Simulation Based And Exact One Porpotion Inference Rossman
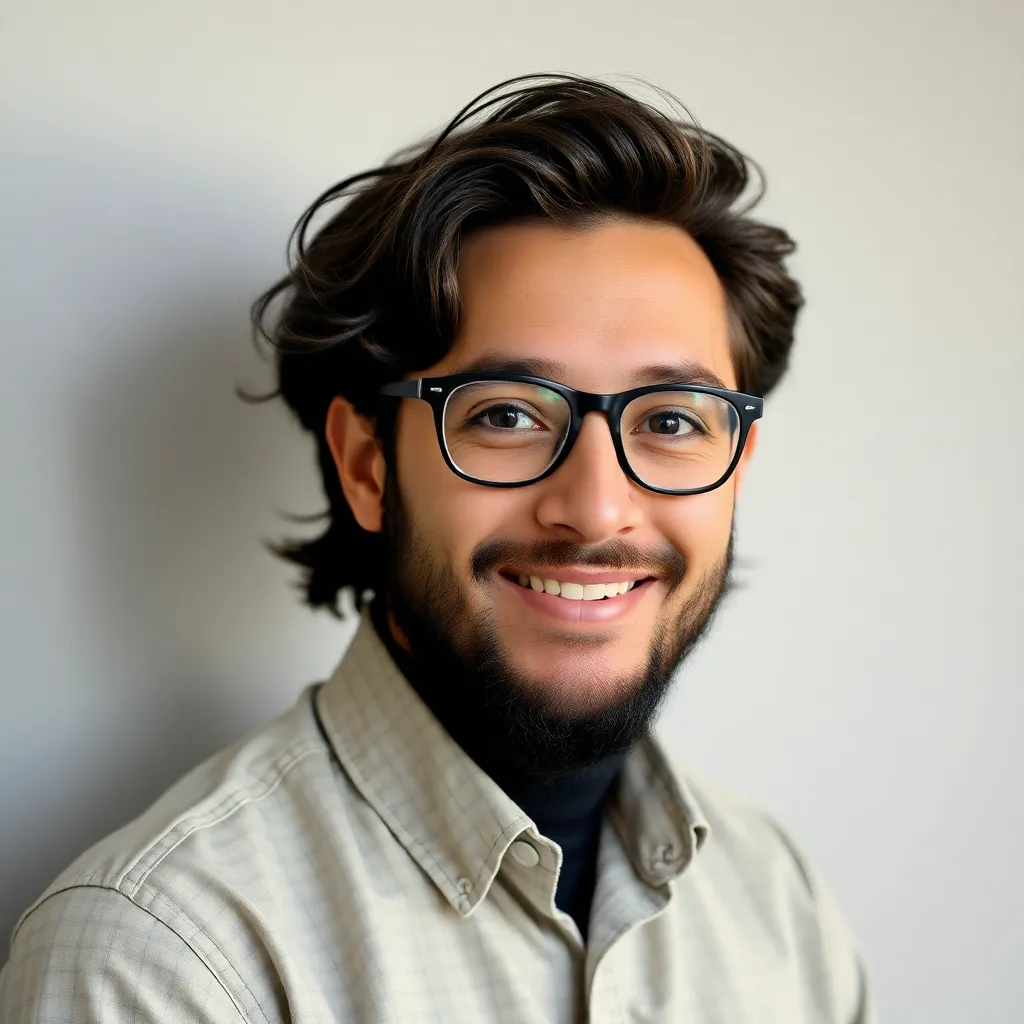
Holbox
May 08, 2025 · 6 min read
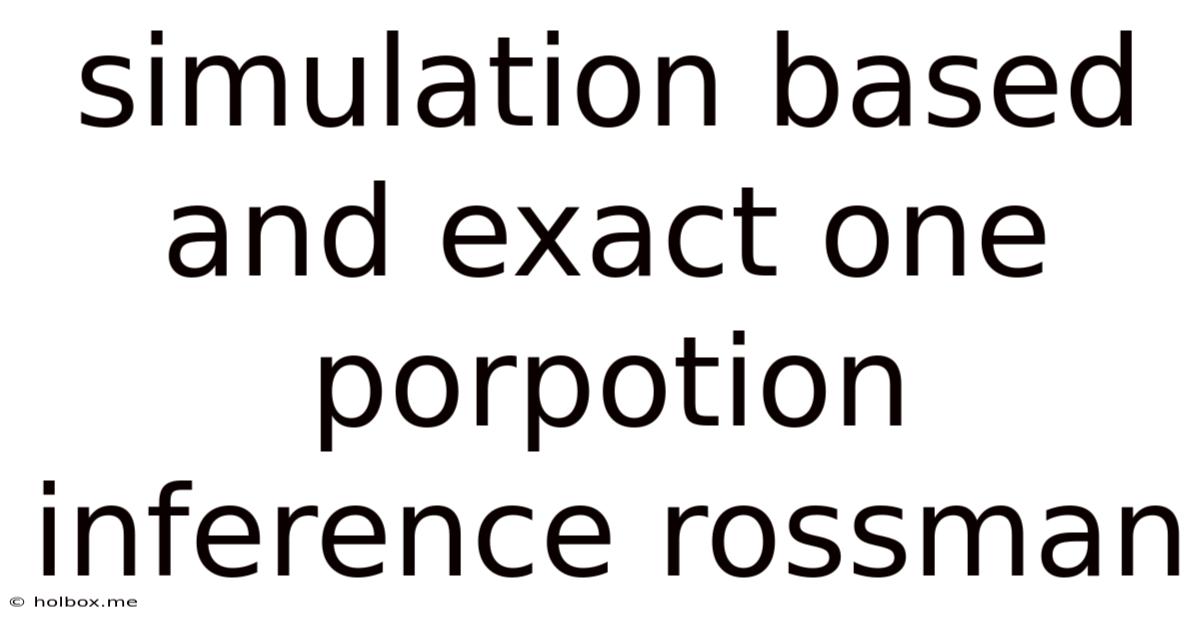
Table of Contents
- Simulation Based And Exact One Porpotion Inference Rossman
- Table of Contents
- Simulation-Based and Exact One-Proportion Inference: A Deep Dive into Rossman's Approach
- Understanding One-Proportion Inference
- Simulation-Based Inference: The Power of Randomization
- The Process of Simulation-Based Inference:
- Advantages of Simulation-Based Inference:
- Limitations of Simulation-Based Inference:
- Exact Inference: The Binomial Distribution
- Performing Exact Inference:
- Advantages of Exact Inference:
- Limitations of Exact Inference:
- Comparing Simulation-Based and Exact Inference
- Practical Applications and Examples
- Conclusion
- Latest Posts
- Latest Posts
- Related Post
Simulation-Based and Exact One-Proportion Inference: A Deep Dive into Rossman's Approach
Rossman's approach to one-proportion inference offers a powerful and insightful alternative to traditional methods. This method, emphasizing simulation-based inference alongside exact methods, provides a deeper understanding of statistical concepts and allows for more robust analysis, especially when dealing with small sample sizes or situations where assumptions of traditional methods are violated. This comprehensive article delves into the core principles of Rossman's approach, exploring both simulation-based and exact methods for one-proportion inference, highlighting their strengths and limitations, and providing practical examples.
Understanding One-Proportion Inference
One-proportion inference deals with situations where we want to make inferences about a population proportion (p) based on a sample proportion (p̂). This is a common problem in various fields, from public health (estimating the prevalence of a disease) to marketing (determining the effectiveness of an advertising campaign). The central question often revolves around testing a hypothesis about the population proportion or constructing a confidence interval to estimate its value.
Traditional methods, often relying on the normal approximation, require certain conditions to be met: a sufficiently large sample size (typically np ≥ 10 and n(1-p) ≥ 10, where n is the sample size) and data that follows a binomial distribution. Rossman's approach, however, offers flexibility and a more intuitive understanding, particularly when these assumptions are not fully satisfied.
Simulation-Based Inference: The Power of Randomization
Simulation-based inference utilizes the power of random sampling to estimate the sampling distribution of the sample proportion. Instead of relying on theoretical distributions, we simulate numerous random samples from a hypothetical population with a specified proportion (often the null hypothesis value). This allows us to observe the distribution of sample proportions under the null hypothesis and assess the plausibility of observing the obtained sample proportion.
The Process of Simulation-Based Inference:
-
Specify the Null Hypothesis: Start by formulating a null hypothesis about the population proportion (e.g., H₀: p = 0.5).
-
Simulate Random Samples: Generate a large number (e.g., 10,000) of random samples from a binomial distribution with the specified null hypothesis proportion and sample size. Each simulated sample yields a sample proportion (p̂).
-
Create a Distribution of Sample Proportions: Organize the simulated sample proportions into a histogram or other visual representation. This forms an empirical sampling distribution under the null hypothesis.
-
Assess the Observed Sample Proportion: Compare the observed sample proportion from the actual data to the simulated sampling distribution. If the observed proportion falls within a region of the simulated distribution considered typical (e.g., within the central 95%), we fail to reject the null hypothesis. Otherwise, we reject the null hypothesis.
-
Calculate a p-value: The proportion of simulated sample proportions as or more extreme than the observed sample proportion provides an estimate of the p-value. A smaller p-value indicates stronger evidence against the null hypothesis.
Advantages of Simulation-Based Inference:
- Intuitive Understanding: The visual representation of the sampling distribution enhances understanding of the statistical process.
- Flexibility: It does not rely on strict assumptions of normality or large sample sizes. This makes it applicable in scenarios where traditional methods are less reliable.
- Handles Small Sample Sizes: Effectively performs inference even with small sample sizes, where the normal approximation might be inadequate.
- Adaptability: Easily adaptable to various hypothesis testing scenarios and confidence interval construction.
Limitations of Simulation-Based Inference:
- Computational Resources: Requires computational power, especially for a very large number of simulations.
- Randomness: The results will vary slightly depending on the random seed used in the simulation.
Exact Inference: The Binomial Distribution
Exact inference for one-proportion relies on the binomial distribution itself. This method is particularly valuable when the sample size is small, making the normal approximation unreliable. The exact method calculates probabilities directly using the binomial probability mass function.
Performing Exact Inference:
-
Specify the Null Hypothesis and Significance Level: Similar to the simulation method, we start with a null hypothesis and a chosen significance level (e.g., α = 0.05).
-
Calculate Probabilities: Use the binomial probability mass function to calculate the probability of observing the obtained sample proportion (or a more extreme result) under the null hypothesis. This probability represents the exact p-value.
-
Decision Based on p-value: Compare the exact p-value to the chosen significance level. If the p-value is less than or equal to the significance level, we reject the null hypothesis; otherwise, we fail to reject it.
Advantages of Exact Inference:
- Accuracy: Provides the most accurate p-value calculation, especially for small sample sizes.
- No Approximations: Avoids the approximations inherent in normal approximations.
- Precise Results: Yields precise results without relying on asymptotic properties.
Limitations of Exact Inference:
- Computationally Intensive: Can be computationally intensive for large sample sizes.
- Limited to Binomial Data: Directly applicable only to data following a binomial distribution.
Comparing Simulation-Based and Exact Inference
Both simulation-based and exact methods offer advantages for one-proportion inference. The choice between them often depends on the sample size and computational resources.
Feature | Simulation-Based Inference | Exact Inference |
---|---|---|
Sample Size | Works well for both large and small sample sizes | Particularly advantageous for small sample sizes |
Assumptions | Less reliant on strict assumptions | Relies on binomial distribution |
Computational Cost | Can be computationally intensive for very large simulations | Can be computationally intensive for large sample sizes |
Accuracy | Approximate p-value | Exact p-value |
Intuition | Highly intuitive due to visual representation | Less intuitive, requires understanding of binomial distribution |
Practical Applications and Examples
Let's consider a scenario: A researcher wants to determine if a new drug is effective in reducing symptoms. In a sample of 50 patients, 35 experience symptom reduction. The null hypothesis is that the drug has no effect (p = 0.5).
Simulation-Based Approach: We'd simulate thousands of samples of size 50 from a binomial distribution with p = 0.5. We'd then compare the observed proportion (35/50 = 0.7) to this simulated distribution. The proportion of simulated proportions greater than or equal to 0.7 would provide the estimated p-value.
Exact Approach: We'd use the binomial probability mass function to calculate the probability of observing 35 or more successes in 50 trials, given p = 0.5. This would give us the exact p-value.
Conclusion
Rossman's approach, integrating simulation-based and exact methods, provides a versatile and insightful framework for one-proportion inference. The simulation approach fosters a deeper understanding of sampling variability and is particularly useful for small samples and situations where assumptions of traditional methods are violated. The exact approach provides precise p-values, especially for smaller samples where the binomial distribution is a more accurate model. The choice between these methods depends on the specific context, sample size, and computational resources available. By understanding both methods, researchers can select the most appropriate technique for their specific inference problem, leading to more robust and reliable conclusions. The emphasis on visual representation and intuitive understanding within Rossman's framework makes it an invaluable tool for teaching and practicing statistical inference.
Latest Posts
Latest Posts
-
150 Km Per Hour In Miles
May 20, 2025
-
69 Inches To Feet And Inches
May 20, 2025
-
60 Days Is How Many Weeks
May 20, 2025
-
How Many Weeks Are In 40 Days
May 20, 2025
-
What Is 33 Feet In Meters
May 20, 2025
Related Post
Thank you for visiting our website which covers about Simulation Based And Exact One Porpotion Inference Rossman . We hope the information provided has been useful to you. Feel free to contact us if you have any questions or need further assistance. See you next time and don't miss to bookmark.