The Correlation Coefficient Is A Measure Of
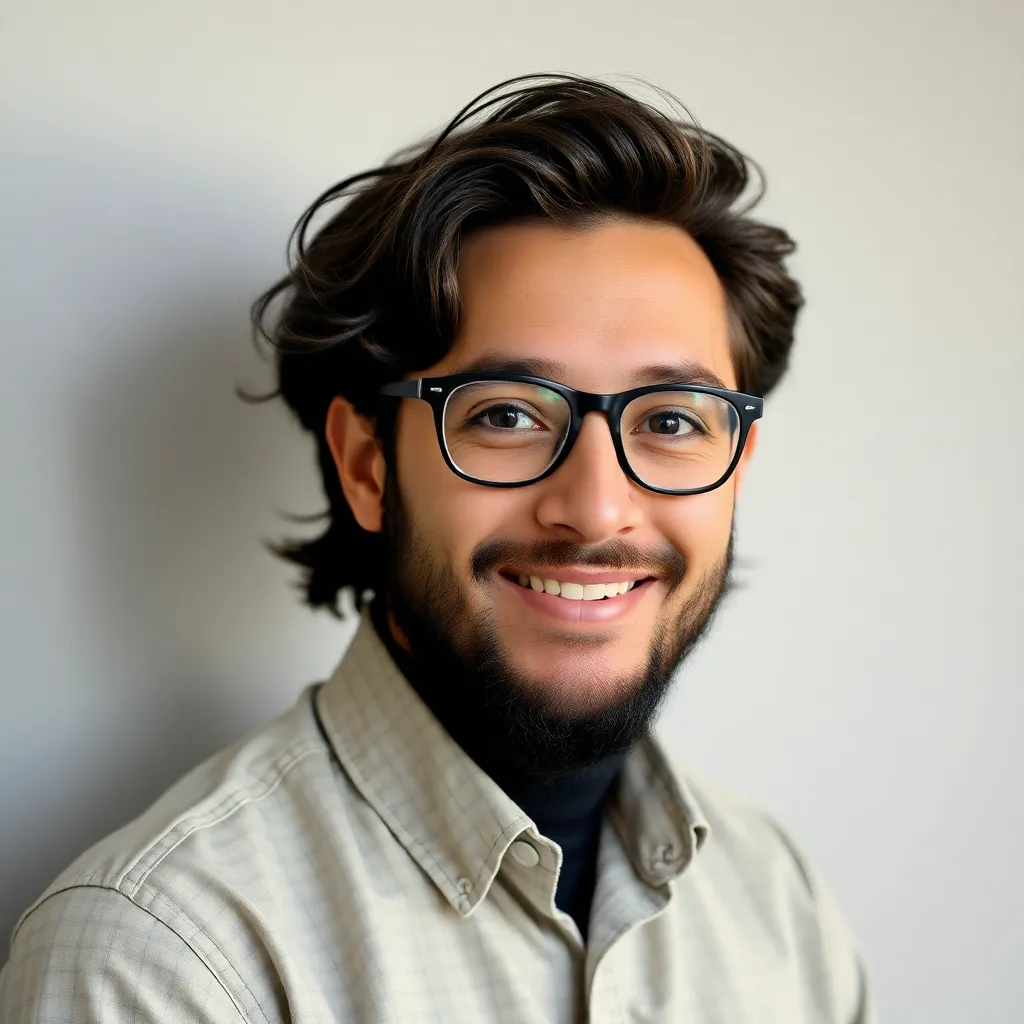
Holbox
May 12, 2025 · 6 min read
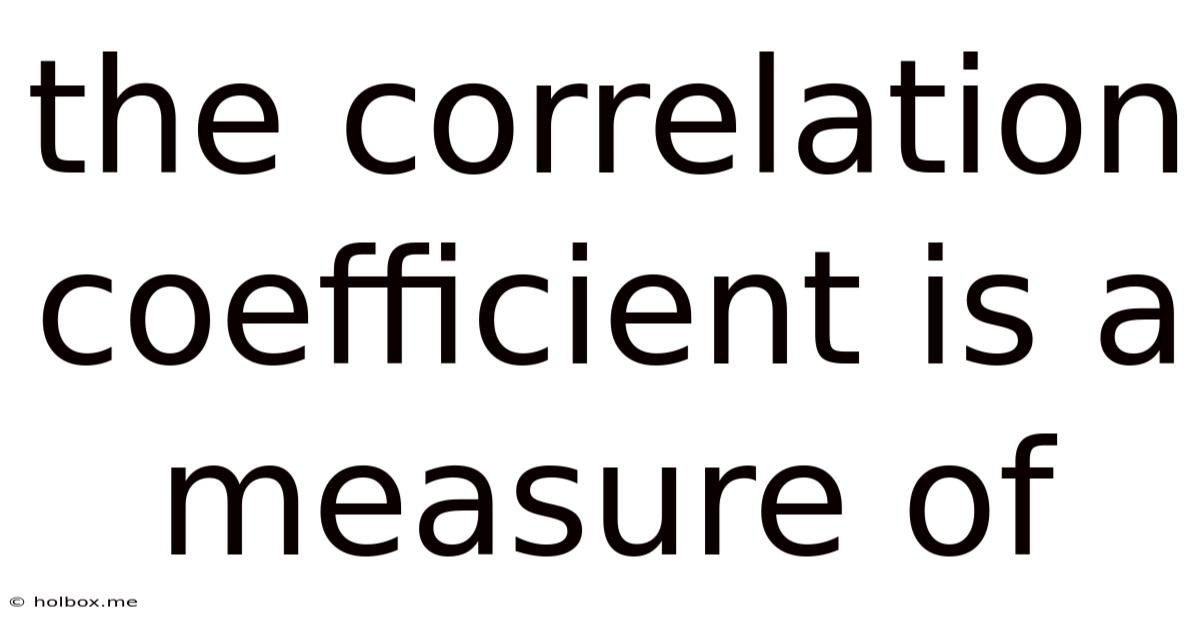
Table of Contents
- The Correlation Coefficient Is A Measure Of
- Table of Contents
- The Correlation Coefficient: A Measure of Association Between Variables
- What is a Correlation Coefficient?
- Visualizing Correlation
- Types of Correlation Coefficients
- 1. Pearson's Product-Moment Correlation Coefficient (Pearson's r)
- 2. Spearman's Rank Correlation Coefficient (Spearman's rho)
- 3. Kendall's Tau Correlation Coefficient (Kendall's tau)
- 4. Point-Biserial Correlation
- Interpreting the Correlation Coefficient
- Limitations of Correlation Coefficients
- Practical Applications of Correlation Coefficients
- Choosing the Right Correlation Coefficient
- Conclusion
- Latest Posts
- Latest Posts
- Related Post
The Correlation Coefficient: A Measure of Association Between Variables
The correlation coefficient is a fundamental statistical measure that quantifies the strength and direction of the linear relationship between two variables. Understanding its nuances is crucial for researchers across various disciplines, from social sciences and economics to engineering and medicine. This article delves deep into the concept of the correlation coefficient, exploring its different types, interpretations, limitations, and practical applications.
What is a Correlation Coefficient?
At its core, the correlation coefficient indicates how closely two variables move together. A positive correlation means that as one variable increases, the other tends to increase as well. Conversely, a negative correlation implies that as one variable increases, the other tends to decrease. A correlation coefficient of zero suggests no linear relationship between the variables, although other, non-linear relationships might still exist.
The most commonly used correlation coefficient is Pearson's r, which measures the linear association between two continuous variables. It ranges from -1 to +1, where:
- +1: Represents a perfect positive linear correlation.
- 0: Represents no linear correlation.
- -1: Represents a perfect negative linear correlation.
Values between these extremes indicate the strength of the correlation. For example, a correlation coefficient of +0.8 indicates a strong positive correlation, while a coefficient of -0.3 indicates a weak negative correlation.
Visualizing Correlation
Understanding correlation is often easier with visual aids. Scatter plots are invaluable tools for visualizing the relationship between two variables. A scatter plot with points clustered tightly around a positively sloped line suggests a strong positive correlation, while points clustered around a negatively sloped line suggest a strong negative correlation. A scattered distribution of points suggests a weak or no correlation.
Types of Correlation Coefficients
While Pearson's r is the most widely used, other correlation coefficients exist, each designed for specific data types and scenarios:
1. Pearson's Product-Moment Correlation Coefficient (Pearson's r)
As mentioned earlier, Pearson's r is suitable for measuring the linear relationship between two continuous variables. It assumes that the data is normally distributed and that the relationship is linear. Its formula involves calculating the covariance of the two variables and dividing it by the product of their standard deviations.
Strengths: Widely understood, widely used, robust for large datasets
Weaknesses: Assumes linearity, sensitive to outliers, not suitable for non-continuous data.
2. Spearman's Rank Correlation Coefficient (Spearman's rho)
Spearman's rho is a non-parametric measure of correlation that assesses the monotonic relationship between two variables. It's particularly useful when the data is ordinal (ranked) or when the assumptions of Pearson's r are violated. Spearman's rho ranks the data before calculating the correlation, making it less sensitive to outliers than Pearson's r.
Strengths: Non-parametric, less sensitive to outliers, applicable to ordinal data.
Weaknesses: Less powerful than Pearson's r if the relationship is linear, doesn't capture the strength of non-monotonic relationships.
3. Kendall's Tau Correlation Coefficient (Kendall's tau)
Similar to Spearman's rho, Kendall's tau is a non-parametric measure of correlation. However, it calculates the correlation by counting concordant and discordant pairs of observations. Concordant pairs are those where the ranks of both variables agree, while discordant pairs are those where the ranks disagree.
Strengths: Less sensitive to outliers than Pearson's r, robust against non-normal distributions.
Weaknesses: Less powerful than Pearson's r for large datasets with linear relationships, computationally intensive for very large datasets.
4. Point-Biserial Correlation
This correlation coefficient measures the association between a continuous variable and a dichotomous variable (a variable with only two categories). For example, it could measure the correlation between height (continuous) and gender (male/female).
Strengths: Specifically designed for continuous and dichotomous variables.
Weaknesses: Limited to this specific type of data comparison.
Interpreting the Correlation Coefficient
The numerical value of the correlation coefficient provides insights into the strength and direction of the relationship, but it's crucial to understand the limitations.
-
Strength of Correlation: The closer the absolute value of the correlation coefficient is to 1, the stronger the correlation. Common interpretations are:
- |r| < 0.3: Weak correlation
- 0.3 ≤ |r| < 0.5: Moderate correlation
- |r| ≥ 0.5: Strong correlation
-
Direction of Correlation: The sign of the correlation coefficient (+ or -) indicates the direction of the relationship.
-
Significance Testing: It's essential to test the statistical significance of the correlation coefficient to determine whether the observed correlation is likely due to chance or reflects a true relationship in the population. This is often done using a hypothesis test, which produces a p-value. A small p-value (typically less than 0.05) suggests that the correlation is statistically significant.
Limitations of Correlation Coefficients
While correlation coefficients are valuable tools, they have several limitations:
-
Correlation does not equal causation: A strong correlation between two variables doesn't necessarily mean that one causes the other. There could be a third, unobserved variable (a confounding variable) influencing both.
-
Linearity Assumption: Pearson's r assumes a linear relationship. If the relationship is non-linear, Pearson's r may not accurately reflect the association.
-
Sensitivity to Outliers: Outliers can significantly influence the correlation coefficient, especially with smaller datasets.
-
Spurious Correlations: Sometimes, correlations can appear statistically significant by pure chance, especially when many variables are examined.
-
Restricted Range: If the range of values for one or both variables is restricted, the correlation coefficient may underestimate the true correlation.
Practical Applications of Correlation Coefficients
Correlation coefficients find extensive applications in various fields:
-
Finance: Analyzing the relationship between asset prices, interest rates, and other economic indicators.
-
Medicine: Investigating the relationship between risk factors and disease outcomes.
-
Social Sciences: Examining the correlation between social and economic variables.
-
Engineering: Assessing the relationship between design parameters and performance characteristics.
-
Psychology: Studying the correlation between personality traits and behaviors.
-
Environmental Science: Analyzing the relationship between environmental factors and ecological outcomes.
Choosing the Right Correlation Coefficient
Selecting the appropriate correlation coefficient depends on the nature of the data and the research question. Consider the following factors:
- Data type: Are the variables continuous, ordinal, or dichotomous?
- Relationship type: Is the relationship expected to be linear or monotonic?
- Assumptions: Are the assumptions of normality and linearity met?
- Sample size: Larger sample sizes provide more reliable correlation estimates.
Conclusion
The correlation coefficient is a powerful statistical tool for quantifying the relationship between two variables. Understanding its different types, interpretations, and limitations is crucial for accurate and meaningful analysis. Researchers should carefully consider the nature of their data and research question when selecting the appropriate correlation coefficient and interpreting the results. Always remember that correlation does not imply causation, and further investigation is often needed to understand the underlying mechanisms driving the observed relationships. Combining correlation analysis with other statistical methods and domain expertise allows for a more comprehensive understanding of the complexities of relationships between variables. By mastering the principles and nuances of correlation analysis, researchers can extract valuable insights from their data and make more informed decisions.
Latest Posts
Latest Posts
-
300 Seconds Is How Many Minutes
May 18, 2025
-
How Many Ounces Are In 14 Grams
May 18, 2025
-
How Many Ounces Is 1 14 Litres
May 18, 2025
-
102 Grams Is How Many Ounces
May 18, 2025
-
How Many Oz In 300 Grams
May 18, 2025
Related Post
Thank you for visiting our website which covers about The Correlation Coefficient Is A Measure Of . We hope the information provided has been useful to you. Feel free to contact us if you have any questions or need further assistance. See you next time and don't miss to bookmark.