The Two Distributions Have Equal Means And Diffrent Standard Deviations
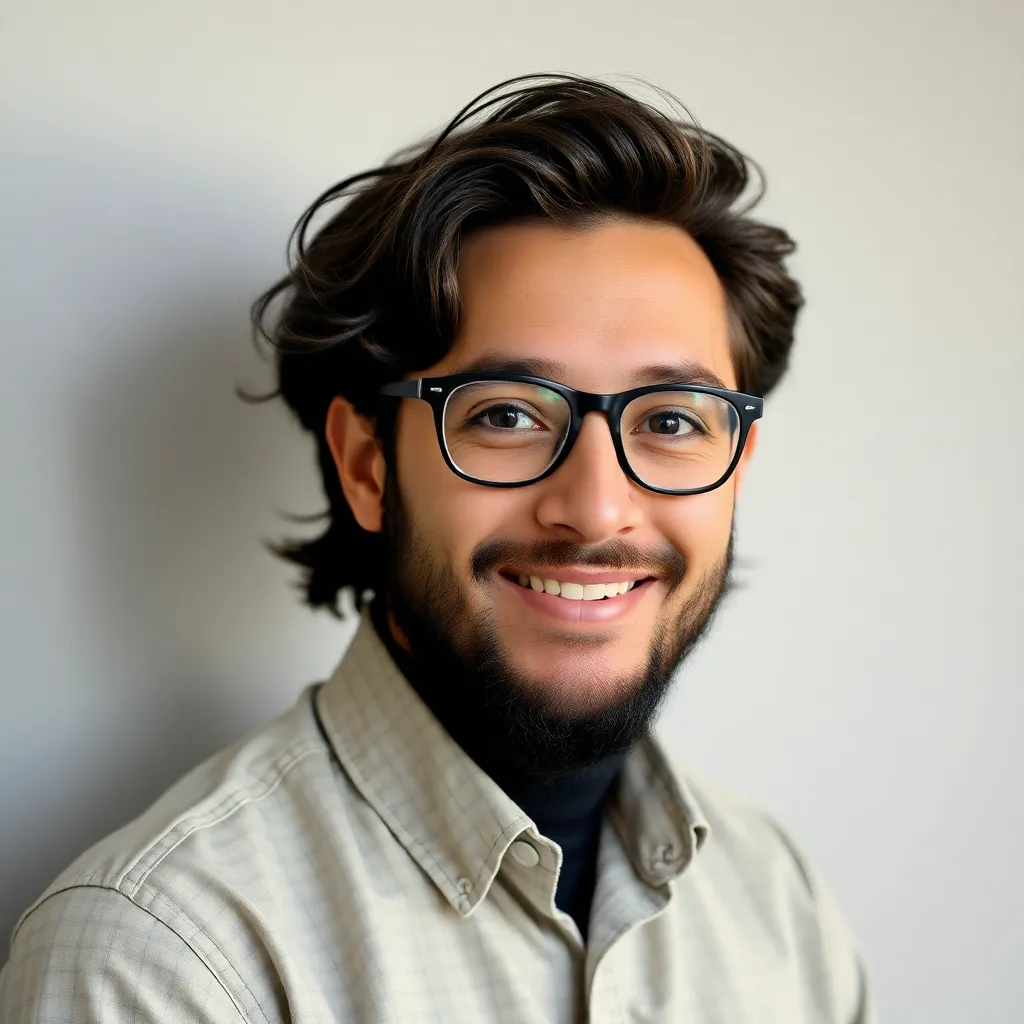
Holbox
Apr 03, 2025 · 5 min read

Table of Contents
- The Two Distributions Have Equal Means And Diffrent Standard Deviations
- Table of Contents
- Two Distributions with Equal Means, Different Standard Deviations: A Deep Dive
- What Does it Mean?
- Visualizing the Difference
- Statistical Significance of the Difference
- 1. Probability and Confidence Intervals
- 2. Hypothesis Testing
- 3. Risk and Uncertainty
- 4. Process Control and Quality Assurance
- Real-World Examples
- Conclusion: The Importance of Standard Deviation
- Latest Posts
- Latest Posts
- Related Post
Two Distributions with Equal Means, Different Standard Deviations: A Deep Dive
Understanding the nuances of statistical distributions is crucial for anyone working with data. While the mean provides a measure of central tendency, the standard deviation reveals the spread or dispersion of the data around that mean. This article delves into the implications of two distributions possessing equal means but differing standard deviations, exploring the consequences across various statistical contexts and providing practical examples.
What Does it Mean?
When we say two distributions have equal means, we mean that the average value of the data points in both distributions is the same. However, when their standard deviations differ, it signifies that the data points in one distribution are more spread out (higher standard deviation) than in the other (lower standard deviation). Imagine two sets of exam scores: both classes have the same average score (equal means), but one class has scores clustered tightly around the average, while the other class has scores ranging widely from very low to very high. This disparity in score distribution is reflected in the difference in their standard deviations.
Key takeaway: Equal means indicate similar central tendencies, while differing standard deviations highlight varying degrees of data dispersion or variability.
Visualizing the Difference
Consider two normal distributions, both with a mean (µ) of 50. Let's say Distribution A has a standard deviation (σ) of 5, while Distribution B has a standard deviation of 10. Visually, Distribution A will be a taller, narrower bell curve, indicating that the data points are concentrated closely around the mean of 50. Distribution B, on the other hand, will be shorter and wider, reflecting the greater spread of data points away from the mean.
Practical Implications: This visual difference translates to significant practical consequences. For instance, if these distributions represent the heights of two populations, Distribution A might represent a population with fairly uniform heights, while Distribution B might represent a population with a wider range of heights.
Statistical Significance of the Difference
The difference in standard deviations, even with equal means, has profound implications for several statistical analyses:
1. Probability and Confidence Intervals
While both distributions have the same mean, the probability of observing a value within a specific range differs significantly. With a smaller standard deviation (Distribution A), there's a higher probability of observing values close to the mean and a lower probability of observing extreme values. Conversely, with a larger standard deviation (Distribution B), there's a higher probability of observing values farther from the mean and a higher likelihood of outliers. This directly affects the width of confidence intervals; Distribution B will have wider confidence intervals for the same confidence level.
2. Hypothesis Testing
When comparing two distributions, standard deviation plays a vital role in hypothesis testing. Statistical tests, like the t-test (for comparing means), are sensitive to variations in standard deviations. If the standard deviations are substantially different, it necessitates the use of more robust statistical methods, such as the Welch's t-test, which accounts for unequal variances. Failing to account for unequal variances can lead to inaccurate conclusions regarding the statistical significance of any observed difference in means.
3. Risk and Uncertainty
In financial modeling, for example, two investment strategies might have equal expected returns (means), but significantly different risk profiles (standard deviations). The strategy with the higher standard deviation entails greater volatility and higher uncertainty, even though the average return might be the same. This underscores that while the average might be the same, the risk associated with achieving that average differs considerably.
4. Process Control and Quality Assurance
In manufacturing, two production lines might produce products with the same average weight (mean), but one line might have a much tighter control over variations in weight (lower standard deviation), resulting in higher quality and fewer defects. The line with a higher standard deviation faces greater quality control challenges due to inconsistencies in output.
Real-World Examples
The concept of equal means and differing standard deviations appears across numerous fields:
-
Education: Two schools might have similar average student test scores, but one school might demonstrate greater variability in student performance (higher standard deviation), suggesting a wider range of student abilities or possibly issues with teaching consistency.
-
Healthcare: Two hospitals might treat patients with similar average recovery times (mean) after a particular surgery, but one hospital might have a higher standard deviation, indicating greater variation in patient outcomes and potentially pointing to inconsistencies in care or patient characteristics.
-
Finance: Two investment funds might have similar average returns, but one fund may exhibit considerably higher volatility (standard deviation), indicating a riskier investment despite the similar average return.
-
Climate Science: Two regions might have similar average temperatures, but one region might display greater variability in daily temperatures (higher standard deviation), reflecting a less stable or predictable climate.
Conclusion: The Importance of Standard Deviation
While the mean provides a valuable summary statistic, it tells only part of the story. The standard deviation provides crucial context regarding the spread and variability of the data. Two distributions with equal means but differing standard deviations showcase the limitations of relying solely on the mean for a complete understanding of data behavior. Analyzing both the mean and the standard deviation provides a more comprehensive and nuanced interpretation of data, leading to more informed decision-making across various disciplines. Understanding the interplay between these two key statistical measures is vital for interpreting data effectively and drawing accurate conclusions. Always consider the standard deviation when analyzing your data to fully appreciate the underlying variability and its implications. Remember that this difference in dispersion impacts probability calculations, hypothesis testing, and risk assessment, making the standard deviation an indispensable tool in data analysis and interpretation.
Latest Posts
Latest Posts
-
Which Of The Following Best Describes The Graph Shown Below
Apr 09, 2025
-
Wellcare Added Which State To Its Footprint For 2025
Apr 09, 2025
-
The Approaches To Quality Assurance Are
Apr 09, 2025
-
Downsizing Is An Effective Way To
Apr 09, 2025
-
Companies Can Improve Job Cost Accuracy By Using
Apr 09, 2025
Related Post
Thank you for visiting our website which covers about The Two Distributions Have Equal Means And Diffrent Standard Deviations . We hope the information provided has been useful to you. Feel free to contact us if you have any questions or need further assistance. See you next time and don't miss to bookmark.