Monitoring Progress And Modeling With Mathematics
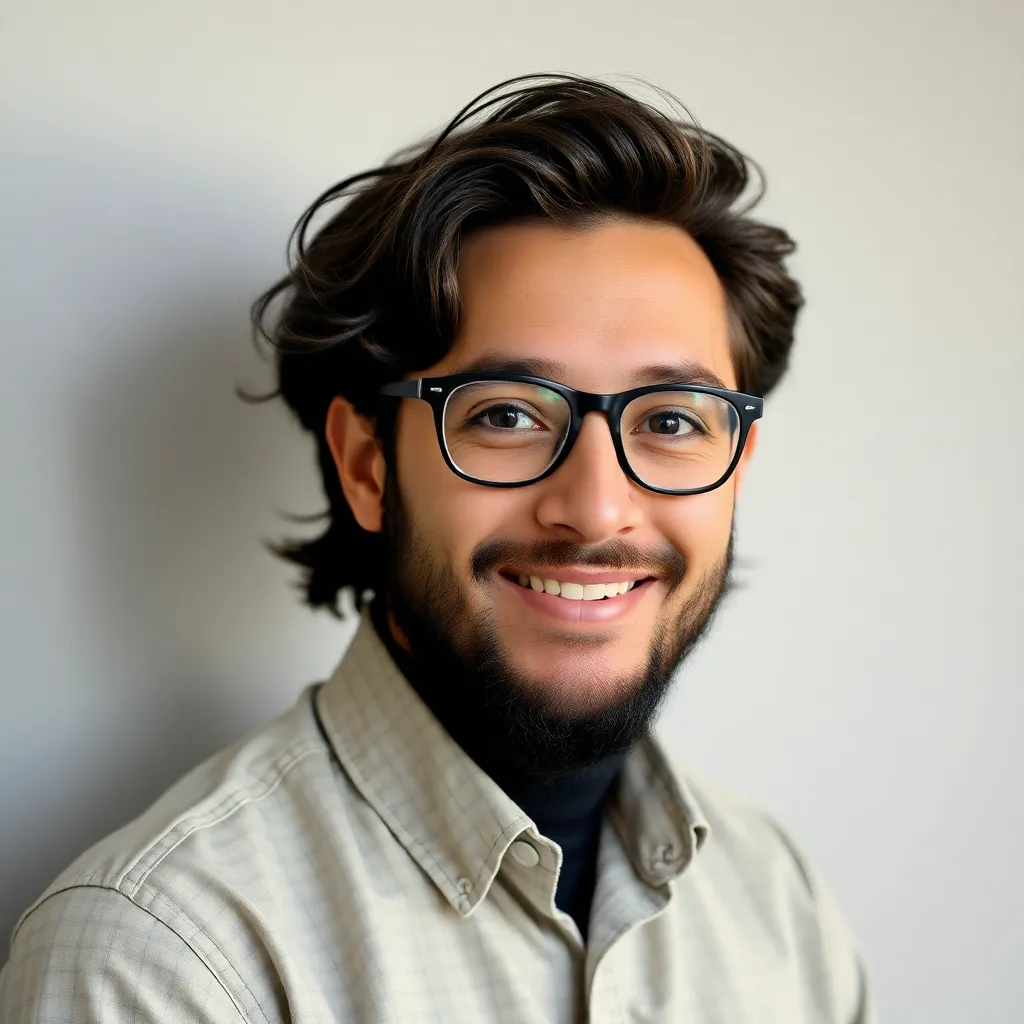
Holbox
Apr 03, 2025 · 6 min read

Table of Contents
- Monitoring Progress And Modeling With Mathematics
- Table of Contents
- Monitoring Progress and Modeling with Mathematics: A Powerful Duo
- The Importance of Monitoring Progress
- 1. Goal Clarity and Motivation: Tracking progress reinforces our commitment to our goals. Seeing tangible evidence of advancement, no matter how small, boosts motivation and helps us stay on track, especially during challenging periods.
- 2. Early Problem Detection: Consistent monitoring allows us to identify potential issues early on. This proactive approach minimizes the impact of setbacks and allows for timely interventions, preventing minor problems from escalating into major obstacles.
- 3. Improved Decision-Making: Data gathered through progress monitoring provides a concrete basis for informed decision-making. Instead of relying on intuition or guesswork, we can make adjustments based on objective evidence, leading to more effective strategies and better outcomes.
- 4. Accountability and Transparency: Formalized progress monitoring fosters accountability, both for individuals and teams. It promotes transparency, allowing stakeholders to understand the current state of affairs and adjust expectations accordingly.
- 5. Enhanced Learning and Adaptation: By analyzing the data collected through monitoring, we can gain valuable insights into what works and what doesn't. This feedback loop facilitates continuous learning and adaptation, leading to improvements in processes and methodologies.
- Mathematical Modeling: The Engine of Prediction
- 1. Linear Models: These models assume a linear relationship between variables. They are relatively simple to understand and implement, making them suitable for situations where the relationship is approximately linear. Simple examples include calculating the distance traveled based on speed and time, or projecting revenue based on sales per unit and the number of units sold.
- 2. Exponential Models: Used when growth or decay is proportional to the current value. Common examples include population growth, radioactive decay, and compound interest. These models often involve exponential functions, such as e<sup>kt</sup>, where 'k' represents the growth or decay rate and 't' represents time.
- 3. Logistic Models: Describe situations where growth is initially rapid but eventually levels off due to limiting factors. These models are frequently used in population ecology, epidemiology, and the spread of information or technology. The logistic function, often represented as S-shaped curve, captures this behavior effectively.
- 4. Differential Equations: These equations describe the rate of change of one or more variables with respect to others. They are powerful tools for modeling dynamic systems, such as fluid flow, chemical reactions, and the spread of diseases. Solving these equations often involves advanced mathematical techniques, but numerical methods can provide approximate solutions.
- 5. Statistical Models: Used to analyze data sets and make inferences about underlying relationships between variables. Regression analysis, for instance, can be used to model the relationship between a dependent variable and one or more independent variables. Statistical models are crucial for understanding variability and uncertainty in predictions.
- Integrating Monitoring and Modeling: Case Studies
- 1. Personal Fitness Goals: Monitoring your weight, exercise duration, and calorie intake provides valuable data. This data can be used to create a simple linear model to project your weight loss over time, assuming a consistent pattern. However, incorporating factors like muscle gain (which can increase weight despite fat loss) requires a more sophisticated model.
- 2. Project Management: Monitoring task completion, resource allocation, and budget expenditure allows for the creation of a Gantt chart, a visual representation of project timeline. Mathematical models can help predict potential delays based on current progress and forecast resource needs based on project complexity. The Critical Path Method (CPM) uses network diagrams and critical path analysis to optimize project scheduling.
- 3. Business Forecasting: Monitoring sales figures, marketing campaign performance, and economic indicators provides data for predicting future revenue. Time series analysis, exponential smoothing, or ARIMA models can be employed to forecast future sales based on past trends and seasonality.
- 4. Environmental Monitoring: Monitoring pollution levels, deforestation rates, and climate change indicators generates crucial data. Mathematical models are vital for simulating environmental processes, predicting the impact of human activities, and informing policy decisions. These models often involve complex differential equations and simulations.
- 5. Epidemiological Modeling: Monitoring the spread of infectious diseases is critical for public health management. Compartmental models (SIR, SEIR) use differential equations to simulate the dynamics of infection, transmission, and recovery, enabling the prediction of outbreak progression and the evaluation of intervention strategies.
- Challenges and Limitations
- 1. Data Quality: The accuracy of predictions heavily relies on the quality of the monitored data. Inaccurate, incomplete, or biased data will lead to unreliable models and erroneous conclusions.
- 2. Model Assumptions: Mathematical models are based on simplifying assumptions. The validity of the model depends on how well these assumptions reflect the reality of the situation. Overly simplistic models may fail to capture crucial aspects of the system being studied.
- 3. Unpredictable Events: Models typically struggle to incorporate unpredictable events, such as natural disasters or sudden economic shifts. These events can significantly disrupt the predicted trajectory.
- 4. Complexity: Many real-world systems are highly complex, involving numerous interacting variables. Building accurate models for such systems can be extremely challenging, requiring advanced mathematical techniques and substantial computational resources.
- 5. Interpretation and Communication: The results of mathematical models must be interpreted carefully and communicated effectively to the relevant stakeholders. Misinterpretation or oversimplification of the results can lead to poor decision-making.
- Conclusion
- Latest Posts
- Latest Posts
- Related Post
Monitoring Progress and Modeling with Mathematics: A Powerful Duo
Mathematics, often perceived as a dry and abstract subject, is in reality a powerful tool for understanding and shaping the world around us. One particularly impactful application lies in its ability to monitor progress and build predictive models. This combination allows us to not only track our achievements but also anticipate future outcomes, optimize strategies, and make informed decisions across a vast range of fields. From personal fitness goals to large-scale engineering projects, the synergy between progress monitoring and mathematical modeling is undeniable.
The Importance of Monitoring Progress
Before delving into the mathematical aspects, let's establish the fundamental importance of monitoring progress. Without a clear understanding of where we stand, it's impossible to gauge effectiveness, identify weaknesses, or make necessary adjustments. Regular monitoring provides several critical benefits:
1. Goal Clarity and Motivation: Tracking progress reinforces our commitment to our goals. Seeing tangible evidence of advancement, no matter how small, boosts motivation and helps us stay on track, especially during challenging periods.
2. Early Problem Detection: Consistent monitoring allows us to identify potential issues early on. This proactive approach minimizes the impact of setbacks and allows for timely interventions, preventing minor problems from escalating into major obstacles.
3. Improved Decision-Making: Data gathered through progress monitoring provides a concrete basis for informed decision-making. Instead of relying on intuition or guesswork, we can make adjustments based on objective evidence, leading to more effective strategies and better outcomes.
4. Accountability and Transparency: Formalized progress monitoring fosters accountability, both for individuals and teams. It promotes transparency, allowing stakeholders to understand the current state of affairs and adjust expectations accordingly.
5. Enhanced Learning and Adaptation: By analyzing the data collected through monitoring, we can gain valuable insights into what works and what doesn't. This feedback loop facilitates continuous learning and adaptation, leading to improvements in processes and methodologies.
Mathematical Modeling: The Engine of Prediction
Mathematical modeling is the process of using mathematical concepts and language to represent and analyze real-world phenomena. It allows us to translate complex situations into manageable equations, providing a framework for prediction and optimization. Various mathematical techniques can be employed, depending on the nature of the problem:
1. Linear Models: These models assume a linear relationship between variables. They are relatively simple to understand and implement, making them suitable for situations where the relationship is approximately linear. Simple examples include calculating the distance traveled based on speed and time, or projecting revenue based on sales per unit and the number of units sold.
2. Exponential Models: Used when growth or decay is proportional to the current value. Common examples include population growth, radioactive decay, and compound interest. These models often involve exponential functions, such as e<sup>kt</sup>, where 'k' represents the growth or decay rate and 't' represents time.
3. Logistic Models: Describe situations where growth is initially rapid but eventually levels off due to limiting factors. These models are frequently used in population ecology, epidemiology, and the spread of information or technology. The logistic function, often represented as S-shaped curve, captures this behavior effectively.
4. Differential Equations: These equations describe the rate of change of one or more variables with respect to others. They are powerful tools for modeling dynamic systems, such as fluid flow, chemical reactions, and the spread of diseases. Solving these equations often involves advanced mathematical techniques, but numerical methods can provide approximate solutions.
5. Statistical Models: Used to analyze data sets and make inferences about underlying relationships between variables. Regression analysis, for instance, can be used to model the relationship between a dependent variable and one or more independent variables. Statistical models are crucial for understanding variability and uncertainty in predictions.
Integrating Monitoring and Modeling: Case Studies
The true power of this combination emerges when we integrate progress monitoring with mathematical modeling. This allows for predictive capabilities and informed decision-making. Let's consider some illustrative examples:
1. Personal Fitness Goals: Monitoring your weight, exercise duration, and calorie intake provides valuable data. This data can be used to create a simple linear model to project your weight loss over time, assuming a consistent pattern. However, incorporating factors like muscle gain (which can increase weight despite fat loss) requires a more sophisticated model.
2. Project Management: Monitoring task completion, resource allocation, and budget expenditure allows for the creation of a Gantt chart, a visual representation of project timeline. Mathematical models can help predict potential delays based on current progress and forecast resource needs based on project complexity. The Critical Path Method (CPM) uses network diagrams and critical path analysis to optimize project scheduling.
3. Business Forecasting: Monitoring sales figures, marketing campaign performance, and economic indicators provides data for predicting future revenue. Time series analysis, exponential smoothing, or ARIMA models can be employed to forecast future sales based on past trends and seasonality.
4. Environmental Monitoring: Monitoring pollution levels, deforestation rates, and climate change indicators generates crucial data. Mathematical models are vital for simulating environmental processes, predicting the impact of human activities, and informing policy decisions. These models often involve complex differential equations and simulations.
5. Epidemiological Modeling: Monitoring the spread of infectious diseases is critical for public health management. Compartmental models (SIR, SEIR) use differential equations to simulate the dynamics of infection, transmission, and recovery, enabling the prediction of outbreak progression and the evaluation of intervention strategies.
Challenges and Limitations
While the combined power of monitoring and modeling is significant, it's crucial to acknowledge inherent challenges and limitations:
1. Data Quality: The accuracy of predictions heavily relies on the quality of the monitored data. Inaccurate, incomplete, or biased data will lead to unreliable models and erroneous conclusions.
2. Model Assumptions: Mathematical models are based on simplifying assumptions. The validity of the model depends on how well these assumptions reflect the reality of the situation. Overly simplistic models may fail to capture crucial aspects of the system being studied.
3. Unpredictable Events: Models typically struggle to incorporate unpredictable events, such as natural disasters or sudden economic shifts. These events can significantly disrupt the predicted trajectory.
4. Complexity: Many real-world systems are highly complex, involving numerous interacting variables. Building accurate models for such systems can be extremely challenging, requiring advanced mathematical techniques and substantial computational resources.
5. Interpretation and Communication: The results of mathematical models must be interpreted carefully and communicated effectively to the relevant stakeholders. Misinterpretation or oversimplification of the results can lead to poor decision-making.
Conclusion
Monitoring progress and modeling with mathematics provide a powerful framework for understanding, predicting, and optimizing various aspects of our lives and work. By combining the benefits of data-driven insights with the predictive power of mathematical models, we can make more informed decisions, improve our efficiency, and achieve better outcomes across diverse fields. However, it is crucial to be aware of the limitations of this approach and to employ critical thinking in interpreting the results. The continuous refinement of both data collection methods and mathematical techniques will further enhance the power and applicability of this dynamic duo. The future of effective decision-making lies in the intelligent integration of these two powerful tools.
Latest Posts
Latest Posts
-
Car And Truck Drivers Tend To Drive As They Live
Apr 08, 2025
-
Draw All Resonance Structures For The Carbonate Ion Co32
Apr 08, 2025
-
When Comparing Free Weights And Weight Machines Free Weights
Apr 08, 2025
-
Choose The Best Lewis Structure For Bf3
Apr 08, 2025
-
The Smaller The Group The More
Apr 08, 2025
Related Post
Thank you for visiting our website which covers about Monitoring Progress And Modeling With Mathematics . We hope the information provided has been useful to you. Feel free to contact us if you have any questions or need further assistance. See you next time and don't miss to bookmark.