Identify The Steps In Ibm's Ladder Approach To Ai
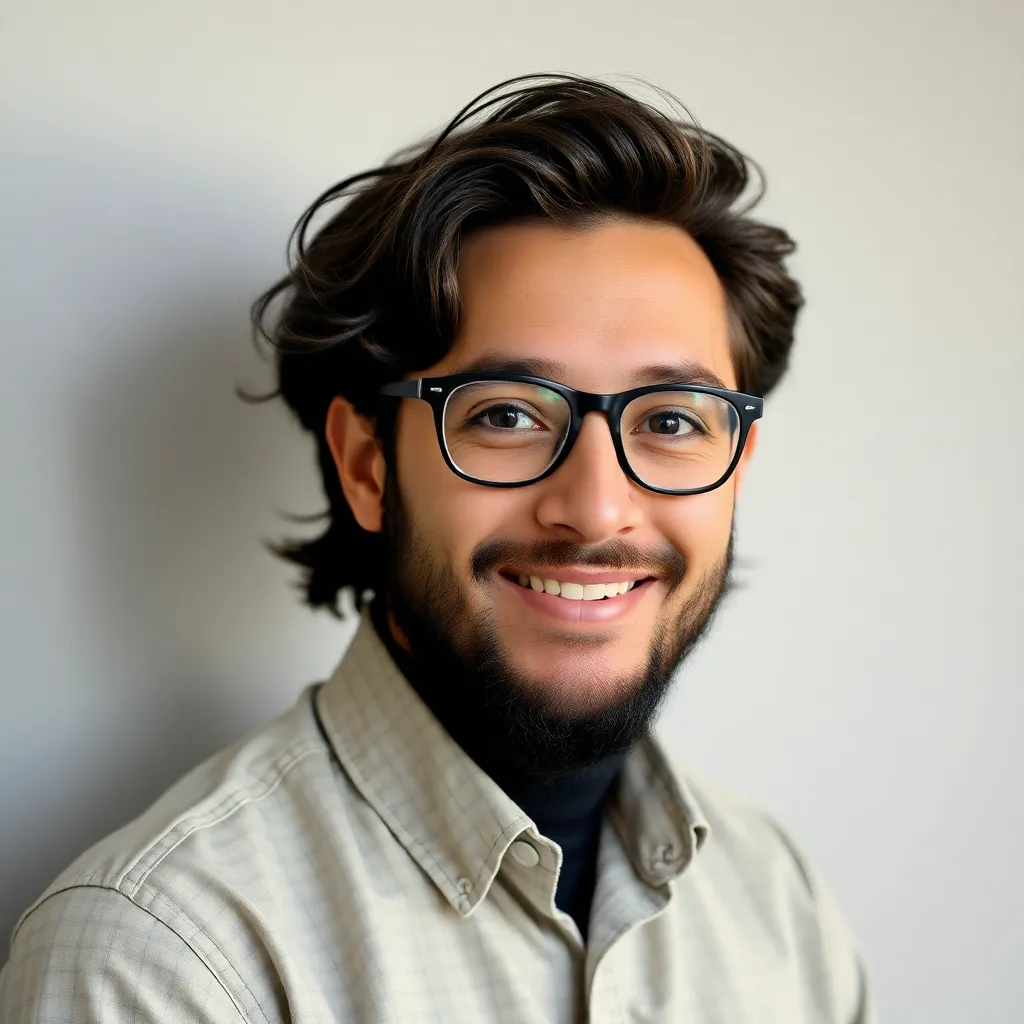
Holbox
May 07, 2025 · 7 min read
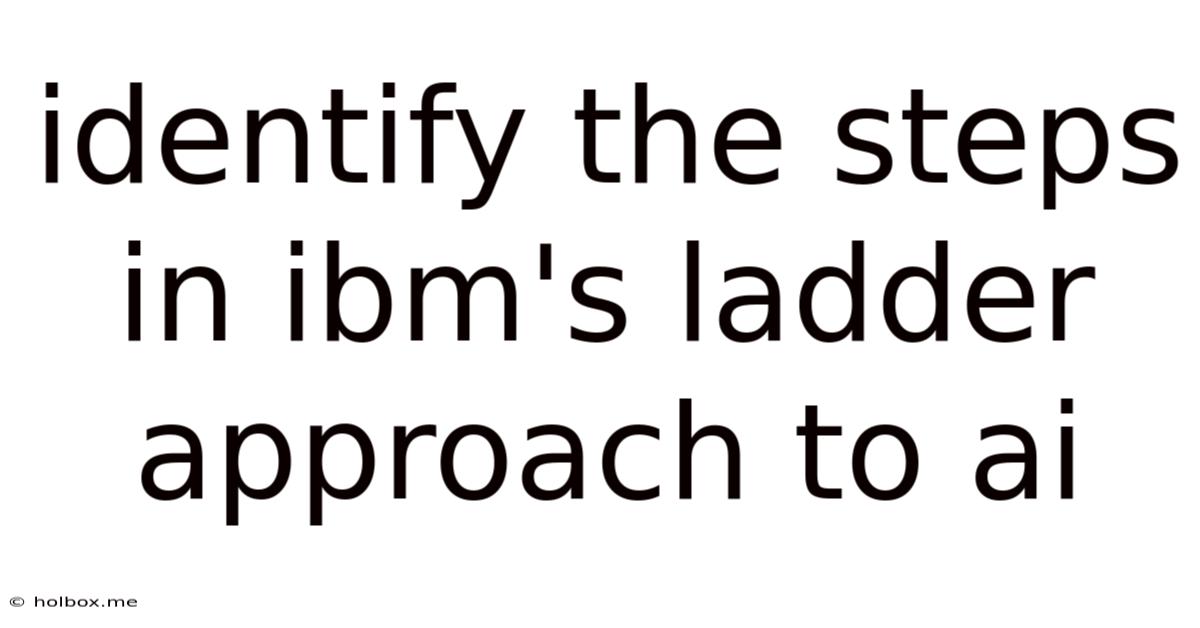
Table of Contents
- Identify The Steps In Ibm's Ladder Approach To Ai
- Table of Contents
- IBM's Ladder Approach to AI: A Step-by-Step Guide to AI Adoption
- Understanding IBM's AI Ladder: A Foundation for Strategic AI Adoption
- The Rungs of IBM's AI Ladder: A Detailed Exploration
- 1. Data & AI Foundations: Building the Base
- 2. Descriptive Analytics: Understanding the Past
- 3. Predictive Analytics: Forecasting the Future
- 4. Prescriptive Analytics: Optimizing Decisions
- 5. Cognitive and Autonomous Systems: Automating Processes
- Navigating the Ladder: Considerations for Successful AI Implementation
- Conclusion: Embracing the Power of IBM's AI Ladder
- Latest Posts
- Related Post
IBM's Ladder Approach to AI: A Step-by-Step Guide to AI Adoption
Artificial intelligence (AI) is rapidly transforming industries, offering unprecedented opportunities for businesses to optimize processes, enhance decision-making, and drive innovation. However, implementing AI effectively can be a complex undertaking. IBM's Ladder approach provides a structured and pragmatic framework for organizations to navigate this complexity, facilitating a gradual and sustainable AI adoption journey. This approach, rather than a sudden leap into complex AI solutions, emphasizes a phased implementation, starting with simpler techniques and progressively scaling up to more advanced capabilities.
This comprehensive guide will delve into the individual steps of IBM's AI Ladder, examining each level in detail and providing practical examples to illustrate their application.
Understanding IBM's AI Ladder: A Foundation for Strategic AI Adoption
IBM's AI Ladder isn't just a technological roadmap; it's a strategic framework for organizational transformation. It acknowledges that AI adoption is not a one-size-fits-all solution and that different organizations possess varying levels of AI maturity. The ladder structure facilitates a gradual progression, allowing organizations to build a strong foundation before tackling more advanced AI capabilities. This minimizes risk, maximizes return on investment (ROI), and fosters a culture of data-driven decision-making.
The Rungs of IBM's AI Ladder: A Detailed Exploration
The IBM AI Ladder is typically depicted as a five-runged structure, each rung representing a distinct level of AI sophistication and capability:
1. Data & AI Foundations: Building the Base
This foundational level focuses on establishing the essential prerequisites for successful AI implementation. It's about creating a robust and reliable infrastructure capable of supporting AI initiatives. Key aspects of this stage include:
-
Data Management: This involves organizing, cleaning, and preparing data for analysis. This includes addressing data quality issues, handling missing values, and ensuring data consistency across different sources. Effective data governance is crucial here, establishing clear policies and procedures for data access, usage, and security. This foundational step is often the most time-consuming but undeniably the most crucial. Poor data quality will invariably lead to poor AI results.
-
Cloud Adoption: Leveraging cloud platforms provides scalable and cost-effective computing resources necessary for processing large datasets. Cloud services offer a range of AI-specific tools and services, accelerating development and deployment. The choice of cloud provider should be aligned with the organization’s specific needs and existing infrastructure.
-
AI Skills Development: Building internal expertise is vital. This involves training existing employees in data science, machine learning, and AI principles, or recruiting professionals with relevant skills. Investing in upskilling and reskilling initiatives is critical for long-term success.
-
Infrastructure Modernization: This includes upgrading existing IT infrastructure to handle the demands of AI workloads. This might involve deploying new hardware, software, and network capabilities. Robust security measures are essential to protect sensitive data.
Example: A retail company at this stage might focus on consolidating its disparate customer data sources into a central data warehouse, implementing cloud-based data storage, and training its data analysts on basic data manipulation techniques.
2. Descriptive Analytics: Understanding the Past
Once a solid foundation is established, the journey moves towards descriptive analytics. This level focuses on understanding past events and identifying patterns within existing data. Techniques employed here include:
-
Data Visualization: Creating dashboards and reports to visualize key performance indicators (KPIs) and gain insights into historical data. This allows for a clear understanding of past trends and patterns.
-
Data Mining: Discovering previously unknown patterns and relationships within datasets using statistical techniques. This helps in identifying correlations and anomalies.
-
Reporting and Business Intelligence: Generating reports and summaries that provide actionable insights into business performance. This allows for informed decision-making based on historical data.
Example: The same retail company could use descriptive analytics to analyze past sales data to identify peak selling seasons, best-selling products, and customer demographics with the highest purchasing power. This information can then be used to inform future marketing strategies and inventory management.
3. Predictive Analytics: Forecasting the Future
This level builds upon descriptive analytics by employing advanced techniques to predict future outcomes. Predictive analytics allows organizations to proactively address potential issues and capitalize on emerging opportunities. Key techniques include:
-
Machine Learning (ML) Models: Building predictive models using algorithms that learn from historical data to predict future events. This can include regression models, classification models, and time series forecasting.
-
Forecasting: Predicting future trends and values based on historical patterns and external factors. This is valuable for planning and resource allocation.
-
Risk Management: Identifying and assessing potential risks based on predictive models. This allows for proactive mitigation strategies.
Example: The retail company could use predictive analytics to forecast future demand for specific products, optimize inventory levels, and personalize marketing campaigns based on predicted customer behavior. They might also predict potential customer churn and implement retention strategies.
4. Prescriptive Analytics: Optimizing Decisions
Prescriptive analytics goes beyond prediction; it focuses on recommending actions to optimize outcomes. This level involves leveraging AI to guide decision-making and improve business processes. Techniques used include:
-
Optimization Algorithms: Employing algorithms to find the best possible solution among multiple options. This can be applied to various business problems, such as supply chain optimization or resource allocation.
-
Simulation and Modeling: Creating simulations to test different scenarios and evaluate the potential impact of various actions. This allows for informed decision-making under uncertainty.
-
Decision Support Systems: Developing systems that provide decision-makers with real-time recommendations based on data analysis and predictive models.
Example: The retail company could use prescriptive analytics to optimize its pricing strategy, recommend personalized product recommendations to customers, or dynamically adjust its supply chain based on predicted demand fluctuations.
5. Cognitive and Autonomous Systems: Automating Processes
This is the most advanced level of the AI Ladder, involving the development and deployment of autonomous systems that can operate with minimal human intervention. This includes:
-
Robotics and Automation: Automating physical tasks through robotics and intelligent automation. This can range from simple robotic process automation (RPA) to more complex autonomous systems.
-
Natural Language Processing (NLP): Enabling machines to understand and respond to human language, enabling the development of chatbots, virtual assistants, and other intelligent interfaces.
-
Computer Vision: Enabling machines to “see” and interpret images and videos, used in applications like object detection, facial recognition, and image analysis.
-
Cognitive Automation: Combining multiple AI techniques to create highly autonomous systems capable of handling complex tasks.
Example: The retail company could use cognitive automation to automate customer service inquiries through intelligent chatbots, optimize warehouse operations using robotic systems, or leverage computer vision to automate inventory management and track product quality.
Navigating the Ladder: Considerations for Successful AI Implementation
Climbing IBM's AI Ladder is a journey, not a sprint. Several crucial factors contribute to a successful ascent:
-
Starting Small and Iterating: Begin with a small, well-defined project to gain experience and build momentum. Iterative development allows for continuous improvement and adaptation.
-
Focusing on Business Value: Align AI initiatives with clear business goals and measure their impact on key performance indicators.
-
Building a Strong Data Strategy: Data is the fuel for AI. A comprehensive data strategy is essential to ensure data quality, accessibility, and security.
-
Investing in Talent and Training: Developing internal expertise is crucial for long-term success. Invest in training programs and recruitment to build a skilled AI team.
-
Embracing Collaboration: Collaboration between IT, business units, and data scientists is essential for successful AI implementation.
-
Managing Risk and Ethical Considerations: Address potential risks associated with AI, including bias, fairness, and privacy.
Conclusion: Embracing the Power of IBM's AI Ladder
IBM's AI Ladder provides a practical and structured approach to AI adoption, allowing organizations to gradually build their AI capabilities and maximize their return on investment. By starting with foundational steps and progressively moving towards more advanced techniques, organizations can effectively navigate the complexities of AI and unlock its transformative potential. The key lies in understanding the nuances of each level, aligning AI initiatives with strategic business goals, and fostering a culture of data-driven decision-making. Following this framework ensures a sustainable and successful journey towards AI maturity.
Latest Posts
Related Post
Thank you for visiting our website which covers about Identify The Steps In Ibm's Ladder Approach To Ai . We hope the information provided has been useful to you. Feel free to contact us if you have any questions or need further assistance. See you next time and don't miss to bookmark.