Statistics For Business And Economics Chapter 7 Solutions
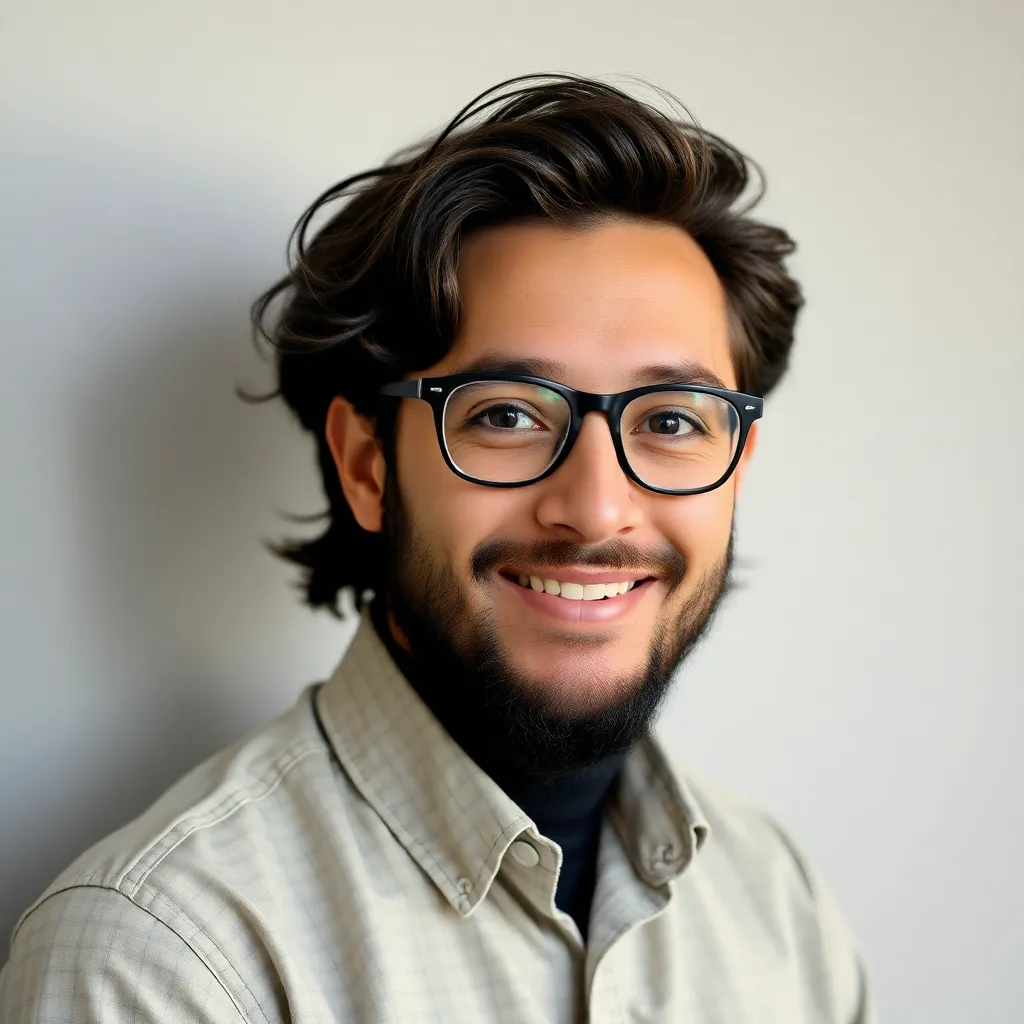
Holbox
May 08, 2025 · 8 min read
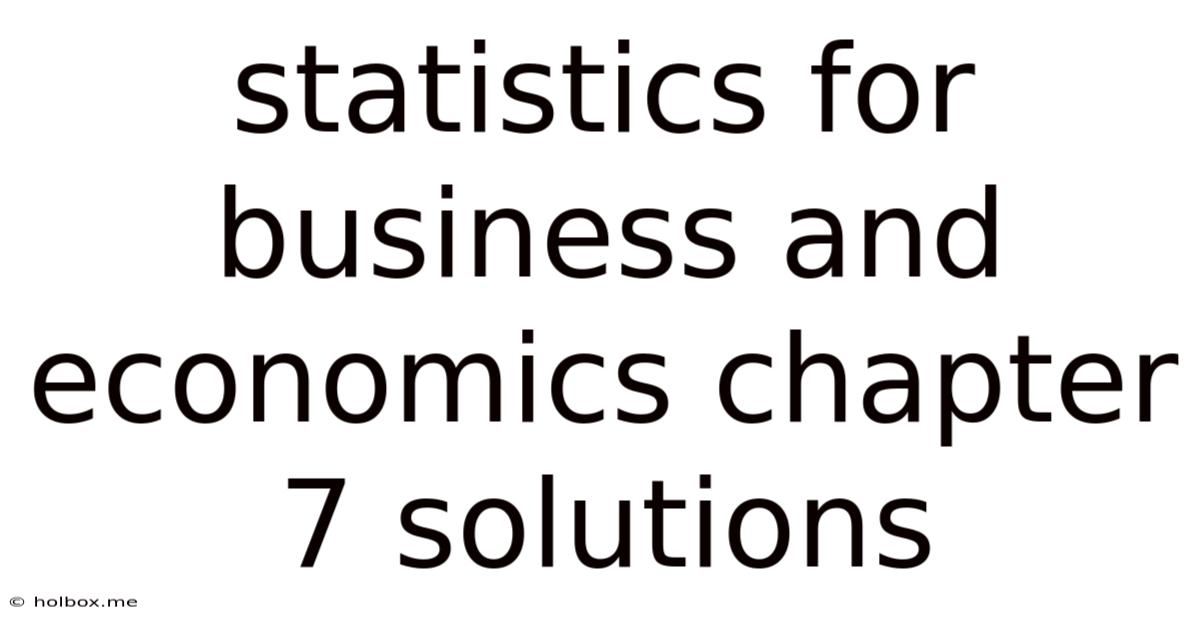
Table of Contents
- Statistics For Business And Economics Chapter 7 Solutions
- Table of Contents
- Statistics for Business and Economics: Chapter 7 Solutions – A Comprehensive Guide
- Understanding Hypothesis Testing: The Foundation
- 1. Formulating Hypotheses:
- 2. Selecting the Appropriate Test Statistic:
- 3. Setting the Significance Level (α):
- 4. Calculating the Test Statistic and p-value:
- 5. Making a Decision:
- Common Chapter 7 Problem Types and Solutions
- One-Sample t-test:
- Two-Sample t-test (Independent Samples):
- Paired t-test:
- ANOVA (Analysis of Variance):
- Chi-Square Test:
- Conclusion
- Latest Posts
- Related Post
Statistics for Business and Economics: Chapter 7 Solutions – A Comprehensive Guide
Chapter 7 of most business statistics textbooks typically covers hypothesis testing, a crucial concept for making informed decisions based on data. This guide provides comprehensive solutions and explanations for common problems encountered in this chapter, helping you master the core principles of hypothesis testing and their applications in business and economics. We'll explore various types of tests, their assumptions, and how to interpret the results. Remember, consulting your specific textbook is crucial for referencing the exact problem statements and datasets.
Understanding Hypothesis Testing: The Foundation
Before diving into specific solutions, let's review the fundamental steps involved in hypothesis testing:
1. Formulating Hypotheses:
This involves stating the null hypothesis (H₀) and the alternative hypothesis (H₁ or Hₐ). The null hypothesis represents the status quo or a claim you're trying to disprove. The alternative hypothesis is what you believe to be true if you reject the null hypothesis. These hypotheses should be clearly defined and mutually exclusive.
- Example: Testing whether the average income of a specific demographic is greater than $50,000.
- H₀: μ ≤ $50,000 (The average income is less than or equal to $50,000)
- H₁: μ > $50,000 (The average income is greater than $50,000)
2. Selecting the Appropriate Test Statistic:
The choice of test statistic depends on several factors:
- Type of data: Is your data continuous or categorical?
- Sample size: Is your sample large enough to assume normality?
- Number of groups: Are you comparing means between two groups, more than two groups, or testing a single mean against a known value?
Common test statistics include:
- Z-test: Used for large samples (typically n ≥ 30) when the population standard deviation is known or for proportions.
- t-test: Used for smaller samples when the population standard deviation is unknown. There are different variations: one-sample t-test, two-sample t-test (independent or paired), and ANOVA (Analysis of Variance) for comparing means across multiple groups.
- Chi-square test: Used for analyzing categorical data and testing for independence or goodness-of-fit.
3. Setting the Significance Level (α):
The significance level, typically set at 0.05 (5%), represents the probability of rejecting the null hypothesis when it's actually true (Type I error). A lower significance level reduces the chance of a Type I error but increases the chance of a Type II error (failing to reject a false null hypothesis).
4. Calculating the Test Statistic and p-value:
This involves using the appropriate formula for your chosen test statistic and calculating the p-value. The p-value is the probability of observing the obtained results (or more extreme results) if the null hypothesis is true.
5. Making a Decision:
- If the p-value is less than or equal to the significance level (α): Reject the null hypothesis. There is sufficient evidence to support the alternative hypothesis.
- If the p-value is greater than the significance level (α): Fail to reject the null hypothesis. There is not enough evidence to support the alternative hypothesis.
Common Chapter 7 Problem Types and Solutions
Let's address some typical problems found in Chapter 7, categorized by test type. Remember to always check your textbook for specific problem details and data.
One-Sample t-test:
Problem Example: A company claims that its new light bulb lasts an average of 1000 hours. A sample of 50 bulbs is tested, and the average lifespan is found to be 980 hours with a standard deviation of 80 hours. Test the company's claim at a 5% significance level.
Solution:
-
Hypotheses:
- H₀: μ = 1000 hours
- H₁: μ ≠ 1000 hours (two-tailed test)
-
Test Statistic: One-sample t-test because the population standard deviation is unknown.
-
Significance Level: α = 0.05
-
Calculation: Using the t-test formula, we calculate the t-statistic. This involves calculating the sample mean, standard error, and then the t-statistic. The degrees of freedom (df) are n-1 = 49. Consult a t-table or statistical software to find the critical t-value for a two-tailed test with 49 degrees of freedom and α = 0.05. Then compare your calculated t-statistic to this critical value. You can also calculate the p-value using statistical software.
-
Decision: If the absolute value of the calculated t-statistic is greater than the critical t-value (or if the p-value is less than 0.05), we reject the null hypothesis. Otherwise, we fail to reject it.
Two-Sample t-test (Independent Samples):
Problem Example: Two different marketing campaigns are tested. Campaign A results in 100 sales with a standard deviation of 15, while Campaign B results in 120 sales with a standard deviation of 20. Test if there is a significant difference in sales between the two campaigns at a 1% significance level. Assume the sample sizes are sufficiently large to justify a t-test.
Solution:
-
Hypotheses:
- H₀: μ₁ = μ₂ (There is no difference in sales)
- H₁: μ₁ ≠ μ₂ (There is a difference in sales)
-
Test Statistic: Two-sample independent t-test.
-
Significance Level: α = 0.01
-
Calculation: The calculation involves calculating the pooled standard deviation (since we are assuming equal variances), the standard error of the difference in means, and then the t-statistic. Degrees of freedom will be calculated using the formula appropriate for the two-sample t-test. Compare the calculated t-statistic with the critical t-value (from a t-table or software) or calculate the p-value.
-
Decision: Based on the comparison of the t-statistic and the critical value or the p-value, we decide whether to reject or fail to reject the null hypothesis.
Paired t-test:
Problem Example: A company wants to test the effectiveness of a new training program. They measure employee productivity before and after the training. Test if the training program significantly improves productivity at a 0.05 significance level.
Solution:
-
Hypotheses:
- H₀: μd = 0 (No difference in productivity)
- H₁: μd > 0 (Productivity increased after training) – This is a one-tailed test because we expect an increase.
-
Test Statistic: Paired t-test because we are comparing the same subjects before and after the intervention.
-
Significance Level: α = 0.05
-
Calculation: The paired t-test works on the differences between the paired observations. Calculate the mean and standard deviation of these differences. Use these values to calculate the t-statistic and compare it to the critical t-value or the p-value.
-
Decision: Make a decision based on the comparison.
ANOVA (Analysis of Variance):
Problem Example: Three different fertilizers are tested on the yield of a particular crop. Compare the average yields for each fertilizer to determine if there are significant differences at a 0.01 significance level.
Solution:
-
Hypotheses:
- H₀: μ₁ = μ₂ = μ₃ (No difference in average yields)
- H₁: At least one mean is different
-
Test Statistic: ANOVA F-test
-
Significance Level: α = 0.01
-
Calculation: ANOVA involves calculating the between-group variance and the within-group variance (error variance). The F-statistic is the ratio of these variances. The degrees of freedom are calculated for both the numerator (between groups) and the denominator (within groups). Compare the calculated F-statistic to the critical F-value (obtained from an F-table or statistical software) or examine the p-value.
-
Decision: If the calculated F-statistic exceeds the critical F-value (or if the p-value is less than 0.01), we reject the null hypothesis. This indicates that at least one fertilizer has a significantly different average yield compared to the others. Post-hoc tests (like Tukey's HSD) might be necessary to determine which specific groups differ.
Chi-Square Test:
Problem Example: A company surveys customers about their satisfaction with a product, categorizing responses as "Satisfied," "Neutral," and "Dissatisfied." Test if there is an association between customer satisfaction and gender (male/female) at a 0.05 significance level.
Solution:
-
Hypotheses:
- H₀: There is no association between satisfaction and gender.
- H₁: There is an association between satisfaction and gender.
-
Test Statistic: Chi-square test of independence.
-
Significance Level: α = 0.05
-
Calculation: Create a contingency table showing the observed frequencies for each combination of satisfaction level and gender. Calculate the expected frequencies under the assumption of independence. Use these values to calculate the chi-square statistic. The degrees of freedom are (number of rows - 1) * (number of columns - 1).
-
Decision: Compare the calculated chi-square statistic to the critical chi-square value (obtained from a chi-square table or software) or examine the p-value. If the calculated value exceeds the critical value (or if the p-value is less than 0.05), we reject the null hypothesis indicating an association between satisfaction and gender.
Conclusion
Mastering hypothesis testing is vital for making data-driven decisions in business and economics. This guide provides a framework for solving various problems encountered in Chapter 7. Remember to consult your textbook for specific problem statements, data, and any unique instructions. Using statistical software will significantly aid in the calculations and interpretation of results. Understanding the underlying principles and the nuances of each test will enable you to confidently analyze data and draw meaningful conclusions. Always remember to clearly state your hypotheses, choose the correct test, interpret the results correctly in context, and communicate your findings effectively.
Latest Posts
Related Post
Thank you for visiting our website which covers about Statistics For Business And Economics Chapter 7 Solutions . We hope the information provided has been useful to you. Feel free to contact us if you have any questions or need further assistance. See you next time and don't miss to bookmark.