The Sum Of The Deviations About The Mean
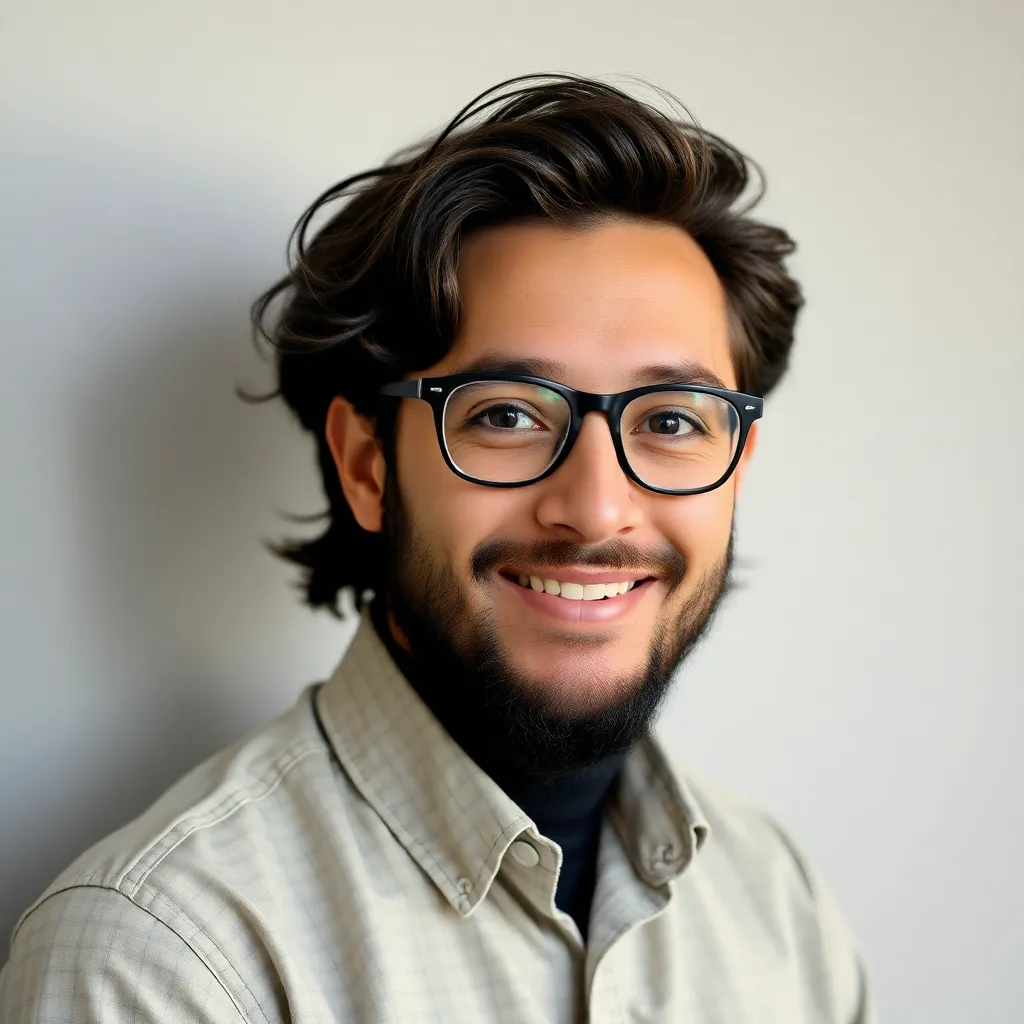
Holbox
May 08, 2025 · 5 min read
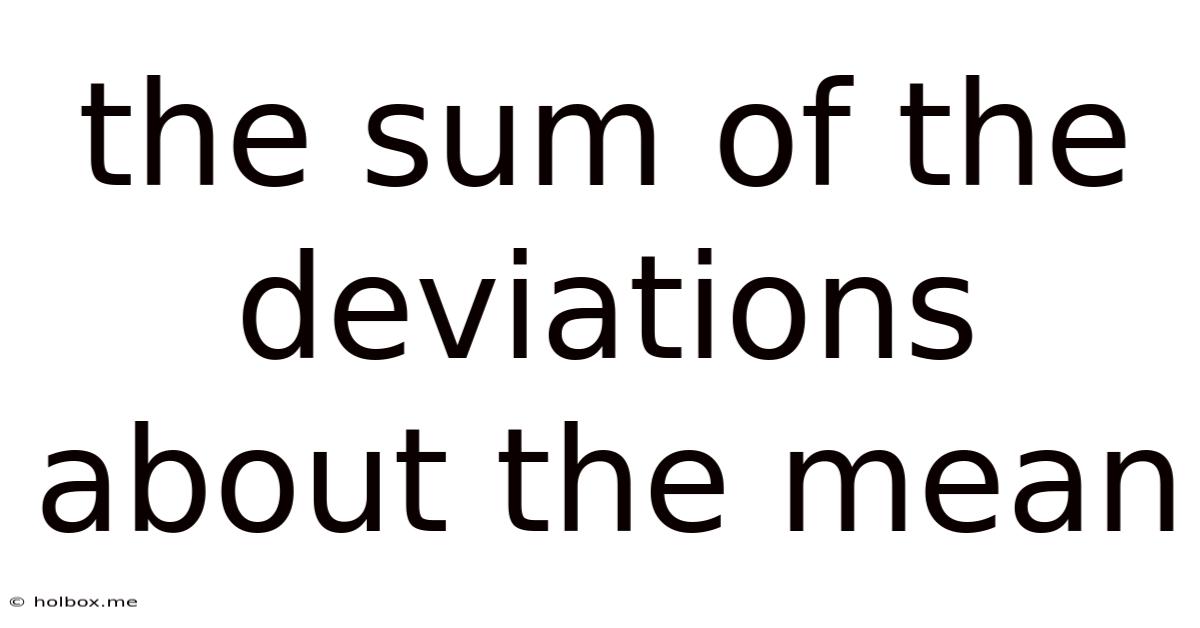
Table of Contents
- The Sum Of The Deviations About The Mean
- Table of Contents
- The Sum of Deviations About the Mean: Why It Matters and How to Use It
- Understanding the Mean and Deviations
- The Mean: A Measure of Central Tendency
- Deviations from the Mean
- The Sum of Deviations About the Mean: Always Zero
- Why the Sum of Deviations is Always Zero: Intuitive Explanation
- Implications and Applications
- 1. Variance and Standard Deviation
- 2. Understanding Data Distribution
- 3. Regression Analysis
- 4. Hypothesis Testing
- Beyond the Sum: Exploring Other Aspects of Deviations
- Analyzing Individual Deviations
- Visualizing Deviations
- Weighted Deviations
- Conclusion: The Significance of a Zero Sum
- Latest Posts
- Latest Posts
- Related Post
The Sum of Deviations About the Mean: Why It Matters and How to Use It
The concept of the sum of deviations about the mean is fundamental in statistics. While seemingly simple, understanding this concept unlocks deeper insights into data analysis and interpretation. This article delves into the sum of deviations about the mean, explaining its properties, practical applications, and its relationship to other statistical measures. We'll explore why this sum is always zero, its implications for understanding data distribution, and its crucial role in calculating variance and standard deviation.
Understanding the Mean and Deviations
Before diving into the sum of deviations, let's solidify our understanding of the mean and deviations.
The Mean: A Measure of Central Tendency
The mean, also known as the average, is a central tendency measure representing the typical value in a dataset. It's calculated by summing all values in the dataset and dividing by the number of values. For example, the mean of the dataset {2, 4, 6, 8} is (2 + 4 + 6 + 8) / 4 = 5.
Deviations from the Mean
A deviation is the difference between an individual data point and the mean of the dataset. Positive deviations indicate data points above the mean, while negative deviations represent data points below the mean. For the dataset {2, 4, 6, 8}, the deviations are:
- 2 - 5 = -3
- 4 - 5 = -1
- 6 - 5 = 1
- 8 - 5 = 3
The Sum of Deviations About the Mean: Always Zero
The crucial property of the sum of deviations about the mean is that it always equals zero. This is not a coincidence; it's a direct consequence of how the mean is defined. The mean is the balancing point of the data; the positive deviations precisely offset the negative deviations.
Mathematical Proof:
Let's represent the dataset as {x₁, x₂, ..., xₙ}, where 'n' is the number of data points. The mean (μ) is calculated as:
μ = (x₁ + x₂ + ... + xₙ) / n
The sum of deviations (Σd) is:
Σd = (x₁ - μ) + (x₂ - μ) + ... + (xₙ - μ)
Expanding this equation, we get:
Σd = x₁ + x₂ + ... + xₙ - nμ
Since μ = (x₁ + x₂ + ... + xₙ) / n, we can substitute this into the equation:
Σd = x₁ + x₂ + ... + xₙ - n[(x₁ + x₂ + ... + xₙ) / n]
Simplifying, we see that the terms cancel out:
Σd = 0
This property holds true regardless of the data distribution—normal, skewed, or otherwise. This seemingly simple fact has profound implications for further statistical analysis.
Why the Sum of Deviations is Always Zero: Intuitive Explanation
Imagine a seesaw perfectly balanced. The mean represents the fulcrum (the balancing point). Each data point is a weight on the seesaw. Positive deviations are weights on one side, pulling down, and negative deviations are weights on the other side, pulling up. Because the mean is the balancing point, the total downward force (positive deviations) must exactly equal the total upward force (negative deviations), resulting in a net force of zero.
Implications and Applications
While the sum of deviations itself is always zero, it's not useless. Its inherent property is crucial for understanding more complex statistical concepts:
1. Variance and Standard Deviation
The sum of deviations' always-zero property necessitates a modification to calculate variability within a dataset. We can't directly use the sum of deviations; instead, we use the sum of squared deviations. This eliminates the canceling effect of positive and negative deviations, providing a measure of dispersion called variance:
Variance (σ²) = Σ(xᵢ - μ)² / (n - 1) (sample variance; using 'n' gives population variance)
The square root of the variance is the standard deviation (σ), which is a more interpretable measure of spread because it's in the same units as the original data. Standard deviation tells us how much individual data points typically deviate from the mean.
2. Understanding Data Distribution
While the sum of deviations is zero, the magnitude of the deviations provides valuable insights into data spread. A dataset with large deviations has high variability, while one with small deviations shows low variability. Analyzing the distribution of these deviations (even though their sum is zero) can reveal patterns and outliers in your data. For example, a large number of unusually high positive deviations might indicate a right-skewed distribution.
3. Regression Analysis
In regression analysis, the sum of deviations plays a crucial role in minimizing the error between predicted and actual values. The "least squares" method aims to find the regression line that minimizes the sum of squared deviations (residuals) between the observed data points and the predicted values on the regression line.
4. Hypothesis Testing
Many statistical hypothesis tests rely on calculating sums of squares. These sums are derived from sums of deviations and are crucial for determining the statistical significance of results. For example, analysis of variance (ANOVA) uses sums of squares to compare the means of multiple groups.
Beyond the Sum: Exploring Other Aspects of Deviations
While the sum of deviations from the mean always equals zero, understanding the individual deviations and their distribution is crucial for a comprehensive analysis.
Analyzing Individual Deviations
Looking at individual deviations can pinpoint outliers or unusual data points that significantly deviate from the mean. These outliers might indicate errors in data collection or interesting phenomena warranting further investigation.
Visualizing Deviations
Creating visual representations, such as histograms or box plots, showing the distribution of deviations can offer valuable insights into the data's spread and symmetry. This visual analysis complements the numerical measures of variance and standard deviation.
Weighted Deviations
In certain situations, some data points might carry more weight than others. For example, in a weighted average, each data point has an associated weight. The sum of weighted deviations can provide a more nuanced view of the data, reflecting the importance of each data point.
Conclusion: The Significance of a Zero Sum
The fact that the sum of deviations about the mean is always zero might seem trivial at first glance. However, this fundamental property underpins many crucial statistical concepts and techniques. Understanding this property is essential for interpreting variance, standard deviation, regression analysis, and various hypothesis tests. By appreciating the implications of this seemingly simple mathematical fact, we gain a deeper understanding of how to analyze and interpret data effectively. While the sum itself provides limited direct information, its role in more complex calculations makes it a cornerstone of statistical analysis. Furthermore, the individual deviations, even though their sum is zero, offer valuable insights into data distribution, variability, and potential outliers. Mastering this concept provides a solid foundation for further exploration into the fascinating world of statistics.
Latest Posts
Latest Posts
-
What Is 110 Degrees Celsius In Fahrenheit
May 19, 2025
-
What Is 31 Degrees Celsius In Fahrenheit
May 19, 2025
-
How Many Grams In 9 Ounces
May 19, 2025
-
How Much Is 110kg In Pounds
May 19, 2025
-
How Many Ounces Is 40 Ml
May 19, 2025
Related Post
Thank you for visiting our website which covers about The Sum Of The Deviations About The Mean . We hope the information provided has been useful to you. Feel free to contact us if you have any questions or need further assistance. See you next time and don't miss to bookmark.