The Distribution Of Z Scores Is Always
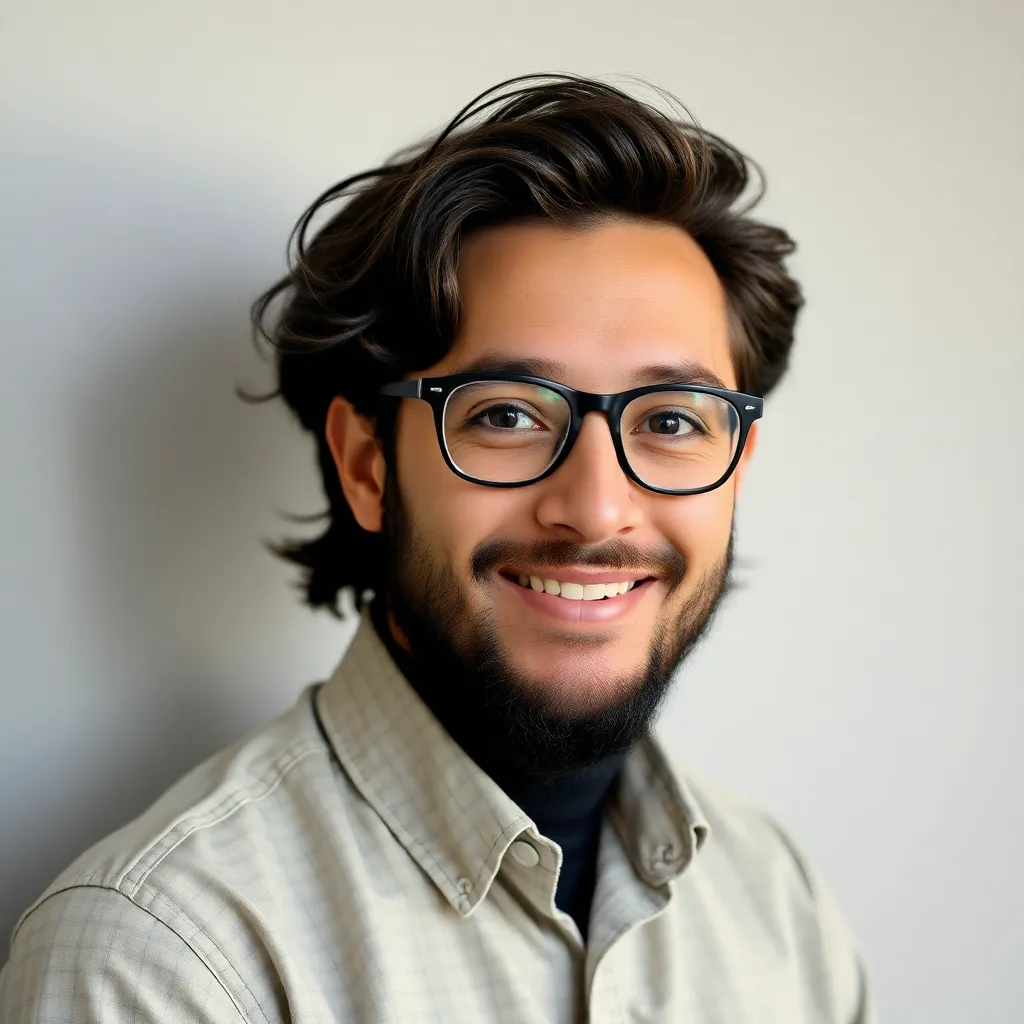
Holbox
Apr 04, 2025 · 6 min read

Table of Contents
- The Distribution Of Z Scores Is Always
- Table of Contents
- The Distribution of Z-Scores is Always… Standard Normal
- What is a Z-Score?
- The Standard Normal Distribution: A Deep Dive
- Why is the Distribution of Z-Scores Always Standard Normal?
- Applications of Z-Scores and the Standard Normal Distribution
- Limitations and Considerations
- Conclusion: The Ubiquity of the Standard Normal Distribution
- Latest Posts
- Latest Posts
- Related Post
The Distribution of Z-Scores is Always… Standard Normal
The distribution of z-scores is always a standard normal distribution. This seemingly simple statement underpins a vast array of statistical analyses and applications. Understanding why this is true, and the implications of this fact, is crucial for anyone working with statistical data. This comprehensive guide will delve into the properties of the standard normal distribution, explore how z-scores achieve this standardization, and demonstrate its practical applications across various fields.
What is a Z-Score?
A z-score, also known as a standard score, represents the number of standard deviations a data point lies from the mean of its distribution. It's a crucial tool for standardizing data, allowing for comparisons between datasets with different means and standard deviations. The formula for calculating a z-score is:
z = (x - μ) / σ
Where:
- x is the individual data point
- μ is the population mean
- σ is the population standard deviation
If you're working with a sample, you would substitute the sample mean (x̄) and sample standard deviation (s) for μ and σ respectively.
The Standard Normal Distribution: A Deep Dive
The standard normal distribution is a specific type of normal distribution with a mean (μ) of 0 and a standard deviation (σ) of 1. Its unique characteristics make it invaluable in statistical inference. Key properties include:
-
Symmetrical: The distribution is perfectly symmetrical around its mean of 0. This means that the probability of a z-score being a certain distance above the mean is equal to the probability of it being that same distance below the mean.
-
Bell-Shaped: It follows the classic bell curve shape, with the majority of data points clustered around the mean and fewer points further away.
-
Area Under the Curve: The total area under the curve is equal to 1, or 100%. This allows us to determine probabilities associated with specific z-scores or ranges of z-scores. This area is often calculated using z-tables or statistical software.
-
Empirical Rule (68-95-99.7 Rule): Approximately 68% of data points fall within one standard deviation of the mean, 95% within two standard deviations, and 99.7% within three standard deviations. This rule provides a quick approximation of probabilities in a standard normal distribution.
Why is the Distribution of Z-Scores Always Standard Normal?
The fundamental reason the distribution of z-scores is always standard normal lies in the z-score transformation itself. The formula linearly transforms any data point from its original distribution to a point on the standard normal distribution.
Let's consider a simple example. Suppose we have a dataset of student test scores with a mean of 75 and a standard deviation of 10. A student scoring 85 would have a z-score of:
z = (85 - 75) / 10 = 1
This means the student's score is one standard deviation above the mean. Regardless of the original distribution's shape, this transformation centers the data around 0 and scales it to have a standard deviation of 1. This process is consistent for every data point in the original dataset.
This transformation effectively:
-
Centers the data: Subtracting the mean (μ) shifts the distribution so that the new mean is 0.
-
Scales the data: Dividing by the standard deviation (σ) scales the distribution so that the new standard deviation is 1.
Consequently, the transformed data (the z-scores) always follows a standard normal distribution, regardless of the original distribution's shape (though the original distribution must have a mean and standard deviation).
Applications of Z-Scores and the Standard Normal Distribution
The ability to standardize data using z-scores and the resulting standard normal distribution has far-reaching implications across numerous fields. Here are some key applications:
-
Comparing Data from Different Distributions: Z-scores allow for direct comparison of data points from datasets with different units, means, and standard deviations. For example, comparing the performance of students on different exams with varying scoring systems.
-
Probability Calculations: The standard normal distribution's well-defined probabilities enable us to easily calculate the probability of observing a particular z-score or a range of z-scores. This is crucial for hypothesis testing and confidence intervals. Z-tables or statistical software packages like R or Python are commonly used for this purpose.
-
Hypothesis Testing: Many statistical hypothesis tests rely on the standard normal distribution. For example, z-tests are used to test hypotheses about population means when the population standard deviation is known or when the sample size is large.
-
Quality Control: In manufacturing and other industries, z-scores are used to monitor the quality of products. By tracking the z-scores of key quality metrics, manufacturers can identify deviations from expected values and take corrective actions.
-
Finance: Z-scores are used in financial modeling to assess risk and creditworthiness. For instance, the Altman Z-score is a commonly used financial model for predicting bankruptcy risk.
-
Medicine and Healthcare: Z-scores are used in various medical applications, such as identifying outliers in clinical trial data, evaluating patient characteristics, and developing diagnostic tools.
-
Social Sciences: In research involving social sciences, z-scores are invaluable in standardizing data across different samples and populations. This allows researchers to make meaningful comparisons across various demographic groups.
Limitations and Considerations
While z-scores and the standard normal distribution are powerful tools, it's crucial to acknowledge their limitations:
-
Assumptions of Normality: Many statistical tests using z-scores assume that the underlying data is normally distributed. If this assumption is violated, the results of these tests might be unreliable. Techniques like transformations or non-parametric tests can be used when dealing with non-normal data.
-
Outliers: Extreme values (outliers) in the dataset can heavily influence the mean and standard deviation, thus affecting the z-scores. Careful examination of the data for outliers is essential before calculating z-scores.
-
Sample Size: When working with samples instead of populations, the accuracy of z-scores depends on the sample size. Larger sample sizes generally provide more reliable estimates of the population parameters.
Conclusion: The Ubiquity of the Standard Normal Distribution
The distribution of z-scores is unequivocally always a standard normal distribution. This fundamental principle underpins a wide range of statistical techniques and has far-reaching applications in various scientific and practical fields. Understanding the properties of the standard normal distribution and the z-score transformation is essential for interpreting statistical results and making informed decisions based on data analysis. Whether analyzing student test scores, assessing financial risk, or evaluating medical data, the power and versatility of z-scores and the standard normal distribution remain indispensable tools in the world of statistics. By mastering this core concept, you gain a crucial foundation for understanding and applying a vast array of statistical methods. Remember to always consider the assumptions and limitations of the approach, and ensure the data appropriately supports the chosen methodology. This ensures the reliability and validity of any conclusions drawn.
Latest Posts
Latest Posts
-
Essentials Of Corporate Finance 11th Edition
Apr 07, 2025
-
Exercise 22 Review Sheet Art Labeling Activity 1
Apr 07, 2025
-
Illnesses Such As Diabetes And Cancer Kill More
Apr 07, 2025
-
Delta Company Produces A Single Product
Apr 07, 2025
-
Theory And Practice Of Counseling And Psychotherapy 11th Edition
Apr 07, 2025
Related Post
Thank you for visiting our website which covers about The Distribution Of Z Scores Is Always . We hope the information provided has been useful to you. Feel free to contact us if you have any questions or need further assistance. See you next time and don't miss to bookmark.