The Standard Deviation Is Used In Conjunction With The
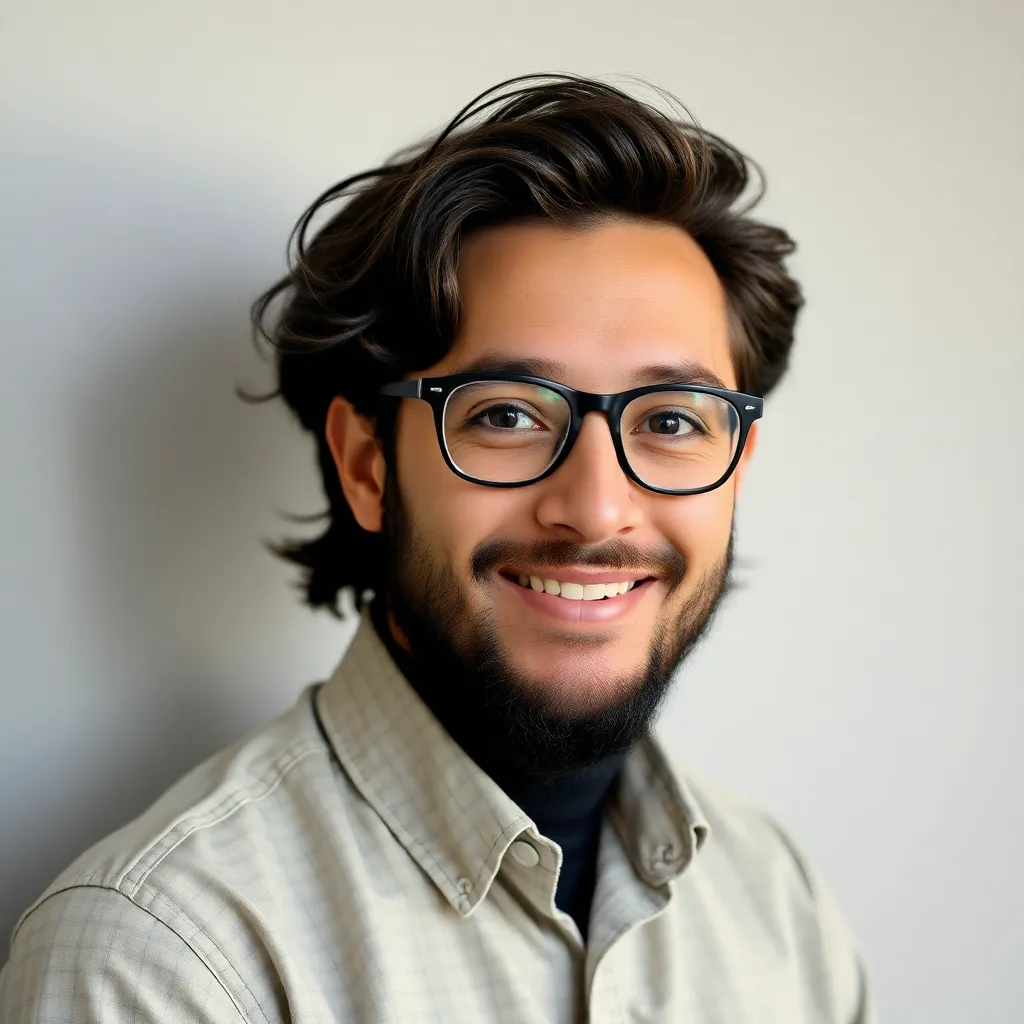
Holbox
May 12, 2025 · 6 min read
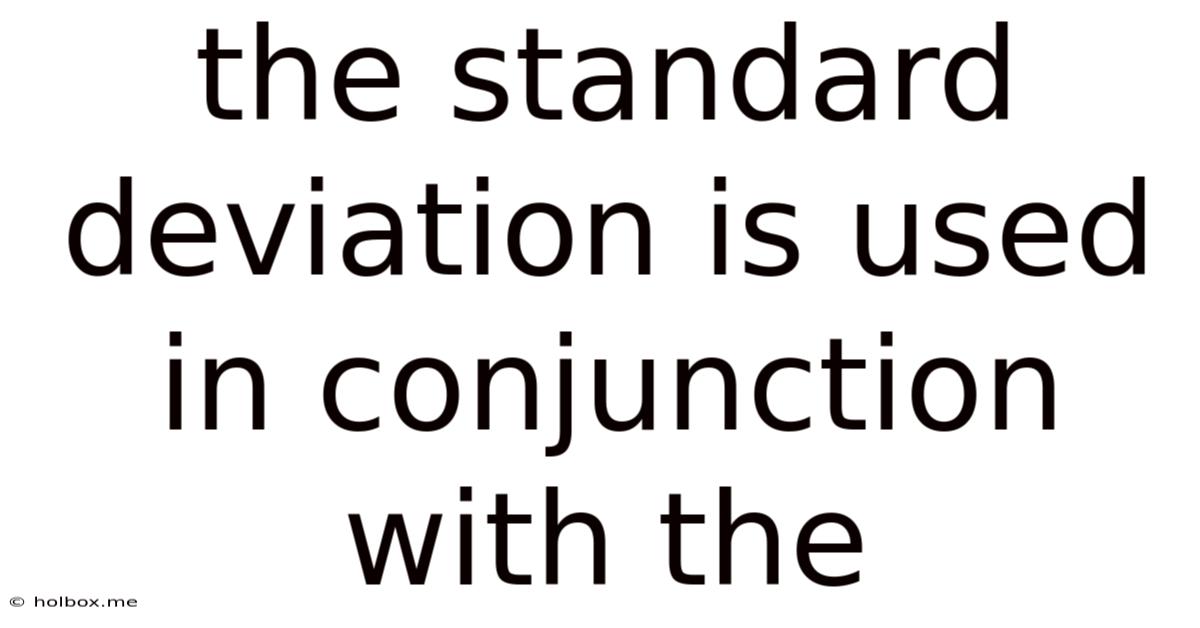
Table of Contents
- The Standard Deviation Is Used In Conjunction With The
- Table of Contents
- The Standard Deviation: Used in Conjunction With the Mean for Powerful Insights
- Understanding the Mean and Standard Deviation
- The Mean: A Measure of Central Tendency
- The Standard Deviation: A Measure of Dispersion
- The Power of the Mean and Standard Deviation Together
- 1. Interpreting Data Distribution: Beyond the Average
- 2. Identifying Outliers and Anomalies
- 3. Making Informed Comparisons
- 4. Probability and Prediction: The Normal Distribution
- 5. Quality Control and Process Monitoring
- Applications Across Diverse Fields
- 1. Finance: Risk Management and Investment Analysis
- 2. Healthcare: Evaluating Treatment Effectiveness
- 3. Meteorology: Weather Forecasting and Climate Analysis
- 4. Education: Assessing Student Performance and Identifying Learning Gaps
- 5. Social Sciences: Understanding Social Trends and Demographics
- Conclusion: A Powerful Duo for Data Analysis
- Latest Posts
- Latest Posts
- Related Post
The Standard Deviation: Used in Conjunction With the Mean for Powerful Insights
The standard deviation, a cornerstone of descriptive statistics, isn't used in isolation. Its true power lies in its synergistic relationship with the mean, providing a comprehensive understanding of data distribution and variability. This article delves deep into this crucial relationship, exploring how the standard deviation, when paired with the mean, unlocks valuable insights across diverse fields.
Understanding the Mean and Standard Deviation
Before we explore their synergistic relationship, let's briefly review each concept individually.
The Mean: A Measure of Central Tendency
The mean, or average, represents the central point of a dataset. It's calculated by summing all data points and dividing by the total number of data points. While simple to compute, the mean alone provides a limited picture of the data. It doesn't reveal how spread out the data points are. A dataset with a mean of 50 could be tightly clustered around 50 or highly dispersed, with some values significantly higher and some significantly lower.
The Standard Deviation: A Measure of Dispersion
This is where the standard deviation steps in. It quantifies the dispersion or spread of the data around the mean. A low standard deviation indicates that the data points are clustered closely around the mean, signifying low variability. Conversely, a high standard deviation suggests that the data points are widely dispersed, indicating high variability. It essentially tells us how much individual data points deviate from the average.
Calculating the Standard Deviation: The calculation involves finding the difference between each data point and the mean, squaring these differences (to eliminate negative values), averaging the squared differences (to get the variance), and finally taking the square root of the variance to obtain the standard deviation. This process ensures that outliers have a proportionally larger influence on the standard deviation, reflecting their impact on the overall spread.
The Power of the Mean and Standard Deviation Together
The true power of these statistical measures emerges when they are used together. The mean provides the central location, while the standard deviation illuminates the data's spread around that central point. This combined information gives a much richer understanding than either measure alone.
1. Interpreting Data Distribution: Beyond the Average
Consider two datasets with the same mean (e.g., 70). One dataset might have a low standard deviation, indicating that most scores are clustered closely around 70. The other might have a high standard deviation, meaning the scores are more widely scattered, with some significantly higher and some significantly lower than 70. The standard deviation provides crucial context to the mean, revealing the shape of the data distribution.
2. Identifying Outliers and Anomalies
By examining the relationship between the mean and standard deviation, we can identify outliers—data points that deviate significantly from the norm. Using techniques like the Z-score (calculated by subtracting the mean from a data point and dividing by the standard deviation), we can determine how many standard deviations a data point lies from the mean. Outliers often warrant further investigation, as they might indicate errors in data collection or reveal unusual events deserving special attention.
3. Making Informed Comparisons
When comparing two or more datasets, considering only the means can be misleading. The standard deviations must also be taken into account. For instance, two groups might have similar mean test scores, but one group could have a significantly higher standard deviation, suggesting greater variability in student performance within that group. This information is critical for educational interventions and resource allocation.
4. Probability and Prediction: The Normal Distribution
The combination of mean and standard deviation is particularly powerful when dealing with normally distributed data. The normal distribution, often depicted as a bell curve, is a symmetrical probability distribution where data points cluster around the mean. In a normal distribution, a specific percentage of data falls within a certain number of standard deviations from the mean:
- 68% of data falls within one standard deviation of the mean.
- 95% of data falls within two standard deviations of the mean.
- 99.7% of data falls within three standard deviations of the mean.
This knowledge allows us to make probability estimations and predictions about future data points within the dataset. For instance, if we know the mean height and standard deviation of adult males in a population, we can estimate the probability that a randomly selected male will fall within a certain height range.
5. Quality Control and Process Monitoring
In manufacturing and other industries, the mean and standard deviation are essential tools for quality control. By monitoring the mean and standard deviation of key product characteristics, manufacturers can ensure that their production processes remain stable and meet quality standards. Significant deviations from established mean and standard deviation parameters can signal potential problems requiring immediate attention.
Applications Across Diverse Fields
The powerful synergy between the mean and standard deviation extends across numerous fields:
1. Finance: Risk Management and Investment Analysis
In finance, the standard deviation is a critical measure of risk. A high standard deviation in investment returns indicates greater volatility and higher risk. Investors often use the mean and standard deviation to assess the risk-reward profile of different investment options. The Sharpe ratio, a commonly used metric, directly incorporates both mean return and standard deviation to evaluate investment performance relative to risk.
2. Healthcare: Evaluating Treatment Effectiveness
In clinical trials, the mean and standard deviation are used to assess the effectiveness of new treatments. By comparing the mean outcomes (e.g., blood pressure, recovery time) in treatment and control groups, along with their standard deviations, researchers can determine if the treatment is statistically significant and if it produces consistent results.
3. Meteorology: Weather Forecasting and Climate Analysis
Meteorologists use the mean and standard deviation to analyze weather patterns and make forecasts. For example, they might analyze the mean temperature and standard deviation over a period to predict the likelihood of extreme weather events. Similarly, climate scientists utilize these metrics to study long-term climate trends and assess the impact of climate change.
4. Education: Assessing Student Performance and Identifying Learning Gaps
Educators use the mean and standard deviation to analyze student performance on tests and assessments. This helps identify areas where students are struggling and inform teaching strategies. The standard deviation highlights the variability in student performance, indicating whether certain groups of students require additional support.
5. Social Sciences: Understanding Social Trends and Demographics
Social scientists use the mean and standard deviation to analyze social data, such as income levels, crime rates, and public opinion. These measures reveal trends and patterns within populations and inform social policies and programs. For instance, analyzing the mean income and standard deviation can highlight income inequality within a community.
Conclusion: A Powerful Duo for Data Analysis
The mean and standard deviation are not merely individual statistical measures; they are a powerful duo that provides a far more comprehensive understanding of data than either measure alone. Their combined use allows us to interpret data distributions, identify outliers, make informed comparisons, understand probability, monitor processes, and derive meaningful insights across diverse fields. Understanding this synergistic relationship is crucial for anyone working with data, from scientists and analysts to investors and educators. By leveraging the power of the mean and standard deviation, we can extract richer meaning from data and make more informed decisions. This understanding forms a fundamental building block for more advanced statistical analysis and data-driven decision-making.
Latest Posts
Latest Posts
-
How Many Ounces In 475 Grams
May 19, 2025
-
What Is Ten Percent Of 80
May 19, 2025
-
How Much Is 43 Kg In Lbs
May 19, 2025
-
What Is 64 Inches In Feet
May 19, 2025
-
How Many Inches Is 75 Mm
May 19, 2025
Related Post
Thank you for visiting our website which covers about The Standard Deviation Is Used In Conjunction With The . We hope the information provided has been useful to you. Feel free to contact us if you have any questions or need further assistance. See you next time and don't miss to bookmark.