-linear relationships, meaning that changes in the data are not simply proportional to past values. Nonlinear models, such as neural networks, are necessary to capture these complex dependencies.
9. Long Memory: Persistence of Effects
Some time series exhibit long memory, where past values continue to influence future values over extended periods. This contrasts with short-memory processes, where the influence of past values decays quickly. Models like fractional ARIMA can handle long-memory processes.
10. Multi-variate Time Series: Interdependencies
Many real-world problems involve multivariate time series, where multiple time series are interconnected. Techniques like vector autoregression (VAR) models are needed to analyze these interdependencies.
Understanding these advanced aspects of time series behavior is crucial for tackling complex real-world problems.
Conclusion
Time series data exhibits a rich array of behaviors, each with significant implications for analysis and forecasting. Understanding trends, seasonality, cyclicity, randomness, autocorrelation, heteroscedasticity, and structural breaks is paramount for accurate modeling and prediction. By carefully considering these characteristics, analysts can build robust models that provide valuable insights and inform effective decision-making across a vast range of applications. The ongoing development of advanced time series methods continues to enhance our capacity to unravel the complexities inherent in this vital data type. Continuous learning and adaptation to new techniques are essential for staying at the forefront of time series analysis.
Latest Posts
Latest Posts
-
Which Of Following Is True About Energy Drinks And Mixers
Apr 12, 2025
-
Write An Equation For The Polynomial Graphed Below
Apr 12, 2025
-
Select The Reagents Necessary To Facilitate The Transformation Shown
Apr 12, 2025
-
Which Medication Is Associated With Sedation As A Side Effect
Apr 12, 2025
-
Draw The Addition Products Formed When One Equivalent
Apr 12, 2025
Related Post
Thank you for visiting our website which covers about Time Series Data May Exhibit Which Of The Following Behaviors . We hope the information provided has been useful to you. Feel free to contact us if you have any questions or need further assistance. See you next time and don't miss to bookmark.