Pre-trained Multi Task Generative Ai Models Are Called:
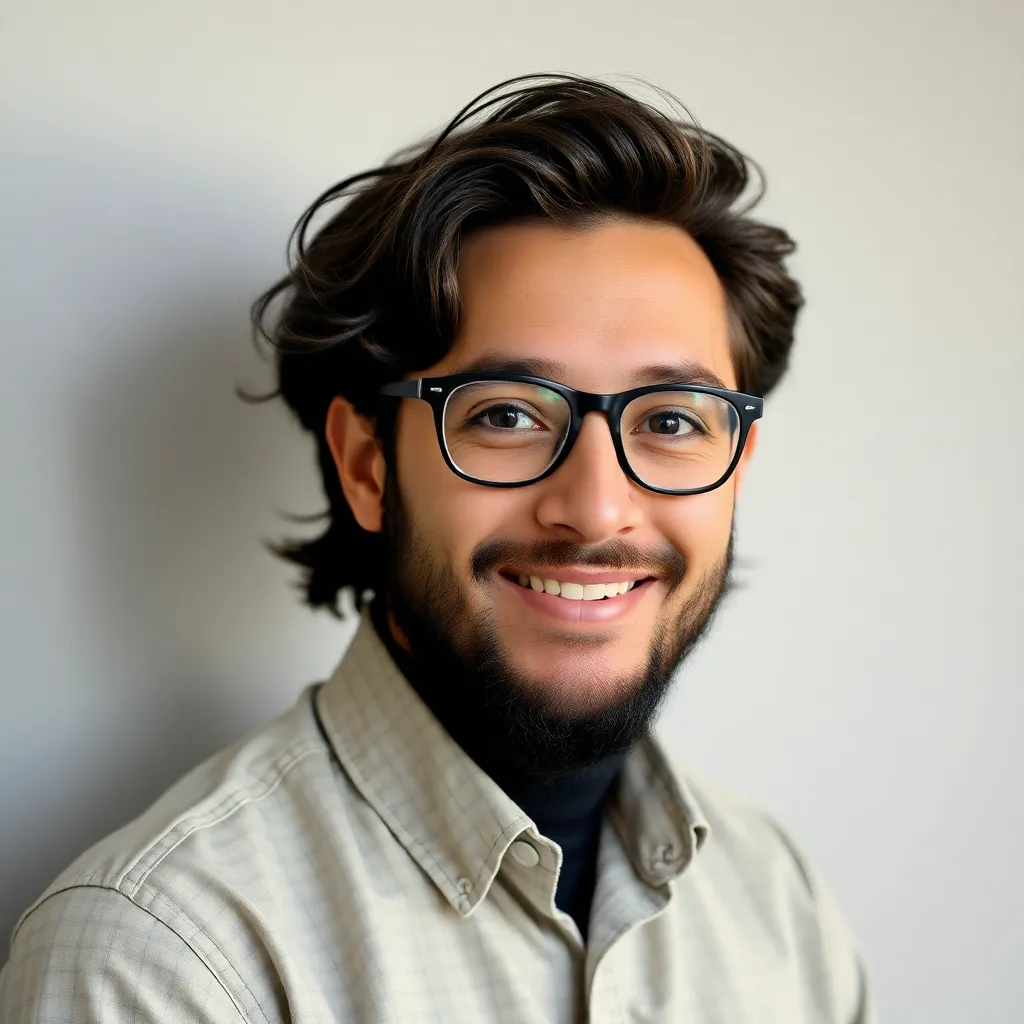
Holbox
Mar 13, 2025 · 6 min read

Table of Contents
- Pre-trained Multi Task Generative Ai Models Are Called:
- Table of Contents
- Pre-trained Multi-task Generative AI Models: A Deep Dive
- What are Pre-trained Multi-task Generative AI Models?
- Architectures and Training Methodologies
- Popular Applications
- Advantages and Limitations
- Future Trends and Developments
- Conclusion
- Latest Posts
- Related Post
Pre-trained Multi-task Generative AI Models: A Deep Dive
Pre-trained multi-task generative AI models represent a significant leap forward in artificial intelligence. These powerful models aren't just capable of performing a single task; they're trained on massive datasets to handle a multitude of generative tasks simultaneously, exhibiting impressive adaptability and efficiency. Understanding their capabilities and limitations is crucial for anyone looking to leverage the power of AI in various applications. This comprehensive guide will delve into the intricacies of these models, exploring their architecture, training methodologies, applications, and future prospects.
What are Pre-trained Multi-task Generative AI Models?
At their core, these models are sophisticated algorithms designed to generate new content. Unlike single-task models trained for specific purposes (like image classification or text translation), multi-task models are trained on a diverse range of tasks concurrently. This simultaneous training allows them to learn shared representations and features across different domains, leading to several key advantages:
- Improved Efficiency: Training a single model for multiple tasks is generally more efficient than training separate models for each task. This reduces computational costs and development time.
- Enhanced Generalization: Exposure to diverse tasks during training helps the model generalize better to unseen data and handle novel situations more effectively.
- Reduced Overfitting: Training on a large, varied dataset reduces the risk of overfitting, a phenomenon where a model performs exceptionally well on training data but poorly on new, unseen data.
- Transfer Learning Capabilities: The knowledge gained from one task can be transferred to another, improving performance on related tasks. This is a significant advantage in low-resource scenarios where data for a specific task might be limited.
These models typically leverage deep learning architectures, most commonly variations of transformers, convolutional neural networks (CNNs), or recurrent neural networks (RNNs). The specific architecture and training methods depend on the tasks the model is designed to handle.
Architectures and Training Methodologies
The architecture of a pre-trained multi-task generative AI model is often complex, reflecting the diverse tasks it's designed to perform. However, some common design principles include:
- Shared Embedding Layers: Many models use shared embedding layers to represent input data (text, images, audio) in a common latent space. This allows the model to learn relationships between different data types and tasks.
- Task-Specific Heads: Separate task-specific heads are often added on top of the shared layers. These heads are responsible for performing the specific task, such as generating text, creating images, or translating languages.
- Multi-Head Attention Mechanisms: Transformer architectures, particularly popular for natural language processing (NLP) tasks, rely heavily on multi-head attention mechanisms. These mechanisms allow the model to attend to different parts of the input data simultaneously, capturing complex relationships.
- Parameter Efficient Training: Techniques like Mixture of Experts (MoE) and dynamic routing are increasingly used to train large models efficiently, allowing for scalability and handling of diverse tasks without an explosion in the number of parameters.
The training process involves exposing the model to a massive dataset containing examples of various tasks. This dataset needs to be carefully curated to ensure sufficient representation of different task types and data modalities. The training process often involves:
- Unsupervised Pre-training: The model is initially pre-trained on a large, unlabeled dataset to learn general representations. This stage focuses on learning the underlying structure of the data without explicit task supervision.
- Supervised Fine-tuning: After pre-training, the model is fine-tuned on labeled datasets for specific tasks. This stage focuses on adapting the model's knowledge to the specific tasks it will perform.
- Multi-task Learning: The model is trained simultaneously on multiple tasks, allowing it to learn shared representations and features across different domains.
Popular Applications
The versatility of pre-trained multi-task generative AI models allows for their application across a wide range of domains, including:
- Natural Language Processing (NLP): Text generation, translation, summarization, question answering, sentiment analysis, and chatbot development are all areas where these models excel. They can generate human-quality text, translate languages fluently, and even engage in nuanced conversations.
- Computer Vision: Image generation, object detection, image captioning, and image segmentation are facilitated by these models. They can create realistic images, identify objects within images, and even generate descriptive captions.
- Audio Processing: Music generation, speech synthesis, and speech recognition are becoming increasingly sophisticated thanks to multi-task generative models. They can compose music in various styles, generate realistic speech, and accurately transcribe spoken words.
- Robotics: These models are playing a critical role in enhancing robot control, enabling robots to adapt to different environments and tasks more effectively.
- Drug Discovery: The ability to generate novel molecules and predict their properties is significantly improved through the application of these models in this field, accelerating the drug discovery process.
Advantages and Limitations
Advantages:
- Versatility: Handles multiple tasks with a single model.
- Efficiency: Reduces training time and computational costs.
- Generalization: Adapts better to new and unseen data.
- Transfer Learning: Knowledge gained in one task improves performance in related tasks.
Limitations:
- Data Dependency: Relies heavily on large, high-quality datasets for training.
- Computational Resources: Training and deploying these models requires significant computational power.
- Bias and Fairness: Models trained on biased data can perpetuate and amplify existing biases.
- Explainability: Understanding the decision-making process of these complex models can be challenging.
- Ethical Considerations: The potential misuse of these models for malicious purposes, such as generating deepfakes or spreading misinformation, raises serious ethical concerns.
Future Trends and Developments
The field of pre-trained multi-task generative AI models is constantly evolving. Some key future trends include:
- Increased Model Scalability: Larger and more complex models will continue to be developed, leading to even more impressive performance.
- Improved Efficiency: Research into more efficient training techniques will reduce the computational costs associated with these models.
- Enhanced Explainability: Methods for interpreting and understanding the internal workings of these models will be developed to enhance transparency and trust.
- Addressing Bias and Fairness: Techniques for mitigating bias and ensuring fairness in these models will be crucial for responsible AI development.
- Integration with Other AI Technologies: These models will be integrated with other AI technologies, such as reinforcement learning and knowledge graphs, to create even more powerful and versatile AI systems.
- Focus on specific domains: More specialized models will emerge, optimized for particular applications, such as medical image analysis or financial modeling.
Conclusion
Pre-trained multi-task generative AI models are transformative tools with the potential to revolutionize many aspects of our lives. Their ability to handle multiple tasks efficiently and adapt to new situations makes them incredibly versatile and powerful. However, it's crucial to address the limitations and ethical considerations associated with these models to ensure their responsible and beneficial development and deployment. As research progresses and new techniques emerge, we can expect these models to become even more sophisticated and impactful in the years to come. Understanding their capabilities and limitations is vital for anyone looking to leverage the potential of AI in their respective fields. The future of AI is intricately linked to the continued development and refinement of these remarkable models.
Latest Posts
Related Post
Thank you for visiting our website which covers about Pre-trained Multi Task Generative Ai Models Are Called: . We hope the information provided has been useful to you. Feel free to contact us if you have any questions or need further assistance. See you next time and don't miss to bookmark.