Given The Table Of Values Below Which Of The Following
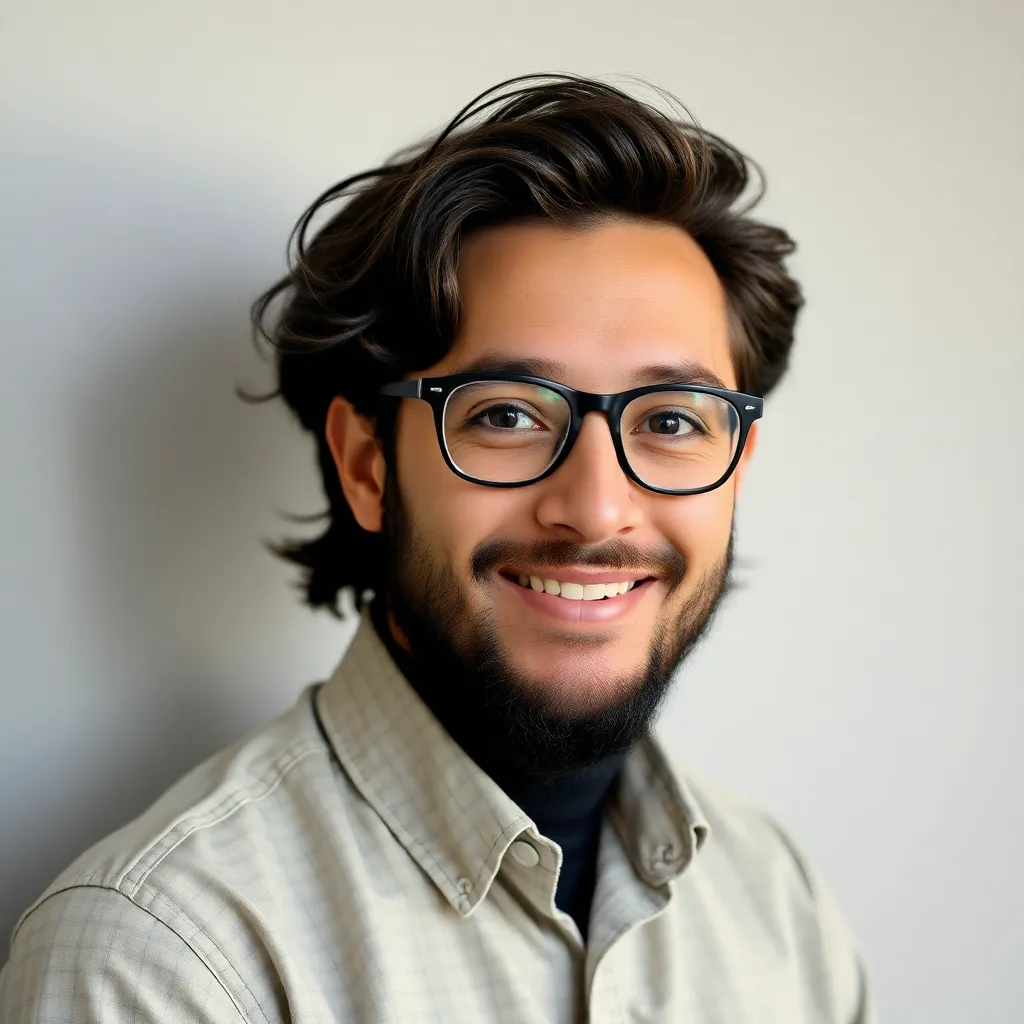
Holbox
May 11, 2025 · 5 min read
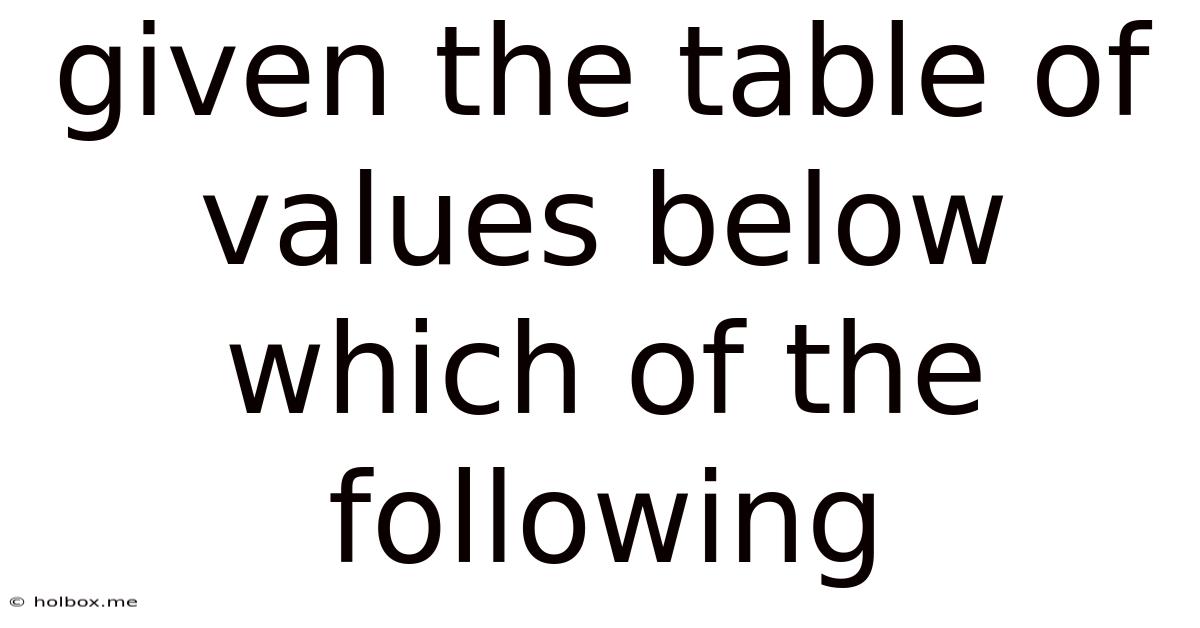
Table of Contents
- Given The Table Of Values Below Which Of The Following
- Table of Contents
- Analyzing Tabular Data: Interpreting Values and Drawing Conclusions
- Understanding the Context: The Foundation of Analysis
- 1. Identifying Variables:
- 2. Defining Units of Measurement:
- 3. Understanding the Data Source:
- Analyzing the Values: Techniques and Strategies
- 1. Descriptive Statistics:
- 2. Visualizations:
- 3. Identifying Trends and Patterns:
- 4. Correlation and Regression Analysis:
- Drawing Conclusions: Interpreting the Results
- 1. Answering the Research Question:
- 2. Supporting Conclusions with Evidence:
- 3. Acknowledging Limitations:
- 4. Communicating Findings Clearly:
- Example: Analyzing Sales Data
- Advanced Analytical Techniques
- Conclusion
- Latest Posts
- Related Post
Analyzing Tabular Data: Interpreting Values and Drawing Conclusions
Given a table of values, the process of analysis goes beyond simply looking at the numbers. It involves understanding the context of the data, identifying trends, drawing meaningful conclusions, and even making predictions. This article will delve into the intricacies of analyzing tabular data, providing a comprehensive guide for interpreting values and formulating accurate conclusions. We'll explore various analytical techniques and demonstrate how to effectively communicate findings.
Understanding the Context: The Foundation of Analysis
Before diving into the numbers, it's crucial to understand the context of the data presented in the table. This includes:
1. Identifying Variables:
- Independent Variable: This is the variable that is manipulated or changed. It's often represented in the rows or columns of the table.
- Dependent Variable: This is the variable that is measured or observed. It's affected by the changes in the independent variable.
- Control Variables: These are variables that are kept constant to ensure a fair comparison. Understanding which variables are controlled is critical for interpreting results accurately.
2. Defining Units of Measurement:
Clearly understanding the units used for each variable is essential. Are we dealing with kilograms, meters, dollars, percentages, or something else? Ambiguity in units can lead to misinterpretations.
3. Understanding the Data Source:
The source of the data is crucial for evaluating its reliability and validity. Is the data from a reputable source? What is the sample size? Is there any potential bias in the data collection method?
Analyzing the Values: Techniques and Strategies
Once the context is understood, we can begin analyzing the values within the table. Several techniques can be employed, depending on the type of data and the research questions:
1. Descriptive Statistics:
Descriptive statistics summarize the main features of the data. Key measures include:
- Mean (Average): The sum of all values divided by the number of values.
- Median: The middle value when the data is arranged in order.
- Mode: The most frequent value.
- Range: The difference between the highest and lowest values.
- Standard Deviation: A measure of the spread or dispersion of the data.
These statistics provide a concise summary of the central tendency and variability of the data.
2. Visualizations:
Visualizing the data through charts and graphs can greatly enhance understanding. Appropriate visualizations include:
- Bar Charts: Effective for comparing categorical data.
- Line Graphs: Ideal for showing trends over time.
- Scatter Plots: Useful for exploring relationships between two variables.
- Histograms: Show the distribution of numerical data.
Choosing the right visualization depends on the type of data and the message you want to convey.
3. Identifying Trends and Patterns:
Careful examination of the values can reveal trends and patterns. Look for:
- Increasing or decreasing trends: Are the values consistently increasing, decreasing, or fluctuating?
- Cycles or seasonality: Do the values repeat in a regular pattern?
- Correlations: Do changes in one variable seem to be related to changes in another variable?
- Outliers: Are there any unusually high or low values that might be errors or require further investigation?
4. Correlation and Regression Analysis:
If the data suggests a relationship between variables, correlation and regression analysis can help quantify this relationship.
- Correlation: Measures the strength and direction of the linear relationship between two variables.
- Regression: Models the relationship between a dependent variable and one or more independent variables, allowing for predictions.
Drawing Conclusions: Interpreting the Results
Once the data has been analyzed, the next step is to draw conclusions. This involves:
1. Answering the Research Question:
The conclusions should directly address the research question that prompted the analysis. What insights have been gained from the data?
2. Supporting Conclusions with Evidence:
Conclusions must be supported by the data. Refer to specific values, trends, and statistical measures to justify the conclusions.
3. Acknowledging Limitations:
It's important to acknowledge any limitations of the analysis. These might include:
- Small sample size: The conclusions might not be generalizable to a larger population.
- Data quality issues: Errors or biases in the data could affect the validity of the conclusions.
- Confounding variables: Uncontrolled variables could influence the results.
4. Communicating Findings Clearly:
The conclusions should be communicated clearly and concisely, using appropriate language and visualizations. Avoid technical jargon that the intended audience might not understand.
Example: Analyzing Sales Data
Let's consider a hypothetical example: a table showing monthly sales of a product over a year.
Month | Sales (Units) |
---|---|
January | 100 |
February | 120 |
March | 150 |
April | 180 |
May | 200 |
June | 220 |
July | 200 |
August | 180 |
September | 150 |
October | 120 |
November | 100 |
December | 150 |
By analyzing this data, we can observe:
- Overall trend: Sales generally increase from January to June, then decrease from July to November, with a slight increase in December. This suggests seasonality, potentially related to factors like weather or holidays.
- Peak sales: Peak sales occur in June, suggesting a potential marketing opportunity to capitalize on this period.
- Seasonal variation: A clear seasonal pattern is visible, with higher sales during the warmer months and lower sales during the colder months.
We could create a line graph to visualize this trend and further support our conclusions. The analysis could then inform marketing strategies, inventory management, and resource allocation for the coming year.
Advanced Analytical Techniques
For more complex datasets, advanced analytical techniques may be necessary:
- Time series analysis: For data collected over time, time series analysis can be used to identify trends, seasonality, and other patterns.
- Multivariate analysis: When dealing with multiple variables, multivariate analysis techniques can uncover relationships and dependencies between them.
- Machine learning: Machine learning algorithms can be used to identify patterns and make predictions from large datasets.
Conclusion
Analyzing tabular data is a crucial skill for making informed decisions in various fields. By understanding the context of the data, employing appropriate analytical techniques, and communicating findings clearly, we can extract valuable insights and use them to improve decision-making. Remember that thoroughness, accuracy, and clarity are paramount in ensuring the effectiveness of any data analysis. The process is iterative, requiring careful consideration and potential adjustments based on the evolving understanding of the data. Through meticulous examination and the application of the strategies outlined above, you can unlock the power of data and draw meaningful conclusions that have real-world applications.
Latest Posts
Related Post
Thank you for visiting our website which covers about Given The Table Of Values Below Which Of The Following . We hope the information provided has been useful to you. Feel free to contact us if you have any questions or need further assistance. See you next time and don't miss to bookmark.