One Of Your Assignments At Work Is To Analyze
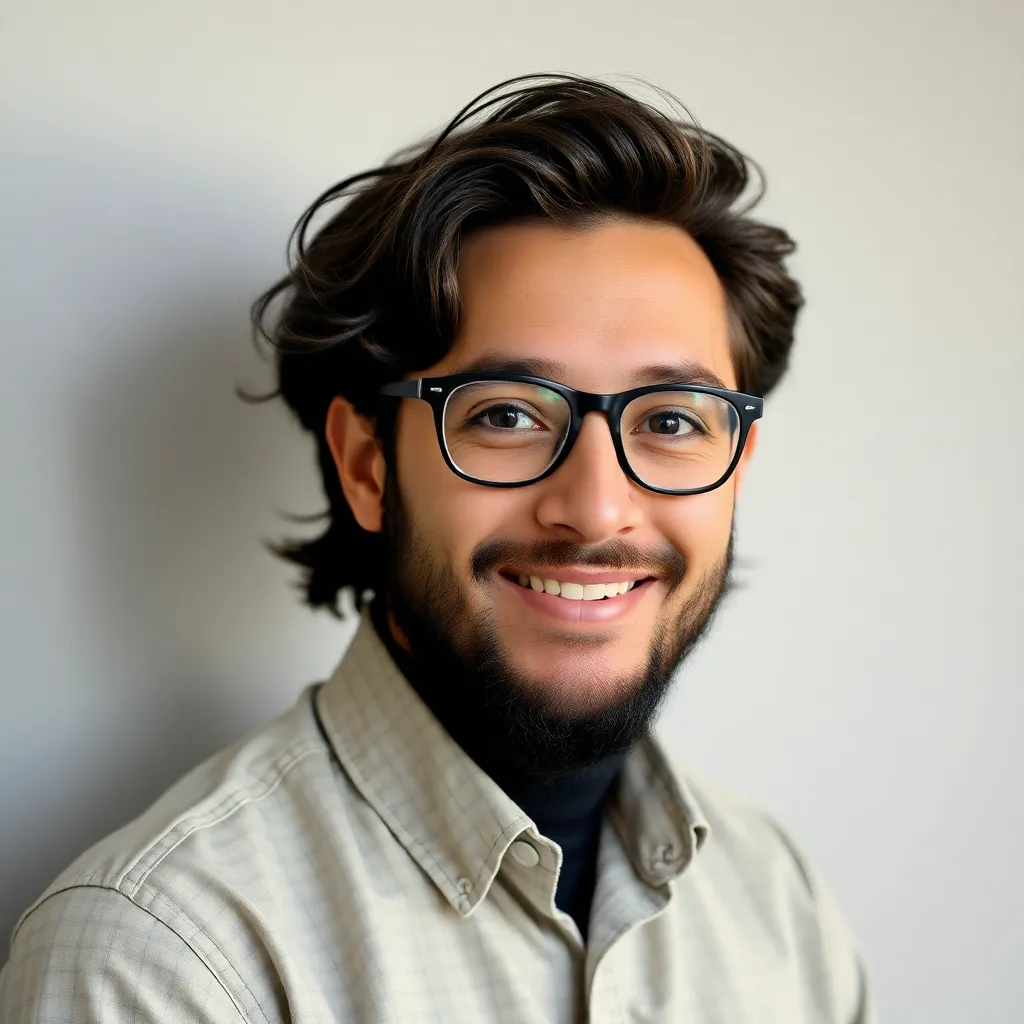
Holbox
Mar 28, 2025 · 7 min read
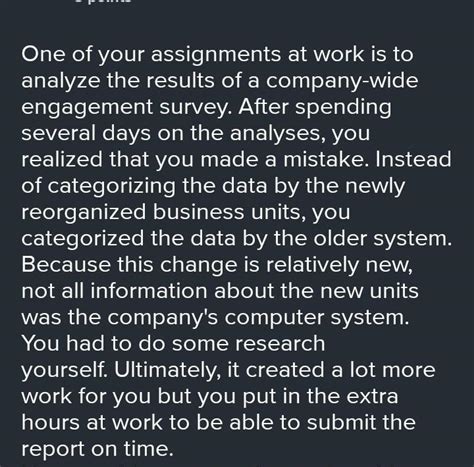
Table of Contents
- One Of Your Assignments At Work Is To Analyze
- Table of Contents
- Decoding the Data Deluge: A Deep Dive into Data Analysis Assignments
- Understanding the Assignment: The Foundation of Effective Analysis
- Data Acquisition and Preparation: The Cornerstone of Reliable Results
- Exploratory Data Analysis (EDA): Unveiling Hidden Patterns
- Advanced Analytical Techniques: Delving Deeper
- Communicating Results: The Final, Crucial Step
- Common Challenges and How to Overcome Them
- Strategies for Success
- Latest Posts
- Latest Posts
- Related Post
Decoding the Data Deluge: A Deep Dive into Data Analysis Assignments
Data analysis is no longer a niche skill; it's the lifeblood of modern businesses. As a data analyst, one of my core responsibilities involves tackling complex data analysis assignments. These aren't simple spreadsheet summaries; they're intricate puzzles requiring a blend of technical expertise, critical thinking, and creative problem-solving. This article will delve into the process of analyzing such assignments, highlighting the key stages involved, common challenges faced, and strategies for success.
Understanding the Assignment: The Foundation of Effective Analysis
Before even touching the data, the first and arguably most crucial step is thoroughly understanding the assignment's objectives. This involves more than simply reading the instructions; it requires a critical evaluation of the underlying business problem the analysis aims to solve. Key questions to ask include:
- What is the main question the analysis needs to answer? This should be clearly defined and concise. Ambiguous objectives will lead to ambiguous results.
- Who is the target audience for this analysis? The level of detail and the type of visualizations will vary depending on whether the audience is senior management, technical stakeholders, or clients.
- What are the key performance indicators (KPIs) that will be used to measure success? Identifying KPIs upfront helps focus the analysis and ensures the results are relevant and impactful.
- What are the data sources available, and are they reliable and comprehensive? Understanding data limitations is crucial to avoid drawing misleading conclusions. This often involves assessing data quality, identifying potential biases, and understanding any missing data.
- What is the timeline for completion? Effective time management is crucial, especially when dealing with multiple assignments.
Data Acquisition and Preparation: The Cornerstone of Reliable Results
Once the assignment's objectives are clear, the next step involves acquiring and preparing the data. This phase often consumes a significant portion of the overall analysis time and is crucial for the accuracy of the results. It includes several key sub-tasks:
-
Data Collection: This might involve accessing databases, extracting data from spreadsheets, scraping web data, or integrating data from various sources. The process requires careful attention to detail and adherence to data governance policies. Understanding the different data types (categorical, numerical, temporal) is also essential for appropriate analysis techniques.
-
Data Cleaning: Real-world data is rarely clean and tidy. It often contains missing values, inconsistencies, outliers, and errors. Data cleaning involves handling these issues, which might include:
- Handling missing values: This could involve imputation (filling in missing values using statistical methods), removing rows or columns with excessive missing data, or using specialized techniques depending on the context.
- Identifying and correcting inconsistencies: This might involve standardizing data formats, correcting spelling errors, or reconciling conflicting data entries.
- Outlier detection and treatment: Outliers can significantly skew results. Appropriate techniques, like box plots or z-score analysis, help identify and address them – whether by removing them, transforming them, or investigating the reasons for their occurrence.
-
Data Transformation: Raw data often needs transformation before analysis. This can involve:
- Feature scaling: Normalizing or standardizing data to ensure features with different scales don't disproportionately influence the analysis.
- Data aggregation: Summarizing data from multiple observations into meaningful aggregates (e.g., calculating averages, sums, or counts).
- Feature engineering: Creating new variables from existing ones to enhance the analysis (e.g., creating interaction terms or ratios).
-
Data Validation: After cleaning and transforming the data, it's crucial to validate its accuracy and consistency. This might involve comparing the processed data to the original source, performing cross-validation checks, or running consistency checks.
Exploratory Data Analysis (EDA): Unveiling Hidden Patterns
Once the data is prepared, exploratory data analysis (EDA) helps to understand its structure, identify patterns, and formulate hypotheses. EDA is an iterative process involving various techniques, including:
-
Descriptive Statistics: Calculating summary statistics like mean, median, standard deviation, percentiles, and frequencies to get a basic understanding of the data's distribution.
-
Data Visualization: Creating charts and graphs (histograms, scatter plots, box plots, etc.) to visually explore relationships between variables and identify potential trends or outliers. Choosing the right visualization is critical for effective communication.
-
Correlation Analysis: Investigating the relationships between different variables using correlation coefficients. This helps identify potential predictors or confounding factors.
-
Hypothesis Testing: Formulating hypotheses based on initial observations and testing them using appropriate statistical methods.
The goal of EDA is not to draw definitive conclusions but to generate insights and guide the subsequent in-depth analysis.
Advanced Analytical Techniques: Delving Deeper
Depending on the assignment's objectives, advanced analytical techniques might be necessary. These could include:
-
Regression Analysis: Predicting a continuous dependent variable based on one or more independent variables. Different types of regression (linear, logistic, polynomial) are used depending on the nature of the data and the research question.
-
Classification Analysis: Predicting a categorical dependent variable based on one or more independent variables. Techniques like decision trees, support vector machines, and neural networks are commonly used.
-
Clustering Analysis: Grouping similar data points together based on their characteristics. This is useful for identifying distinct customer segments or uncovering hidden patterns in the data.
-
Time Series Analysis: Analyzing data collected over time to identify trends, seasonality, and other patterns. Techniques like ARIMA and exponential smoothing are frequently employed.
Communicating Results: The Final, Crucial Step
Effective communication is essential for any data analysis assignment. The analysis results, no matter how insightful, are useless if they cannot be effectively communicated to the intended audience. This involves:
-
Clear and Concise Reporting: Presenting the findings in a clear, concise, and easy-to-understand manner. Avoiding technical jargon and using visuals effectively are crucial.
-
Visualizations: Using appropriate charts and graphs to communicate complex information effectively. Choosing the right visualization depends on the type of data and the message being conveyed.
-
Storytelling: Framing the results within a compelling narrative that highlights the key findings and their implications. This helps the audience connect with the analysis and understand its relevance.
-
Interactive Dashboards: For complex analyses, interactive dashboards can allow stakeholders to explore the data and drill down into specific details.
Common Challenges and How to Overcome Them
Data analysis assignments rarely go smoothly. Several common challenges include:
-
Data Quality Issues: Addressing missing data, inconsistencies, and outliers requires careful planning and execution. Using robust data cleaning and validation techniques is crucial.
-
Limited Data: Insufficient data can limit the insights that can be drawn. This might require additional data collection or the use of more sophisticated statistical techniques.
-
Time Constraints: Meeting deadlines while maintaining quality requires effective time management and prioritization.
-
Lack of Domain Expertise: Understanding the business context is critical for interpreting the results. Collaboration with domain experts is often necessary.
-
Technical Challenges: Using appropriate statistical software and techniques requires technical expertise. Continuous learning and upskilling are essential.
Strategies for Success
To consistently deliver high-quality data analysis, several strategies are crucial:
-
Structured Approach: Following a systematic process, from understanding the objectives to communicating the results, is essential for success.
-
Collaboration: Working effectively with stakeholders, subject matter experts, and other team members is critical.
-
Continuous Learning: The field of data analysis is constantly evolving. Staying up-to-date with the latest techniques and tools is crucial for professional growth.
-
Effective Communication: Clearly and concisely communicating findings is essential for impact.
-
Documentation: Maintaining thorough documentation of the entire analysis process, including data sources, methods used, and results obtained, is vital for reproducibility and auditability.
In conclusion, a data analysis assignment is more than just crunching numbers; it’s about uncovering hidden truths within the data and communicating those truths effectively. By following a structured approach, mastering various analytical techniques, and consistently prioritizing clear communication, you can not only successfully complete your assignments but also contribute significantly to informed decision-making within your organization. The ability to transform raw data into actionable insights is a valuable skill in today's data-driven world. This systematic and detailed approach ensures accuracy, efficiency, and meaningful contributions to the business challenges at hand.
Latest Posts
Latest Posts
-
A Patient Who Presents With A Headache Fever Confusion
Mar 31, 2025
-
Which Of The Following Best Describes A Misrepresentation
Mar 31, 2025
-
Another Common Term For Stockholders Equity Is
Mar 31, 2025
-
In Equity Theory Employees Are Motivated To
Mar 31, 2025
-
A Gallup Poll Conducted Telephone Interviews
Mar 31, 2025
Related Post
Thank you for visiting our website which covers about One Of Your Assignments At Work Is To Analyze . We hope the information provided has been useful to you. Feel free to contact us if you have any questions or need further assistance. See you next time and don't miss to bookmark.