Statistics For Business And Economics 7th Edition Benson
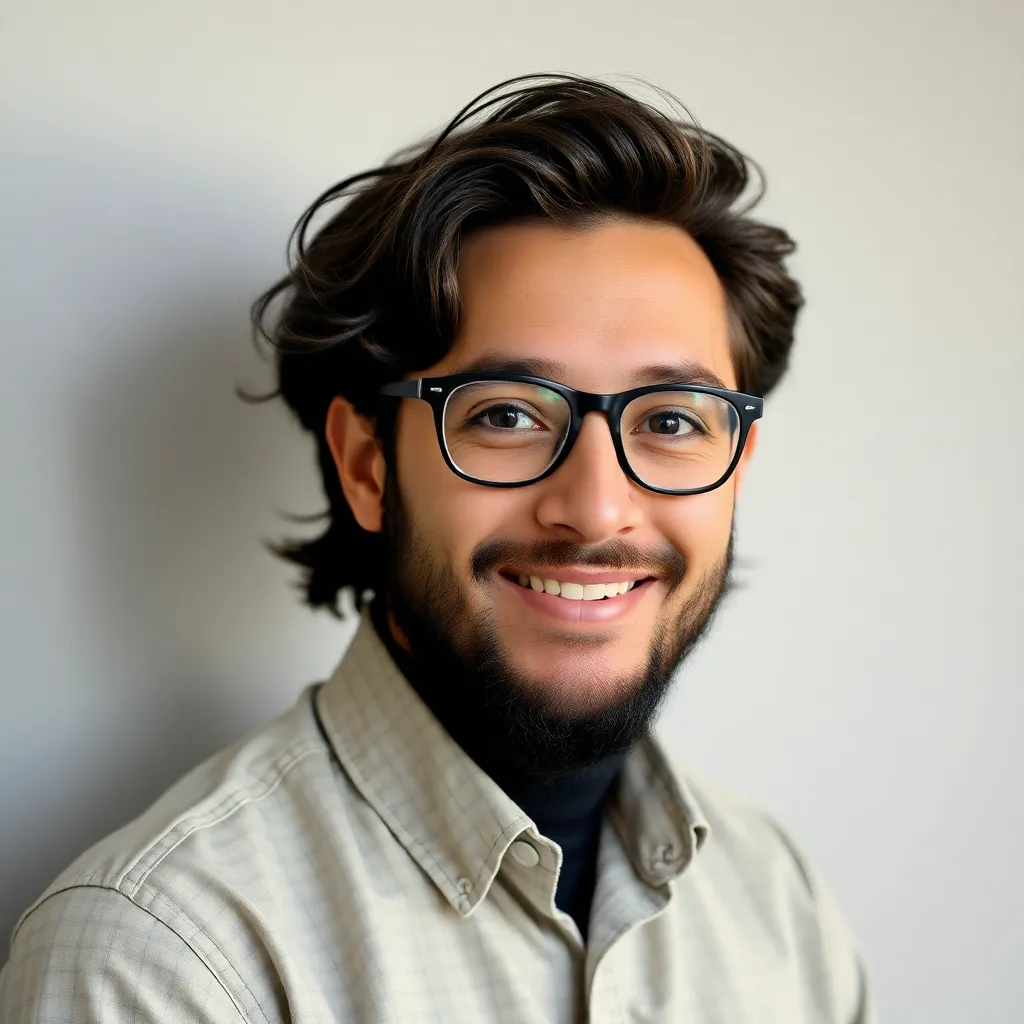
Holbox
Apr 06, 2025 · 7 min read

Table of Contents
- Statistics For Business And Economics 7th Edition Benson
- Table of Contents
- Statistics for Business and Economics, 7th Edition: A Comprehensive Guide
- Understanding Descriptive Statistics: Organizing and Summarizing Data
- Measures of Central Tendency
- Measures of Dispersion
- Data Visualization
- Inferential Statistics: Making Inferences from Sample Data
- Probability Distributions
- Estimation
- Hypothesis Testing
- Regression Analysis: Modeling Relationships Between Variables
- Simple Linear Regression
- Multiple Linear Regression
- Time Series Analysis: Analyzing Data Over Time
- Non-Parametric Methods
- Case Studies and Applications
- Software and Tools
- Conclusion
- Latest Posts
- Latest Posts
- Related Post
Statistics for Business and Economics, 7th Edition: A Comprehensive Guide
Statistics for Business and Economics, 7th Edition by Benson et al. provides a robust introduction to statistical methods relevant to business and economic contexts. This comprehensive guide equips students with the skills to analyze data, interpret results, and make informed decisions. This article delves into the key concepts covered in the book, providing a detailed overview and practical applications.
Understanding Descriptive Statistics: Organizing and Summarizing Data
The foundation of any statistical analysis lies in effectively organizing and summarizing data. Benson's text begins by clearly explaining descriptive statistics, which involves methods for presenting data in a meaningful way. Key elements discussed include:
Measures of Central Tendency
This section focuses on understanding the "center" of a dataset. The book thoroughly covers:
- Mean: The average of all data points, calculated by summing all values and dividing by the number of observations. The text likely emphasizes the limitations of the mean when dealing with skewed data or outliers.
- Median: The middle value when data is arranged in ascending order. This is particularly useful when dealing with outliers, as it's less sensitive to extreme values than the mean.
- Mode: The most frequently occurring value in a dataset. The book probably explains situations where multiple modes might exist.
Measures of Dispersion
Understanding the spread or variability of data is crucial. Key concepts likely covered include:
- Range: The difference between the highest and lowest values. A simple measure, but sensitive to outliers.
- Variance: The average of the squared differences from the mean. This provides a measure of how spread out the data is.
- Standard Deviation: The square root of the variance. This is often preferred to variance because it's expressed in the same units as the original data, making it more easily interpretable.
- Interquartile Range (IQR): The difference between the 75th percentile (third quartile) and the 25th percentile (first quartile). This is a robust measure of dispersion, less sensitive to outliers than the range.
Data Visualization
The book likely emphasizes the importance of visualizing data for effective communication and insight. Techniques covered probably include:
- Histograms: Graphical representations of the frequency distribution of a continuous variable.
- Frequency Distributions: Tabular representations of the frequency of different values or ranges of values.
- Bar Charts: Used to display categorical data, showing the frequency or proportion of each category.
- Pie Charts: Used to show the proportion of different categories within a whole.
- Box Plots: Displaying the median, quartiles, and potential outliers in a dataset. These are particularly useful for comparing distributions.
Inferential Statistics: Making Inferences from Sample Data
Benson's text moves beyond descriptive statistics to cover inferential statistics, which focuses on drawing conclusions about a population based on a sample of data. This section is likely crucial and covers several key areas:
Probability Distributions
A firm understanding of probability distributions is essential for inferential statistics. The book likely covers:
- Discrete Probability Distributions: Such as the binomial and Poisson distributions, used for modeling discrete variables.
- Continuous Probability Distributions: Such as the normal distribution, essential for many statistical tests and confidence intervals.
- The Central Limit Theorem: A fundamental concept explaining why the sampling distribution of the mean tends to be normally distributed, regardless of the population distribution, given a sufficiently large sample size.
Estimation
This section likely focuses on estimating population parameters from sample statistics. Key concepts probably include:
- Point Estimation: Providing a single value as an estimate of a population parameter (e.g., using the sample mean to estimate the population mean).
- Interval Estimation: Constructing a range of values (confidence interval) within which the population parameter is likely to fall, with a specified level of confidence. The book likely explains the concept of margin of error.
Hypothesis Testing
Hypothesis testing is a crucial component of inferential statistics, allowing us to test claims about population parameters. The text likely covers various tests including:
- One-Sample t-test: Used to test whether a sample mean differs significantly from a hypothesized population mean.
- Two-Sample t-test: Used to compare the means of two independent samples.
- Paired t-test: Used to compare the means of two related samples (e.g., before and after measurements).
- Chi-Square Test: Used to test for associations between categorical variables.
- ANOVA (Analysis of Variance): Used to compare the means of three or more groups.
The book will likely stress the importance of setting up null and alternative hypotheses, determining significance levels (alpha), calculating p-values, and interpreting the results in the context of the research question. It should also cover Type I and Type II errors.
Regression Analysis: Modeling Relationships Between Variables
Regression analysis is a powerful tool for modeling relationships between a dependent variable and one or more independent variables. Benson's text likely covers:
Simple Linear Regression
This involves modeling the relationship between one dependent variable and one independent variable using a straight line. The book probably explains:
- Estimating the Regression Equation: Finding the best-fitting line through the data using the method of least squares.
- Interpreting Coefficients: Understanding the meaning of the slope and intercept in the context of the variables.
- Assessing the Goodness of Fit: Measuring how well the model fits the data using measures like R-squared.
- Hypothesis Testing for Coefficients: Testing whether the slope is significantly different from zero.
Multiple Linear Regression
This extends simple linear regression to include multiple independent variables. The text will likely explain:
- Model Building: Selecting relevant independent variables and assessing their impact on the dependent variable.
- Multicollinearity: The problem of high correlation between independent variables and how to address it.
- Model Diagnostics: Assessing the assumptions of the model and identifying potential problems.
Time Series Analysis: Analyzing Data Over Time
For economic and business data, time series analysis is crucial. The book likely includes:
- Trend Analysis: Identifying long-term patterns in data.
- Seasonal Decomposition: Separating seasonal variations from underlying trends.
- Forecasting: Using time series models to predict future values.
Non-Parametric Methods
Benson's text may include an introduction to non-parametric methods, which are statistical techniques that don't rely on assumptions about the distribution of the data. These are particularly useful when dealing with data that is not normally distributed. Examples may include:
- Sign Test: A simple test for comparing two groups when data is ordinal or has unknown distribution.
- Mann-Whitney U Test: A non-parametric alternative to the two-sample t-test.
- Wilcoxon Signed Rank Test: A non-parametric alternative to the paired t-test.
- Spearman's Rank Correlation: A non-parametric measure of association between two variables.
Case Studies and Applications
A strong textbook like Benson's will likely include numerous case studies and real-world examples illustrating the application of statistical methods in business and economic settings. These examples are crucial for solidifying understanding and showcasing the practical relevance of the concepts.
Software and Tools
While the book itself won't contain software, it likely recommends statistical software packages that students can use to perform the analyses discussed. Popular choices include SPSS, SAS, R, and Stata. Understanding how to utilize these tools effectively is crucial for practical application of statistical methods.
Conclusion
Statistics for Business and Economics, 7th Edition by Benson et al., provides a comprehensive and accessible introduction to statistical methods relevant to business and economic decision-making. By covering descriptive and inferential statistics, regression analysis, and time series analysis, the book equips students with the skills needed to analyze data, interpret results, and make informed decisions in a variety of contexts. The inclusion of case studies, real-world examples, and likely recommendations for statistical software further enhances the learning experience, ensuring that students are well-prepared to apply their statistical knowledge to practical problems. The book likely serves as a strong foundation for further studies in econometrics, business analytics, and other quantitative fields. The focus on clear explanations, practical applications, and likely the inclusion of numerous exercises will aid in students gaining a thorough understanding of the subject matter.
Latest Posts
Latest Posts
-
Which Of The Following Statements Is The Most Accurate
Apr 14, 2025
-
7 70 Draw The Shear And Moment Diagrams For The Beam
Apr 14, 2025
-
All Of The Following Are Property Plant And Equipment Except
Apr 14, 2025
-
Which Of The Following Is An Organic Molecule
Apr 14, 2025
-
Choose All That Are Characteristics Of Neuroglia
Apr 14, 2025
Related Post
Thank you for visiting our website which covers about Statistics For Business And Economics 7th Edition Benson . We hope the information provided has been useful to you. Feel free to contact us if you have any questions or need further assistance. See you next time and don't miss to bookmark.