The Probability Distribution Of Is Called A
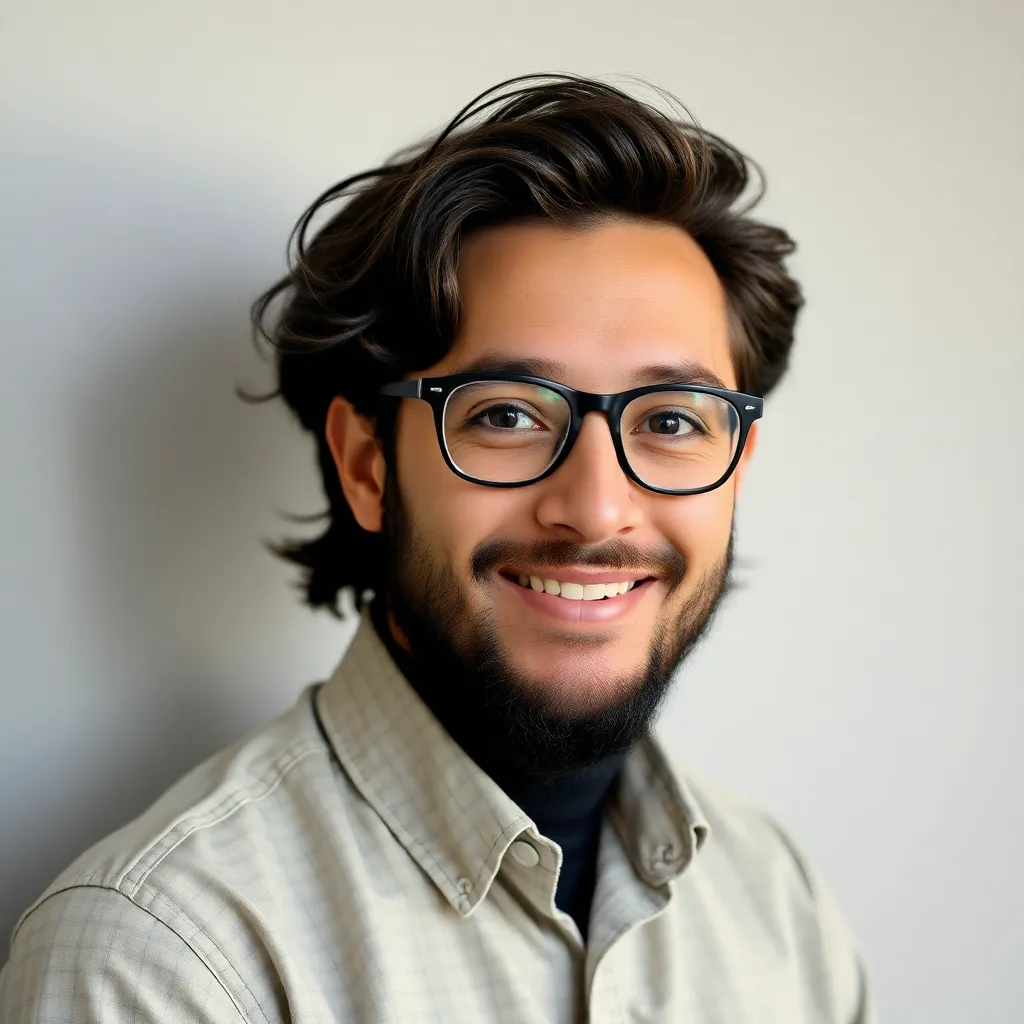
Holbox
Mar 31, 2025 · 7 min read

Table of Contents
- The Probability Distribution Of Is Called A
- Table of Contents
- The Probability Distribution of X is Called a... A Comprehensive Guide
- Understanding Probability Distributions: A Foundation
- 1. Discrete Probability Distributions:
- 2. Continuous Probability Distributions:
- Determining the Appropriate Probability Distribution
- Detailed Examples and Applications
- Advanced Considerations and Challenges
- Conclusion: The Importance of Correct Distribution Selection
- Latest Posts
- Latest Posts
- Related Post
The Probability Distribution of X is Called a... A Comprehensive Guide
Determining the correct name for a probability distribution hinges on understanding its underlying characteristics and the mathematical functions that define it. This article delves into various common and less-common probability distributions, exploring their properties and identifying scenarios where each is applicable. We'll focus on understanding how the distribution of a random variable (X) is determined and categorized. This isn't just an exercise in theoretical statistics; understanding these distributions is crucial for data analysis, statistical modeling, and making informed decisions in numerous fields.
Understanding Probability Distributions: A Foundation
Before diving into specific distributions, let's establish a foundational understanding. A probability distribution describes the likelihood of obtaining different possible values for a random variable. This variable, often denoted as X, can represent various phenomena, from the height of students in a class to the number of defects in a manufactured product. Probability distributions are classified into two main categories:
1. Discrete Probability Distributions:
These distributions deal with discrete random variables, which can only take on a finite number of values or a countably infinite number of values. Examples include:
- The number of heads when flipping a coin three times: This can only be 0, 1, 2, or 3.
- The number of cars passing a certain point on a highway in an hour: This is a countably infinite value (though practically limited).
Common discrete distributions include:
- Bernoulli Distribution: Models the probability of success or failure in a single trial (e.g., flipping a coin once).
- Binomial Distribution: Describes the probability of getting a certain number of successes in a fixed number of independent Bernoulli trials (e.g., the number of heads in 10 coin flips).
- Poisson Distribution: Models the probability of a given number of events occurring in a fixed interval of time or space, given a known average rate (e.g., the number of customers arriving at a store per hour).
- Geometric Distribution: Describes the probability of the number of trials needed to achieve the first success in a sequence of independent Bernoulli trials.
- Negative Binomial Distribution: Generalizes the geometric distribution, describing the probability of the number of trials needed to achieve a specified number of successes.
- Hypergeometric Distribution: Models the probability of drawing a certain number of successes in a sample without replacement from a finite population.
2. Continuous Probability Distributions:
These distributions deal with continuous random variables, which can take on any value within a given range. Examples include:
- The height of a person: Height can take on any value within a certain range.
- The temperature of a room: Temperature can be any value within a realistic range.
Common continuous distributions include:
- Uniform Distribution: All values within a given range have an equal probability of occurring.
- Normal Distribution (Gaussian Distribution): A bell-shaped curve, characterized by its mean (μ) and standard deviation (σ). It's incredibly common in nature and statistics.
- Exponential Distribution: Models the time until an event occurs in a Poisson process (e.g., the time until a machine breaks down).
- Gamma Distribution: A generalization of the exponential distribution, useful for modeling waiting times and other positive-valued variables.
- Beta Distribution: Defined on the interval [0, 1], often used to model probabilities or proportions.
- Chi-Square Distribution: Used in hypothesis testing and arises from the sum of squared standard normal variables.
- t-Distribution (Student's t-Distribution): Similar to the normal distribution but with heavier tails, used in hypothesis testing with small sample sizes.
- F-Distribution: Used in ANOVA (analysis of variance) to compare variances of different groups.
- Weibull Distribution: Used in reliability engineering to model the time until failure of a component.
Determining the Appropriate Probability Distribution
The choice of the appropriate probability distribution depends heavily on the nature of the data and the context of the problem. Here’s a breakdown of how to approach this crucial step:
-
Identify the type of random variable: Is it discrete or continuous? This immediately narrows down the possibilities.
-
Examine the data: Create histograms, box plots, and other visual representations of the data to understand its shape, central tendency, and dispersion. Does the data appear symmetric, skewed, or multimodal?
-
Consider the underlying process: What is the mechanism generating the data? Is it a sequence of independent trials (suggesting binomial or geometric distributions), a rate-based process (suggesting Poisson or exponential distributions), or something else?
-
Check for common patterns: Does the data resemble a known distribution? This often involves comparing the data's characteristics (mean, variance, skewness, kurtosis) to the theoretical properties of various distributions. Statistical software packages can help with this.
-
Perform goodness-of-fit tests: These tests formally assess whether the data fits a particular distribution. Examples include the chi-squared test and the Kolmogorov-Smirnov test.
Detailed Examples and Applications
Let's examine some specific examples to solidify our understanding:
Example 1: The Number of Defective Items in a Batch
Suppose a batch of 100 items is produced, and the probability of any individual item being defective is 0.05. We want to find the probability of exactly 3 defective items in the batch. The appropriate distribution here is the binomial distribution, as we have a fixed number of independent trials (items) with a constant probability of success (defect).
Example 2: The Time Until a Machine Malfunctions
A machine’s time to failure follows an exponential distribution with a mean time to failure of 1000 hours. We want to find the probability that the machine will last more than 1500 hours. The exponential distribution is suitable because it models the time until an event (failure) occurs in a Poisson process, which often applies to equipment lifespans.
Example 3: The Height of Students
The heights of students in a large class are likely to follow a normal distribution. This is because many natural phenomena exhibit a bell-shaped distribution, and height is often influenced by numerous independent factors.
Example 4: The Distribution of Rainfall
Rainfall amounts in a given region over a year might be better modeled by a gamma distribution due to the positive values and potential for skewed data. The Gamma distribution allows for a wider variety of shapes than the normal distribution, accommodating the potential for higher probabilities at lower values and a longer tail for extreme events.
Example 5: Customer Arrivals at a Store
The number of customers arriving at a store during a specific time interval can often be modeled using a Poisson distribution. The key here is the rate of arrival, which is assumed to be constant and independent of previous arrivals.
Advanced Considerations and Challenges
Choosing the correct distribution isn't always straightforward. Several challenges can arise:
- Data limitations: Small sample sizes can make it difficult to accurately assess the distribution.
- Mixed distributions: Real-world data might not perfectly fit any single distribution; it may be a mixture of several distributions.
- Model misspecification: Choosing an incorrect distribution can lead to flawed conclusions in statistical analyses.
In these situations, more advanced techniques, such as mixture models or non-parametric methods, might be necessary.
Conclusion: The Importance of Correct Distribution Selection
Choosing the correct probability distribution is fundamental to accurate statistical analysis, modeling, and prediction. Understanding the characteristics of different distributions, their applications, and the methods for selecting the appropriate distribution is vital for any data scientist, statistician, or anyone working with data-driven decision making. By carefully considering the nature of the data and the underlying process, you can ensure that your analyses are based on a solid foundation, leading to more reliable and insightful results. Remember to always visualize your data and consider the underlying process to aid in your selection, making your work more robust and meaningful. The right distribution is the key to unlocking the true story your data is trying to tell.
Latest Posts
Latest Posts
-
If The Following Items Needed To Be Stored
Apr 03, 2025
-
Comparative Advantage Refers To The Ability To
Apr 03, 2025
-
One Of The Dangers Of Self Report Personality Measures Is That
Apr 03, 2025
-
What Op Amp Circuit Configuration Is This
Apr 03, 2025
-
Art Labeling Activity Overview Of Cranial Nerves
Apr 03, 2025
Related Post
Thank you for visiting our website which covers about The Probability Distribution Of Is Called A . We hope the information provided has been useful to you. Feel free to contact us if you have any questions or need further assistance. See you next time and don't miss to bookmark.