Putting Forecast Errors Into Perspective Is Best Done Using
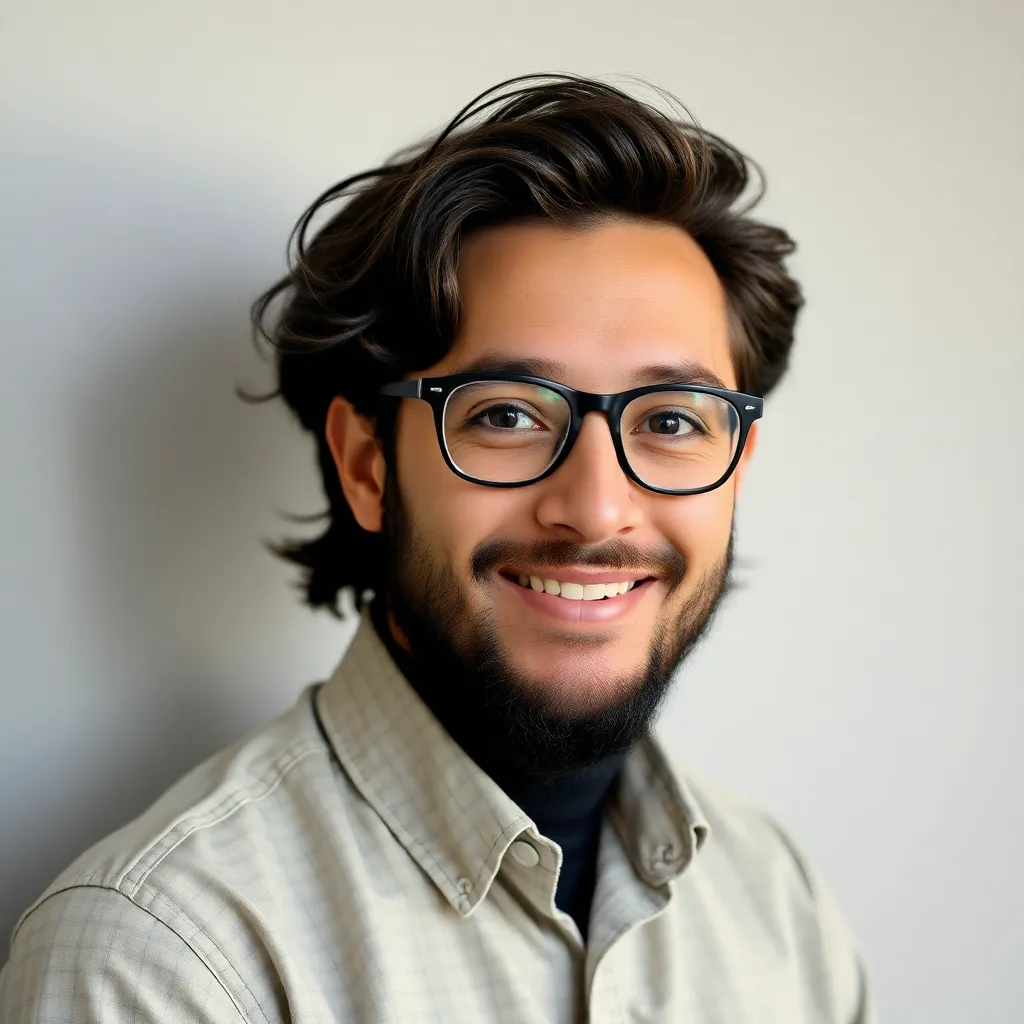
Holbox
May 08, 2025 · 6 min read
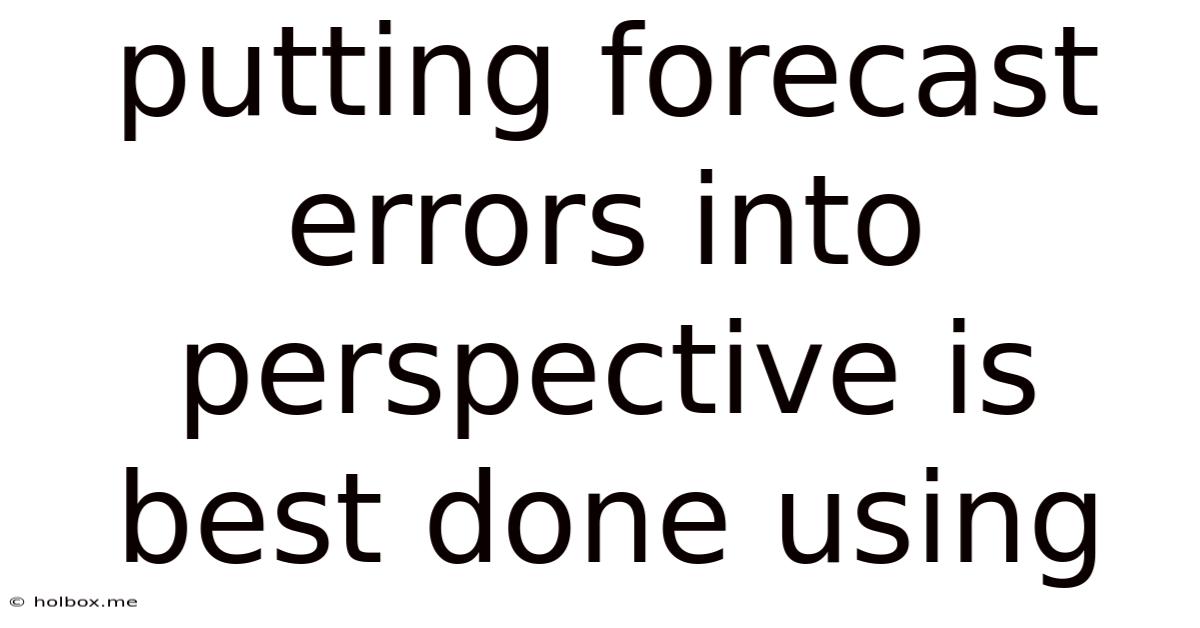
Table of Contents
- Putting Forecast Errors Into Perspective Is Best Done Using
- Table of Contents
- Putting Forecast Errors into Perspective: A Deep Dive into Effective Methods
- Understanding the Nature of Forecast Errors
- 1. Data limitations:
- 2. Model limitations:
- 3. External factors:
- Effective Methods for Putting Forecast Errors into Perspective
- 1. Visualizing Errors:
- 2. Quantifying Errors:
- 3. Understanding Error Decomposition:
- 4. Using Confidence Intervals:
- 5. Comparing Forecasts:
- 6. Communicating Uncertainty Effectively:
- Advanced Techniques for Error Analysis
- Putting it all together: A Practical Example
- Conclusion
- Latest Posts
- Related Post
Putting Forecast Errors into Perspective: A Deep Dive into Effective Methods
Forecasting, whether it's predicting sales figures, weather patterns, or stock prices, is an inherently uncertain endeavor. No forecast is perfect; errors are inevitable. The key isn't to eliminate errors entirely – that's practically impossible – but to understand them, quantify them, and effectively manage their impact. This article delves into the best methods for putting forecast errors into perspective, equipping you with the tools to make more informed decisions based on your predictions.
Understanding the Nature of Forecast Errors
Before we discuss how to put forecast errors into perspective, let's first define what they are and why they occur. Forecast errors represent the difference between the predicted value and the actual observed value. These errors can stem from a multitude of sources, including:
1. Data limitations:
- Incomplete data: Lack of sufficient historical data can lead to inaccurate models and projections. The more data you have, generally, the better your forecast will be, but even vast datasets can contain biases.
- Poor data quality: Inaccurate, inconsistent, or outdated data will inevitably result in flawed forecasts. Data cleaning and validation are crucial steps in the forecasting process.
- Data bias: Historical data may not accurately reflect future trends, especially in rapidly changing environments. For example, a forecast based on pre-pandemic sales data would likely be inaccurate when applied to the post-pandemic landscape.
2. Model limitations:
- Model selection: Choosing the wrong forecasting model for the data can significantly impact accuracy. The optimal model depends on factors such as data characteristics, forecasting horizon, and desired level of detail.
- Parameter estimation: Even with the right model, inaccurate parameter estimation can lead to significant errors. This involves determining the optimal values for the model's variables, which often requires iterative refinement.
- Model overfitting: A model that is too complex and overfits the training data may perform poorly on unseen data. Simplicity and generalizability are crucial for robust forecasts.
3. External factors:
- Unforeseen events: Unexpected events, such as natural disasters, economic crises, or political upheavals, can significantly disrupt forecasts. These "black swan" events are difficult, if not impossible, to predict accurately.
- Changes in market dynamics: Shifts in consumer behavior, competition, or technology can render forecasts obsolete. Regularly updating your models and assumptions is critical in dynamic environments.
- Human error: Mistakes in data entry, model implementation, or interpretation can all contribute to forecast errors. Careful attention to detail and robust quality control procedures are essential.
Effective Methods for Putting Forecast Errors into Perspective
Now that we understand the sources of forecast errors, let's explore effective methods for analyzing and contextualizing them:
1. Visualizing Errors:
Graphical representations provide powerful insights into forecast error patterns. Key visualizations include:
- Time series plots: Plotting the forecast alongside the actual values reveals trends in error magnitude and direction. This visual representation helps identify periods of high or low accuracy.
- Histograms and density plots: These illustrate the distribution of forecast errors, revealing whether errors are normally distributed, skewed, or exhibit other patterns. Understanding the error distribution is crucial for probability assessments.
- Scatter plots: Plotting forecast errors against independent variables (e.g., time, seasonality, economic indicators) can highlight potential correlations and reveal systematic biases.
2. Quantifying Errors:
Numerical measures provide a concise summary of forecast accuracy:
- Mean Absolute Error (MAE): The average absolute difference between the predicted and actual values. Provides a straightforward measure of average error magnitude.
- Root Mean Squared Error (RMSE): The square root of the average squared difference between predicted and actual values. Penalizes larger errors more heavily than MAE.
- Mean Absolute Percentage Error (MAPE): The average absolute percentage difference between predicted and actual values. Useful for comparing forecasts across different scales.
- Weighted error metrics: Assign different weights to errors based on their importance or impact. For example, errors in peak demand periods might be weighted more heavily than errors during off-peak periods.
3. Understanding Error Decomposition:
Analyzing the sources of error can provide valuable insights for model improvement. This involves breaking down the total error into:
- Bias: The systematic tendency of a forecast to overestimate or underestimate the actual value. A high bias indicates a systematic problem with the model or data.
- Variance: The variability of the forecast errors around the mean. High variance suggests the model is sensitive to small changes in input data.
- Random error: The unpredictable component of the forecast error that cannot be attributed to bias or variance.
4. Using Confidence Intervals:
Instead of focusing solely on a point estimate, present forecasts as ranges of plausible values. Confidence intervals provide a probabilistic assessment of the forecast accuracy. For example, a 95% confidence interval indicates that there is a 95% chance that the actual value will fall within the specified range.
5. Comparing Forecasts:
Benchmarking your forecast against alternative methods or simpler models helps to assess its performance and identify areas for improvement. This can involve comparing different forecasting models, incorporating expert judgment, or using naive forecasting methods as a baseline.
6. Communicating Uncertainty Effectively:
Clearly communicating the limitations of your forecast is crucial for responsible decision-making. This involves:
- Transparency: Openly discuss the assumptions, limitations, and potential sources of error in your forecast.
- Contextualization: Present the forecast within the broader context of the problem, emphasizing the uncertainty inherent in the prediction.
- Sensitivity analysis: Explore how changes in key assumptions impact the forecast, highlighting the range of possible outcomes.
Advanced Techniques for Error Analysis
For more sophisticated error analysis, consider employing advanced statistical techniques:
- Time series decomposition: Separating the time series data into its components (trend, seasonality, cyclical, irregular) helps isolate sources of error and refine the forecasting model.
- Autoregressive Integrated Moving Average (ARIMA) models: Powerful time series models that can capture complex patterns and dependencies in the data.
- Exponential Smoothing methods: Adapt to changing trends and incorporate recent data with more weight.
- Machine learning algorithms: Advanced techniques like neural networks can capture complex non-linear relationships but require careful tuning and validation.
Putting it all together: A Practical Example
Let's imagine you're forecasting monthly sales for a new product. You've developed a model and generated forecasts for the next six months. To put your forecast errors into perspective, you would:
- Gather actual sales data: Once the months pass, collect the actual sales figures.
- Calculate error metrics: Compute MAE, RMSE, and MAPE to quantify the overall accuracy.
- Visualize errors: Create time series plots, histograms, and scatter plots to identify patterns and trends in the errors.
- Decompose errors: Analyze the bias, variance, and random error components to pinpoint areas for improvement.
- Communicate results: Present the forecast with confidence intervals and clearly state the assumptions and limitations of your model.
By systematically analyzing errors, you can continually refine your forecasting process, leading to more accurate predictions and improved decision-making.
Conclusion
Putting forecast errors into perspective is a crucial aspect of effective forecasting. It’s not about eliminating errors entirely, but about understanding their nature, quantifying their impact, and using that knowledge to improve your forecasting process and ultimately, your decision-making. By employing the methods outlined in this article, you can gain valuable insights, refine your models, and communicate your predictions with greater clarity and confidence. Remember that continuous monitoring, evaluation, and adaptation are key to successful forecasting in any domain. The process is iterative; each forecast provides valuable learning for the next.
Latest Posts
Related Post
Thank you for visiting our website which covers about Putting Forecast Errors Into Perspective Is Best Done Using . We hope the information provided has been useful to you. Feel free to contact us if you have any questions or need further assistance. See you next time and don't miss to bookmark.