Probability Is The Likelihood That An Outcome Occurs. True False
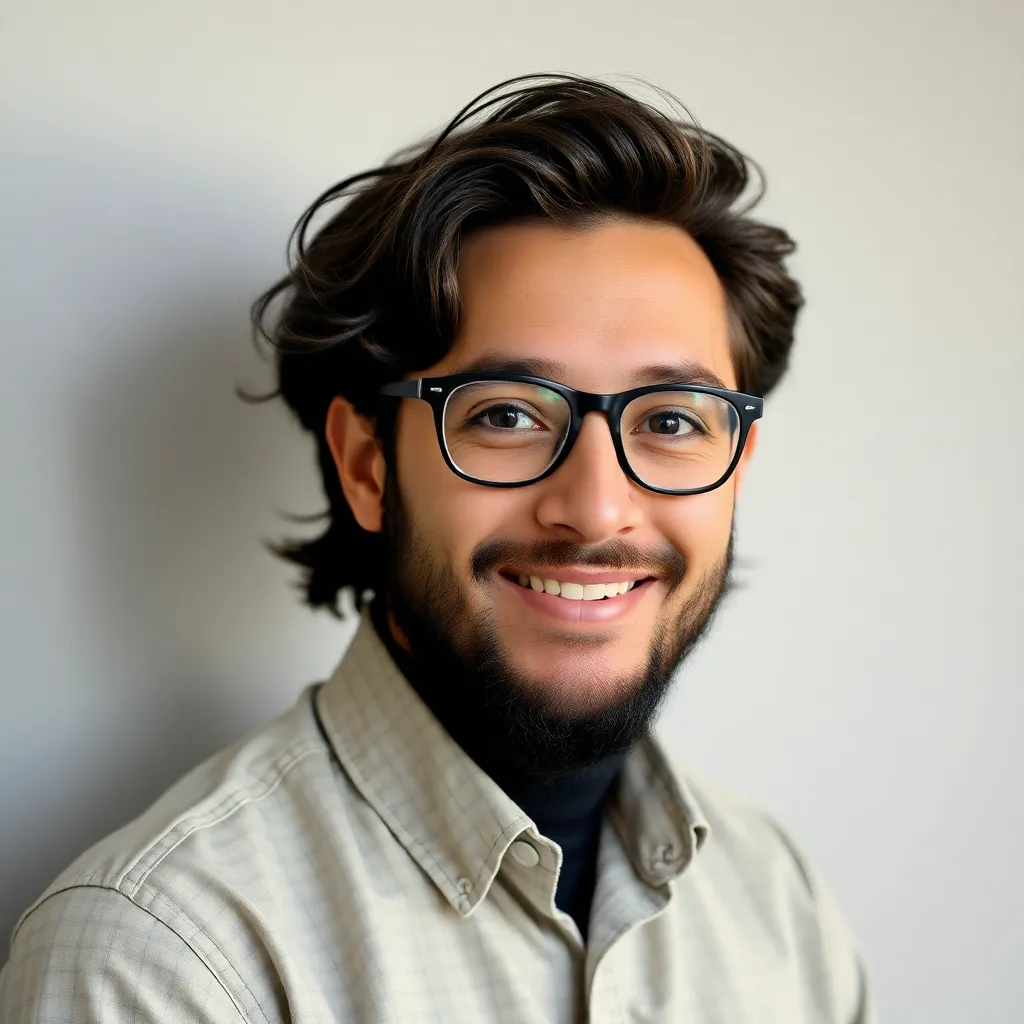
Holbox
Apr 26, 2025 · 7 min read
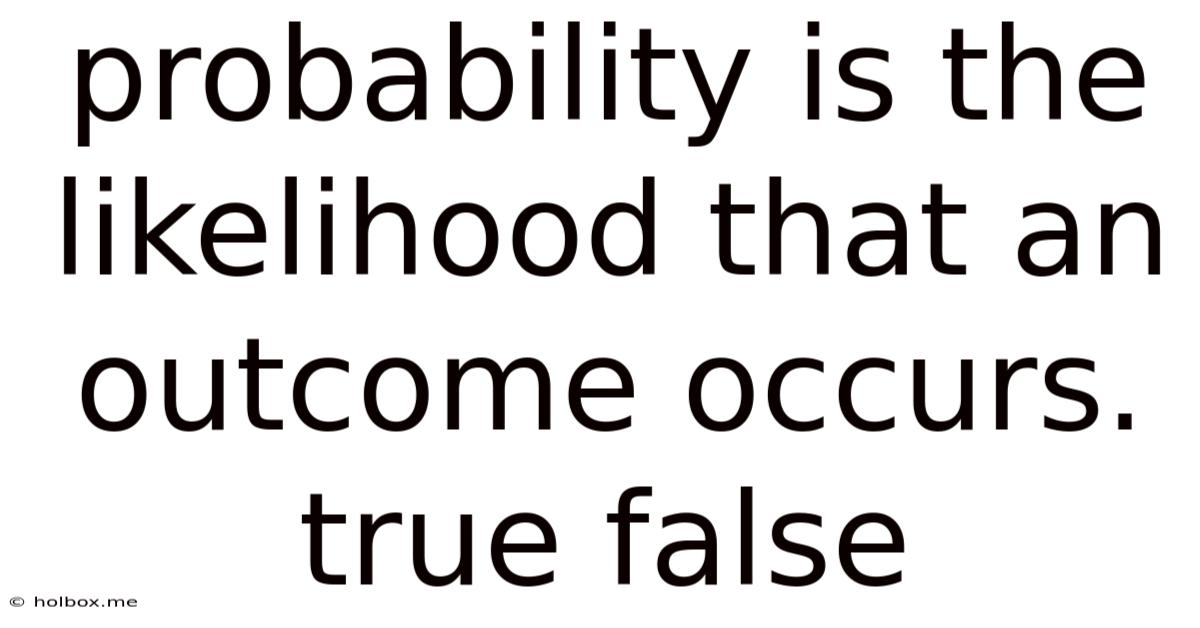
Table of Contents
- Probability Is The Likelihood That An Outcome Occurs. True False
- Table of Contents
- Probability: Is It Simply the Likelihood of an Outcome? (True, with Nuances)
- Understanding Probability: Beyond Simple Likelihood
- Defining Probability: A Formal Approach
- Different Interpretations of Probability
- 1. Frequentist Probability: The Relative Frequency Approach
- 2. Bayesian Probability: Incorporating Prior Knowledge
- 3. Classical Probability: Equally Likely Outcomes
- 4. Propensity Probability: Inherent Likelihood
- Randomness and Probability
- Beyond Basic Probability: Advanced Concepts
- 1. Conditional Probability: Probability Given Prior Information
- 2. Joint Probability: Probability of Multiple Events
- 3. Independent Events: Events with No Influence
- 4. Dependent Events: Interrelated Events
- 5. Probability Distributions: Describing Random Variables
- 6. Expected Value: The Average Outcome
- 7. Variance and Standard Deviation: Measuring Spread
- 8. Central Limit Theorem: The Power of Large Samples
- Applications of Probability Theory
- Conclusion: Probability - More Than Just Likelihood
- Latest Posts
- Latest Posts
- Related Post
Probability: Is It Simply the Likelihood of an Outcome? (True, with Nuances)
The statement "Probability is the likelihood that an outcome occurs" is largely true, but requires significant nuance to fully capture the richness and complexity of probability theory. While it provides a basic understanding, it overlooks crucial aspects like randomness, sample spaces, different probability interpretations, and the mathematical framework that underpins this seemingly simple concept. Let's delve deeper.
Understanding Probability: Beyond Simple Likelihood
The intuitive notion of probability – the chance of something happening – is indeed at the heart of the matter. However, formal probability theory goes far beyond this intuitive understanding. It provides a rigorous mathematical framework for quantifying and analyzing uncertainty. This framework allows us to move beyond simple estimations of likelihood and delve into precise calculations and predictions.
Defining Probability: A Formal Approach
In mathematical terms, probability is a number between 0 and 1 (inclusive), assigned to an event. An event is a specific outcome or a set of outcomes within a larger sample space. The sample space encompasses all possible outcomes of a given experiment or process. A probability of 0 means the event is impossible; a probability of 1 means the event is certain. Probabilities between 0 and 1 represent varying degrees of likelihood.
For instance, consider flipping a fair coin. The sample space consists of two possible outcomes: heads (H) and tails (T). The probability of getting heads is 0.5 (or 50%), and the probability of getting tails is also 0.5. This is based on the assumption of a fair coin, where both outcomes are equally likely.
Different Interpretations of Probability
The seemingly straightforward concept of probability actually encompasses various interpretations, each with its own strengths and limitations:
1. Frequentist Probability: The Relative Frequency Approach
This is the most common interpretation, particularly in scientific applications. Frequentist probability defines the probability of an event as the limit of its relative frequency in a large number of repeated trials. For example, if we flip a coin 1000 times and get heads 503 times, the relative frequency of heads is 503/1000 = 0.503. As the number of trials approaches infinity, this relative frequency is believed to converge towards the true probability of getting heads.
Strengths: Objective and based on observable data.
Limitations: Requires repeatable experiments; doesn't address events that cannot be repeated (e.g., the probability of a specific historical event).
2. Bayesian Probability: Incorporating Prior Knowledge
Bayesian probability incorporates prior knowledge or beliefs about an event into the calculation of its probability. This is done using Bayes' theorem, which updates the probability based on new evidence. For instance, if we have prior knowledge about a coin being biased (e.g., we suspect it lands on heads more often), this information is incorporated into our probability calculation. The Bayesian approach allows for subjective probabilities reflecting our beliefs, which can then be refined through data.
Strengths: Incorporates prior knowledge, useful for situations with limited data.
Limitations: Subjectivity in assigning prior probabilities can introduce bias.
3. Classical Probability: Equally Likely Outcomes
This is the simplest interpretation, applicable when all outcomes in the sample space are equally likely. The probability of an event is then calculated as the ratio of favorable outcomes to the total number of possible outcomes. For example, the probability of rolling a 6 on a fair six-sided die is 1/6.
Strengths: Simple and intuitive, applicable to many games of chance.
Limitations: Restrictive; requires the assumption of equally likely outcomes, which may not always hold true in real-world situations.
4. Propensity Probability: Inherent Likelihood
This interpretation views probability as the inherent propensity or tendency of an event to occur. It suggests that each event has an inherent probability, regardless of whether it is repeated or observed. This is a more philosophical interpretation and less focused on empirical observation.
Strengths: Addresses events that cannot be repeated.
Limitations: Difficult to quantify or measure directly.
Randomness and Probability
Probability inherently deals with randomness. Randomness implies that the outcome of an event cannot be predicted with certainty, even if we have complete knowledge of the underlying process. This doesn't mean that random events are chaotic or without pattern; rather, it means that the outcome is uncertain before the event occurs. Probability provides a framework for quantifying and understanding this uncertainty. True randomness, however, is a complex concept and is often debated, particularly in the context of generating random numbers for simulations and other applications. Truly random processes are quite difficult to achieve in practice.
Beyond Basic Probability: Advanced Concepts
The basic definition of probability as likelihood only scratches the surface. A deep understanding requires exploring various advanced concepts:
1. Conditional Probability: Probability Given Prior Information
Conditional probability deals with the probability of an event occurring given that another event has already occurred. This is denoted as P(A|B), representing the probability of event A occurring given that event B has occurred. Bayes' theorem is a crucial tool for calculating conditional probabilities.
2. Joint Probability: Probability of Multiple Events
Joint probability refers to the probability of two or more events occurring simultaneously. This is denoted as P(A and B), or P(A ∩ B).
3. Independent Events: Events with No Influence
Two events are independent if the occurrence of one does not affect the probability of the other. For example, flipping a coin twice; the outcome of the first flip doesn't influence the outcome of the second.
4. Dependent Events: Interrelated Events
In contrast, dependent events are those where the occurrence of one event influences the probability of the other. For instance, drawing two cards from a deck without replacement; the probability of the second card depends on the first card drawn.
5. Probability Distributions: Describing Random Variables
A probability distribution describes the likelihood of different outcomes for a random variable. Random variables are variables whose values are numerical outcomes of random phenomena. Common distributions include the normal (Gaussian) distribution, binomial distribution, Poisson distribution, and many others, each suited for different types of random processes.
6. Expected Value: The Average Outcome
The expected value is the average outcome of a random variable, weighted by its probability distribution. It provides a measure of the central tendency of a random variable.
7. Variance and Standard Deviation: Measuring Spread
Variance and standard deviation quantify the spread or dispersion of a probability distribution around its expected value. A high variance indicates a greater degree of uncertainty.
8. Central Limit Theorem: The Power of Large Samples
The central limit theorem is a fundamental result in probability theory, stating that the average of a large number of independent random variables, regardless of their individual distributions, tends towards a normal distribution. This theorem has immense practical applications in statistics and inference.
Applications of Probability Theory
Probability theory underpins countless fields:
-
Statistics: Probability is fundamental to statistical inference, hypothesis testing, and estimation.
-
Machine Learning: Machine learning algorithms heavily rely on probabilistic models for classification, prediction, and decision-making.
-
Finance: Probability is crucial for risk assessment, portfolio management, and option pricing.
-
Physics: Probability plays a vital role in quantum mechanics, describing the behavior of subatomic particles.
-
Engineering: Probability is used for reliability analysis, quality control, and risk management in engineering design.
-
Medicine: Probability is used in epidemiological studies, clinical trials, and diagnostic testing.
Conclusion: Probability - More Than Just Likelihood
In summary, the statement "Probability is the likelihood that an outcome occurs" is a good starting point, but an incomplete representation of this complex and powerful field. Probability theory provides a robust mathematical framework for quantifying uncertainty, encompassing various interpretations, advanced concepts, and widespread applications across diverse disciplines. While the intuitive notion of likelihood is crucial, a thorough understanding necessitates delving into the nuances of randomness, different probability interpretations, and the sophisticated mathematical tools used for analysis and prediction. This deeper exploration reveals the true scope and importance of probability in our world.
Latest Posts
Latest Posts
-
Calculate The Current I2 Flowing In Emf Source E2
May 08, 2025
-
The Teacher Said That You Were Correct
May 08, 2025
-
Music Business Handbook And Career Guide 13th Edition Pdf
May 08, 2025
-
A Company Truck Can Carry 1500
May 08, 2025
-
Davis Advantage For Townsends Essentials Of Psychiatric Mental Health Nursing
May 08, 2025
Related Post
Thank you for visiting our website which covers about Probability Is The Likelihood That An Outcome Occurs. True False . We hope the information provided has been useful to you. Feel free to contact us if you have any questions or need further assistance. See you next time and don't miss to bookmark.