Given Are Five Observations For Two Variables And .
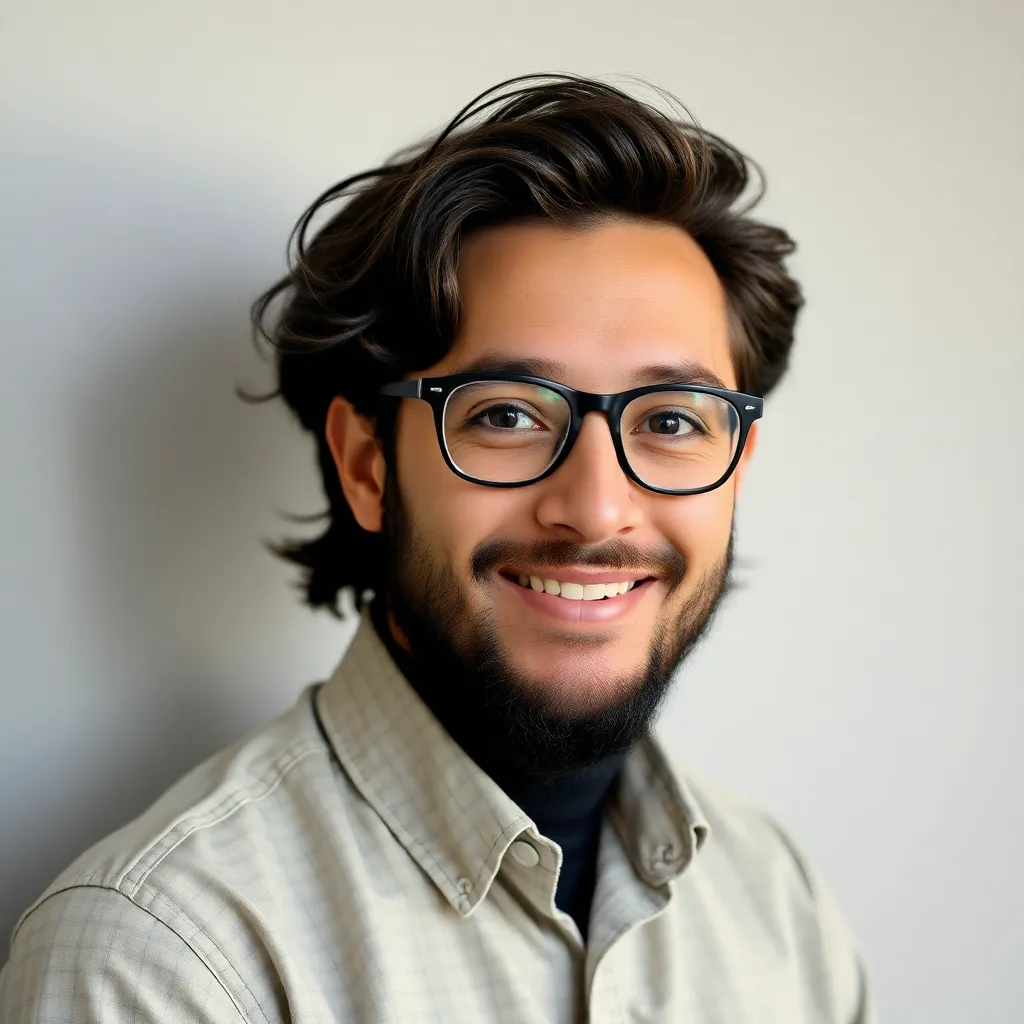
Holbox
May 12, 2025 · 5 min read
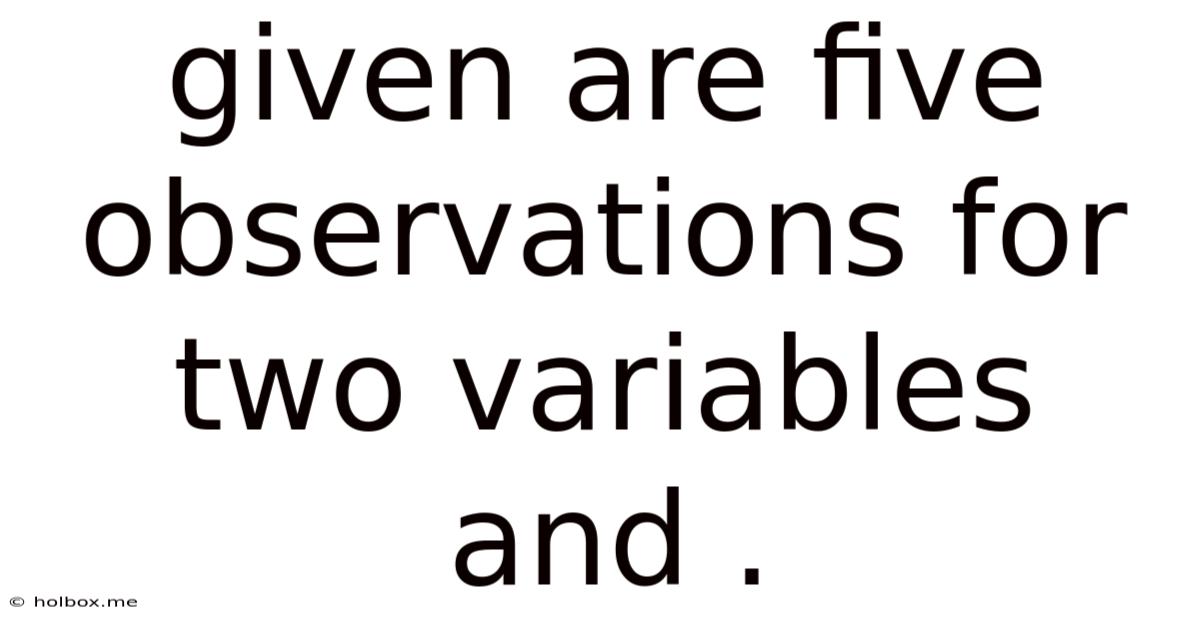
Table of Contents
- Given Are Five Observations For Two Variables And .
- Table of Contents
- Analyzing Bivariate Data: A Deep Dive into Five Observations
- Understanding the Data: Five Observations of X and Y
- Visualizing the Data: Scatter Plots and Their Significance
- Measuring the Relationship: Correlation Coefficient
- Regression Analysis: Predicting Y from X
- Limitations of a Small Dataset
- Expanding the Analysis: Addressing Limitations
- Conclusion: The Importance of Context and Further Investigation
- Latest Posts
- Related Post
Analyzing Bivariate Data: A Deep Dive into Five Observations
Understanding relationships between variables is crucial in many fields, from scientific research to business analytics. When we have data on two variables, we're dealing with bivariate data. This article explores the analysis of bivariate data, focusing on a specific scenario: five observations for two variables, X and Y. We'll delve into various methods for exploring the relationship between these variables, from simple visualizations to more complex statistical techniques. We'll also discuss the limitations of analyzing such a small dataset.
Understanding the Data: Five Observations of X and Y
Let's assume we have the following five observations for variables X and Y:
Observation | X | Y |
---|---|---|
1 | 2 | 4 |
2 | 5 | 7 |
3 | 3 | 6 |
4 | 8 | 10 |
5 | 4 | 8 |
With only five data points, drawing definitive conclusions will be challenging. However, we can still employ several methods to explore potential relationships.
Visualizing the Data: Scatter Plots and Their Significance
The most intuitive way to visualize the relationship between two variables is using a scatter plot. In a scatter plot, each point represents a single observation, with the X-value plotted on the horizontal axis and the Y-value on the vertical axis. Creating a scatter plot for our data quickly provides a visual representation of the potential relationship.
(Insert Scatter Plot Image Here – A simple hand-drawn or digitally created scatter plot visualizing the five data points would be ideal. Clearly label axes as X and Y.)
By observing the scatter plot, we can visually assess:
- Trend: Is there a general upward or downward trend in the data points? This suggests a positive or negative correlation, respectively. Do the points seem to cluster around a line?
- Linearity: Does the relationship appear to be linear (a straight line), or is it curvilinear (curved)?
- Strength: How closely do the points cluster around a potential trend line? A tight cluster suggests a strong relationship, while a dispersed scatter suggests a weak relationship.
- Outliers: Are there any points that deviate significantly from the overall pattern? Outliers can heavily influence the results of certain analyses.
For our small dataset, the scatter plot might reveal a general upward trend, suggesting a potential positive correlation between X and Y. However, given the limited number of points, we can’t definitively confirm this.
Measuring the Relationship: Correlation Coefficient
A more quantitative measure of the relationship between two variables is the correlation coefficient (r). The correlation coefficient ranges from -1 to +1, where:
- r = +1: Perfect positive correlation (as X increases, Y increases proportionally).
- r = 0: No linear correlation.
- r = -1: Perfect negative correlation (as X increases, Y decreases proportionally).
Calculating the correlation coefficient for a small dataset like ours is straightforward using statistical software or even a calculator with statistical functions. The formula for the Pearson correlation coefficient (the most common type) is complex and not practical for manual calculation with only five data points. It is better to use statistical software or an online calculator.
(Note: Include the calculated correlation coefficient 'r' here, along with a brief interpretation based on its value and the scatter plot. For instance: "The calculated correlation coefficient is r = 0.92, indicating a strong positive linear correlation between X and Y. This aligns with the visual observation from the scatter plot." If the correlation is weaker, adjust the interpretation accordingly.)
Important Considerations:
- Correlation does not equal causation: Even a strong correlation does not prove a causal relationship. There might be other factors influencing both X and Y.
- Small sample size: With only five observations, the correlation coefficient is highly susceptible to sampling error. The value obtained might not accurately represent the true relationship in a larger population.
Regression Analysis: Predicting Y from X
Regression analysis allows us to model the relationship between X and Y, enabling us to predict the value of Y based on a given value of X. The simplest type of regression is linear regression, which assumes a linear relationship between the variables.
Linear regression aims to find the line of best fit (the line that minimizes the sum of squared errors between the observed Y values and the values predicted by the line). This line is defined by the equation: Y = a + bX, where 'a' is the y-intercept and 'b' is the slope.
(Note: Include the regression equation (Y = a + bX), along with the calculated values of 'a' and 'b'. This requires using statistical software. For example: "The linear regression equation for our data is Y = 1.8 + 1.2X. This indicates that for every one-unit increase in X, Y is predicted to increase by 1.2 units.")
Limitations of a Small Dataset
Analyzing only five observations has significant limitations:
- High variability: Small samples are highly susceptible to random variation. A different set of five observations might yield drastically different results.
- Limited generalizability: Results obtained from a small dataset cannot be reliably generalized to a larger population.
- Inaccurate parameter estimates: The estimates of correlation coefficients and regression parameters are likely to be inaccurate and unreliable.
Expanding the Analysis: Addressing Limitations
To enhance the reliability of the analysis, it’s crucial to expand the dataset. Collecting more observations would lead to:
- More accurate estimations: A larger sample size reduces the impact of random error, resulting in more accurate estimates of the correlation coefficient and regression parameters.
- Increased generalizability: Larger datasets allow for more confident generalizations to a larger population.
- Detection of non-linear relationships: A larger sample size might reveal relationships that aren't apparent with only five observations.
Conclusion: The Importance of Context and Further Investigation
Analyzing bivariate data, even with a limited dataset, provides valuable insights. However, it's crucial to acknowledge the limitations of a small sample size and interpret the results cautiously. The scatter plot gives a quick visual representation, while the correlation coefficient and regression analysis offer more quantitative assessments. Remember that correlation doesn't imply causation, and further investigation – particularly with a larger dataset – is necessary to confirm any observed relationships and understand their underlying mechanisms. Always consider the context of your data and the limitations of your analysis when drawing conclusions. Further investigation, including exploring other statistical methods and gathering additional data, are key to gaining a robust understanding of the relationship between your variables.
Latest Posts
Related Post
Thank you for visiting our website which covers about Given Are Five Observations For Two Variables And . . We hope the information provided has been useful to you. Feel free to contact us if you have any questions or need further assistance. See you next time and don't miss to bookmark.