If The Coefficient Of Determination Is Close To 1 Then
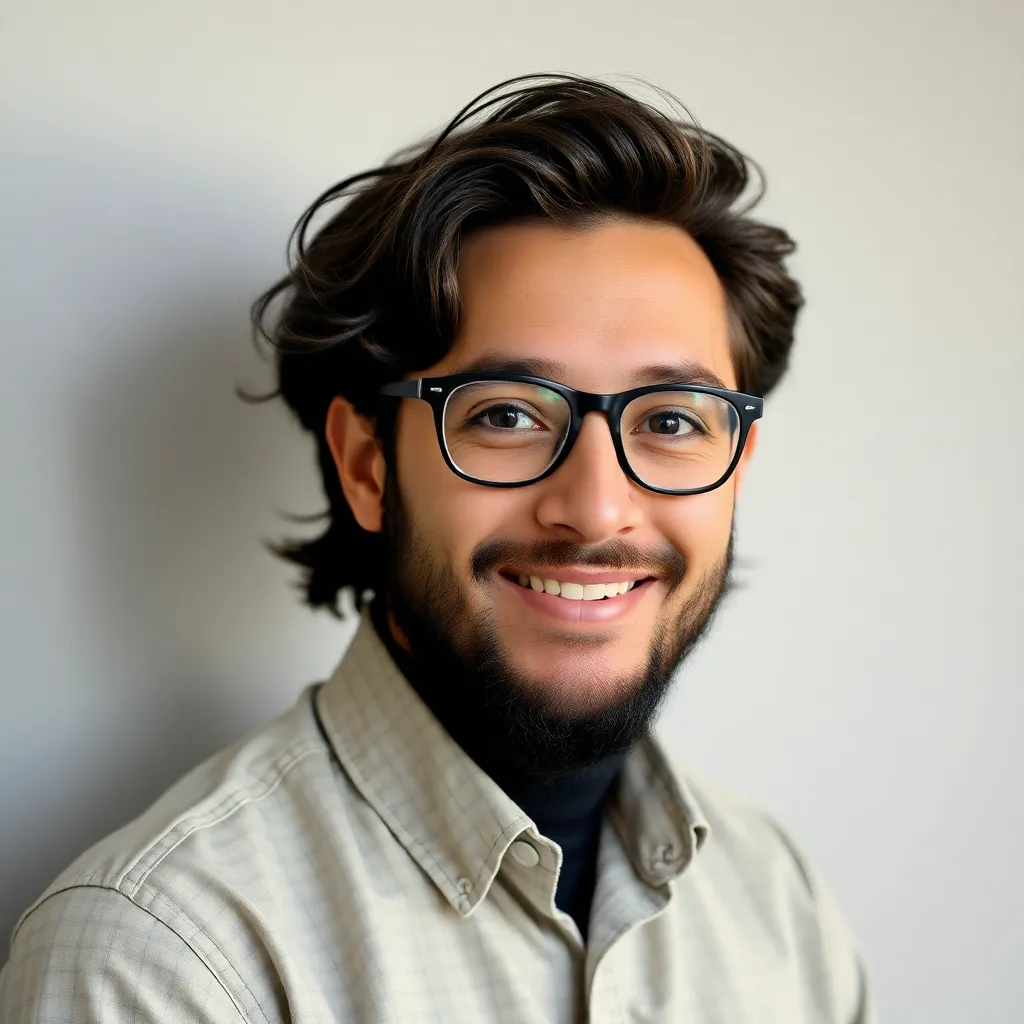
Holbox
Apr 04, 2025 · 6 min read

Table of Contents
- If The Coefficient Of Determination Is Close To 1 Then
- Table of Contents
- If the Coefficient of Determination is Close to 1, Then… A Deep Dive into R-squared
- Understanding R-squared: A Quick Recap
- When R-squared is Close to 1: What Does It Imply?
- Examples of Scenarios with High R-squared:
- Caveats and Limitations: When a High R-squared Isn't Enough
- 1. Overfitting: The Enemy of Generalization
- 2. Causation vs. Correlation: A Critical Distinction
- 3. Data Quality and Outliers: The Foundation of Reliability
- 4. Ignoring Model Assumptions: The Importance of Diagnostics
- 5. Context Matters: R-squared in Different Fields
- Adjusted R-squared: A More Robust Measure
- Improving R-squared: Strategies and Techniques
- Conclusion: R-squared – A Valuable Tool, Not a Sole Determinant
- Latest Posts
- Latest Posts
- Related Post
If the Coefficient of Determination is Close to 1, Then… A Deep Dive into R-squared
The coefficient of determination, more commonly known as R-squared (R²), is a crucial statistical measure used in regression analysis. It quantifies the proportion of variance in the dependent variable that is predictable from the independent variable(s). A value close to 1 signifies a strong relationship, but understanding what this means in practical terms requires a deeper dive. This article explores the implications of an R-squared value approaching 1, discussing its strengths, limitations, and proper interpretation within the context of different scenarios.
Understanding R-squared: A Quick Recap
Before delving into the implications of an R-squared value close to 1, let's briefly review its fundamental meaning. R-squared represents the percentage of the total variation in the dependent variable that is explained by the independent variables in the model. Mathematically, it's the ratio of the explained variance to the total variance:
R² = Explained Variance / Total Variance
A higher R-squared indicates a better fit of the model to the data, suggesting that the independent variables are good predictors of the dependent variable. Conversely, a lower R-squared implies a weaker relationship, with less of the dependent variable's variation being explained by the model. The R-squared value always falls between 0 and 1 (or 0% and 100%).
When R-squared is Close to 1: What Does It Imply?
When the coefficient of determination approaches 1 (e.g., 0.95, 0.98, or even higher), it strongly suggests a significant relationship between the dependent and independent variables. This indicates that:
-
High Predictive Power: The model has a high ability to predict the value of the dependent variable based on the values of the independent variables. This is invaluable for forecasting and making informed decisions.
-
Strong Correlation: A high R-squared reflects a strong correlation between the variables included in the model. This doesn't necessarily imply causation, but it does highlight a strong association.
-
Good Model Fit: The model accurately captures the underlying relationship between the variables. The observed data points lie closely around the regression line, minimizing the residuals (the differences between the predicted and actual values).
-
Reduced Uncertainty: The high explanatory power reduces uncertainty in predictions. The model provides more precise estimations of the dependent variable, making it more reliable for decision-making.
Examples of Scenarios with High R-squared:
-
Predicting Housing Prices: A model predicting house prices based on factors like location, size, and amenities might have a high R-squared if these factors are strong determinants of price.
-
Analyzing Sales Revenue: A model predicting sales revenue based on marketing spend and advertising campaigns could yield a high R-squared if these variables are major drivers of sales.
-
Modeling Stock Prices: While challenging, a model predicting stock prices based on various economic indicators might achieve a relatively high R-squared, although it's important to acknowledge the inherent volatility and randomness in stock markets.
Caveats and Limitations: When a High R-squared Isn't Enough
While a high R-squared is generally desirable, it's crucial to understand its limitations:
1. Overfitting: The Enemy of Generalization
A high R-squared doesn't automatically guarantee a good model. A model can achieve a high R-squared by simply including many independent variables, even if some are irrelevant. This leads to overfitting, where the model performs exceptionally well on the training data but poorly on new, unseen data. Overfitting essentially means the model has memorized the training data rather than learning the underlying patterns. Techniques like cross-validation and regularization help mitigate overfitting.
2. Causation vs. Correlation: A Critical Distinction
A high R-squared indicates a strong correlation, but it does not prove causation. Two variables might have a strong correlation, but one might not necessarily cause the other. There could be other confounding factors influencing both variables, or the relationship might be spurious (random). Careful analysis and consideration of potential confounders are vital.
3. Data Quality and Outliers: The Foundation of Reliability
The quality of the data significantly impacts the R-squared value. Outliers (extreme data points) can disproportionately influence the regression line and inflate the R-squared, leading to a misleadingly high value. Careful data cleaning and outlier detection are essential for accurate results. Robust regression techniques can help minimize the influence of outliers.
4. Ignoring Model Assumptions: The Importance of Diagnostics
Regression models rely on certain assumptions (e.g., linearity, independence of errors, constant variance). Violating these assumptions can lead to biased and unreliable estimates, even if the R-squared is high. Diagnostic checks, such as residual plots and tests for heteroscedasticity and autocorrelation, are critical for assessing model validity.
5. Context Matters: R-squared in Different Fields
The interpretation of R-squared also depends on the context of the analysis. In some fields, a relatively lower R-squared might be acceptable, while in others, a very high R-squared might be expected. For example, in social sciences, R-squared values often fall in the moderate range, while in physics or engineering, much higher values might be common.
Adjusted R-squared: A More Robust Measure
To address the issue of overfitting, the adjusted R-squared is often preferred over the standard R-squared. The adjusted R-squared penalizes the inclusion of irrelevant variables, providing a more accurate reflection of the model's predictive power. It's particularly useful when comparing models with different numbers of independent variables. A higher adjusted R-squared indicates a better model, even if it has fewer variables.
Improving R-squared: Strategies and Techniques
While a high R-squared is a goal, achieving it ethically and meaningfully requires careful attention to several factors:
-
Feature Engineering: Carefully selecting and transforming independent variables can significantly improve the model's explanatory power. This includes creating new variables, transforming existing ones (e.g., logarithmic transformations), and using interaction terms.
-
Model Selection: Choosing the right regression model (e.g., linear, polynomial, logistic) is crucial for accurately capturing the relationship between variables. Different models are better suited for different data patterns.
-
Regularization Techniques: Techniques like Ridge Regression and Lasso Regression help prevent overfitting by adding penalties to the model's complexity. These methods shrink the coefficients of less important variables, improving the model's generalization ability.
-
Dealing with Outliers and Missing Data: Addressing outliers and missing data is crucial for ensuring data quality and model reliability. Methods include outlier detection techniques, imputation (filling in missing values), and robust regression methods.
Conclusion: R-squared – A Valuable Tool, Not a Sole Determinant
An R-squared value close to 1 is generally desirable, suggesting a strong relationship between variables and high predictive power. However, it's vital to interpret this value cautiously, considering the limitations of R-squared and employing additional diagnostic checks. Overfitting, correlation versus causation, data quality issues, and model assumptions all need careful consideration. Using adjusted R-squared, employing appropriate model selection techniques, and focusing on data quality are essential for building robust and reliable models. Ultimately, a high R-squared is a valuable indicator, but it should not be the sole determinant of model quality. A comprehensive approach, incorporating various statistical techniques and a deep understanding of the data and the research question, is crucial for drawing meaningful conclusions.
Latest Posts
Latest Posts
-
The Patient Is Awake And Alert The States Quizlet
Apr 08, 2025
-
A Companys Required Rate Of Return Is Called The
Apr 08, 2025
-
With The Community Interested In Eating Healthy
Apr 08, 2025
-
To Form An Ion A Sodium Atom
Apr 08, 2025
-
Which Of The Following Is A Presentation Method Of Training
Apr 08, 2025
Related Post
Thank you for visiting our website which covers about If The Coefficient Of Determination Is Close To 1 Then . We hope the information provided has been useful to you. Feel free to contact us if you have any questions or need further assistance. See you next time and don't miss to bookmark.