The Square Of The Standard Deviation Is Called The
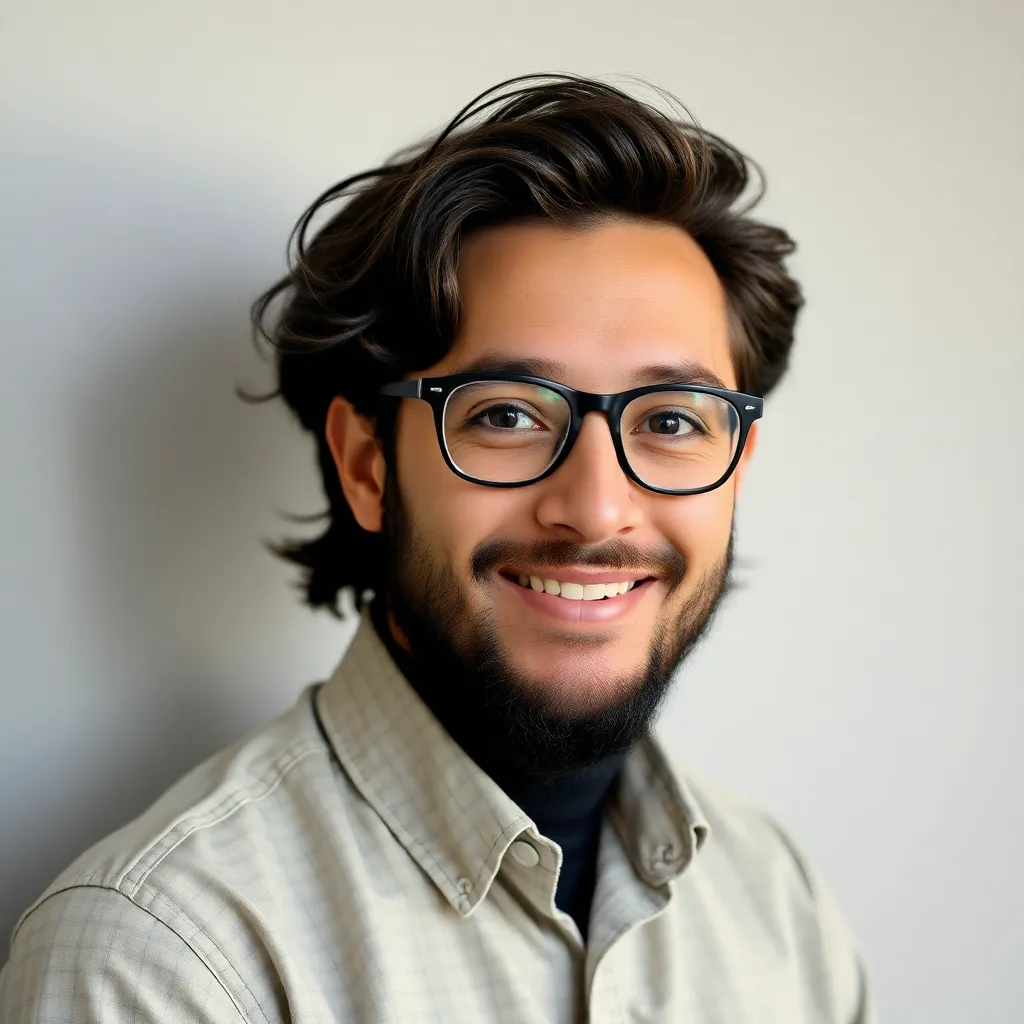
Holbox
Apr 25, 2025 · 6 min read
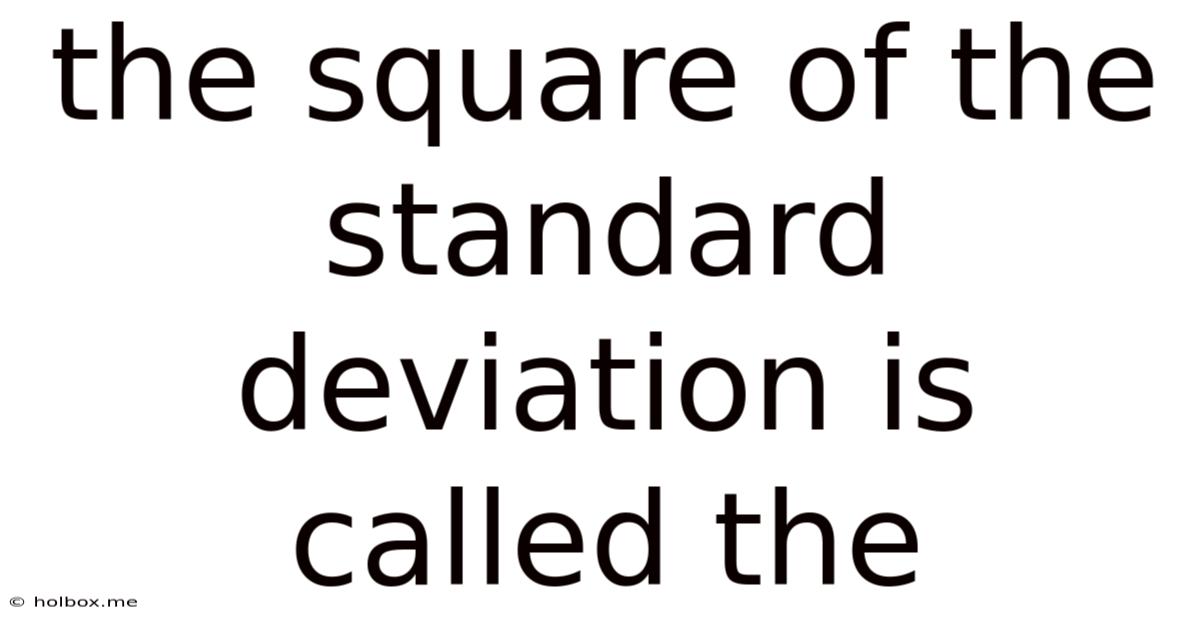
Table of Contents
- The Square Of The Standard Deviation Is Called The
- Table of Contents
- The Square of the Standard Deviation is Called the Variance: A Deep Dive into Statistical Dispersion
- Understanding Variance: A Measure of Data Spread
- Variance vs. Standard Deviation: What's the Difference?
- Calculating Variance: A Step-by-Step Guide
- Calculating Population Variance
- Calculating Sample Variance
- Understanding the Significance of Variance
- Interpreting Variance: What Does it Tell Us?
- Variance in Different Contexts: Practical Applications
- Finance: Portfolio Risk Management
- Engineering: Quality Control and Process Optimization
- Environmental Science: Climate Change Analysis
- Healthcare: Evaluating Treatment Efficacy
- Conclusion: Variance – A Foundation of Statistical Analysis
- Latest Posts
- Latest Posts
- Related Post
The Square of the Standard Deviation is Called the Variance: A Deep Dive into Statistical Dispersion
The square of the standard deviation is called the variance. While both metrics measure the spread or dispersion of a dataset, understanding their differences and the reasons behind using one over the other is crucial for effective data analysis and interpretation. This article will delve deep into the concept of variance, its calculation, interpretation, and its relationship with the standard deviation, exploring its significance in various statistical applications.
Understanding Variance: A Measure of Data Spread
In statistics, variance quantifies the degree of spread or dispersion in a dataset. A high variance indicates that the data points are far from the mean (average), while a low variance suggests that the data points are clustered closely around the mean. It's a crucial measure for understanding data variability and making informed decisions based on the data's characteristics. Think of it as a summary statistic that tells you how much the individual data points deviate from the average value.
Variance vs. Standard Deviation: What's the Difference?
The standard deviation and variance are closely related, yet distinct, measures of dispersion. The standard deviation is simply the square root of the variance. While variance provides a measure of dispersion in squared units (e.g., squared dollars, squared centimeters), the standard deviation returns a value in the original units, making it easier to interpret and directly compare with the mean.
The choice between using variance or standard deviation often depends on the context and the specific application. While standard deviation is often preferred for its interpretability, variance plays a vital role in many statistical calculations and theoretical frameworks. For instance, variance is a fundamental component in calculating the sample variance of a population.
Calculating Variance: A Step-by-Step Guide
Calculating the variance involves several steps, which we'll break down for both population and sample data.
Calculating Population Variance
The population variance (σ²) represents the average of the squared differences from the mean for an entire population. Here's how to calculate it:
-
Calculate the mean (μ): Sum all the data points and divide by the total number of data points (N).
μ = Σxᵢ / N
-
Calculate the squared differences: For each data point (xᵢ), subtract the mean (μ) and square the result:
(xᵢ - μ)²
-
Sum the squared differences: Add up all the squared differences from step 2:
Σ(xᵢ - μ)²
-
Divide by the population size: Divide the sum of squared differences by the total number of data points (N):
σ² = Σ(xᵢ - μ)² / N
Calculating Sample Variance
The sample variance (s²) estimates the population variance using a sample of data. A crucial difference lies in the denominator used for the calculation. Using N in the sample variance would underestimate the population variance. To compensate for this, we divide by N-1, which is known as Bessel's correction. Here's how to calculate the sample variance:
-
Calculate the sample mean (x̄): Sum all the data points and divide by the number of data points (n).
x̄ = Σxᵢ / n
-
Calculate the squared differences: For each data point (xᵢ), subtract the sample mean (x̄) and square the result:
(xᵢ - x̄)²
-
Sum the squared differences: Add up all the squared differences from step 2:
Σ(xᵢ - x̄)²
-
Divide by (n-1): Divide the sum of squared differences by (n-1), where n is the sample size:
s² = Σ(xᵢ - x̄)² / (n-1)
Understanding the Significance of Variance
The variance, despite its less intuitive interpretation compared to standard deviation, holds significant importance in various statistical contexts:
-
ANOVA (Analysis of Variance): Variance is the cornerstone of ANOVA, a powerful statistical test used to compare the means of two or more groups. ANOVA assesses whether the differences in group means are statistically significant or due to random chance by analyzing the variance within and between groups.
-
Regression Analysis: In regression analysis, variance plays a crucial role in determining the goodness-of-fit of a model. The variance explained by the model is compared to the total variance in the data to assess the model's predictive power (R-squared).
-
Probability Distributions: Variance is a key parameter in defining various probability distributions, such as the normal distribution, where it determines the spread of the distribution.
-
Financial Modeling: In finance, variance is often used to measure the risk associated with an investment. A higher variance indicates greater volatility and higher risk.
-
Quality Control: In manufacturing and quality control, variance helps to monitor the consistency and variability of a production process. Low variance suggests a consistent process, while high variance indicates inconsistencies requiring attention.
Interpreting Variance: What Does it Tell Us?
While the standard deviation provides a more intuitive measure of dispersion (in the same units as the data), understanding variance offers valuable insights:
-
Magnitude of Spread: A larger variance indicates a greater spread of data around the mean. Conversely, a smaller variance signifies that the data points are clustered tightly around the mean.
-
Comparison Across Datasets: Variance allows for comparing the variability of different datasets, even if the units of measurement differ. While the standard deviation values themselves might be in different units, the variance, being in squared units, facilitates comparison.
-
Identifying Outliers: A high variance often points towards the presence of outliers, which are data points that significantly differ from the rest of the data.
-
Model Evaluation: In statistical modeling, variance is critical for assessing the goodness-of-fit and predictive ability of the model.
Variance in Different Contexts: Practical Applications
Let's explore some practical applications of variance across different fields:
Finance: Portfolio Risk Management
In the world of finance, variance (and its square root, standard deviation) is a fundamental concept for measuring the risk associated with investments. A high variance in stock prices signifies significant price fluctuations and a higher risk for investors. Portfolio managers use variance to optimize portfolios, aiming to achieve the desired return with the lowest possible risk.
Engineering: Quality Control and Process Optimization
In engineering and manufacturing, variance is critical for quality control. By monitoring the variance of key product parameters, manufacturers can identify and rectify inconsistencies in the production process. A lower variance suggests better process control, and hence higher product quality.
Environmental Science: Climate Change Analysis
Environmental scientists utilize variance to analyze climate data, examining fluctuations in temperature, rainfall, and other climate parameters. Understanding the variance of climate variables helps assess the degree of variability and identify trends in climate patterns over time.
Healthcare: Evaluating Treatment Efficacy
In healthcare, variance plays a crucial role in evaluating the efficacy of different treatments. By analyzing the variance in patient outcomes, researchers can determine the effectiveness of a treatment and identify whether the variability in outcomes is statistically significant.
Conclusion: Variance – A Foundation of Statistical Analysis
The square of the standard deviation, the variance, is a fundamental concept in statistics, providing a powerful measure of data dispersion. While the standard deviation offers a more easily interpretable measure of spread, the variance plays a crucial role in various statistical methods and applications, particularly ANOVA and regression analysis. Understanding both variance and standard deviation is critical for effective data analysis, interpretation, and decision-making across diverse fields. Whether analyzing financial risks, optimizing manufacturing processes, or evaluating climate trends, the ability to calculate and interpret variance is essential for any data scientist or analyst.
Latest Posts
Latest Posts
-
The Graph Demonstrates That Changes In Investment
May 07, 2025
-
Indicate Which Compounds Below Can Have Diastereomers And Which Cannot
May 07, 2025
-
The Stock Of Fdi Refers To The
May 07, 2025
-
Po Box 98878 Las Vegas Nevada
May 07, 2025
-
A Tale Of Two Gases Answer Key
May 07, 2025
Related Post
Thank you for visiting our website which covers about The Square Of The Standard Deviation Is Called The . We hope the information provided has been useful to you. Feel free to contact us if you have any questions or need further assistance. See you next time and don't miss to bookmark.