Forecasting Risk Is Defined As The Possibility That
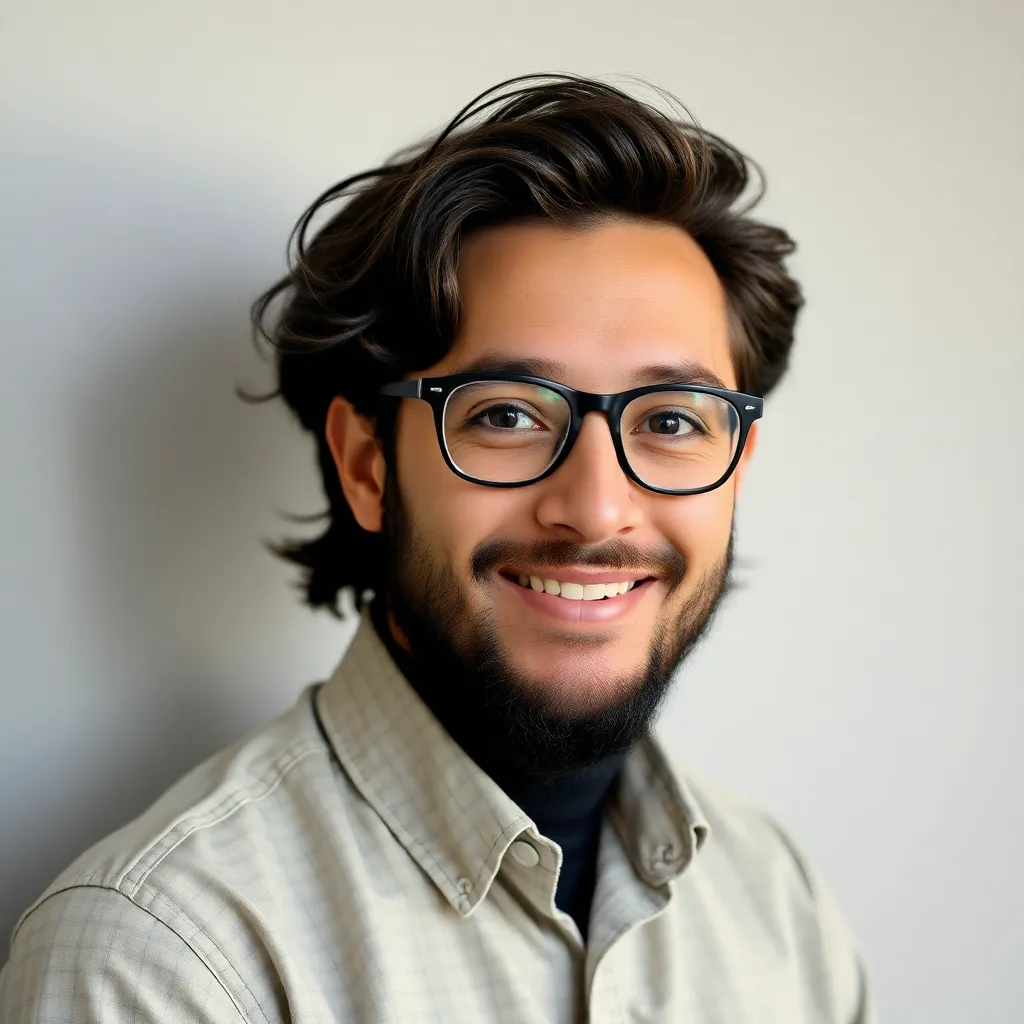
Holbox
May 10, 2025 · 6 min read
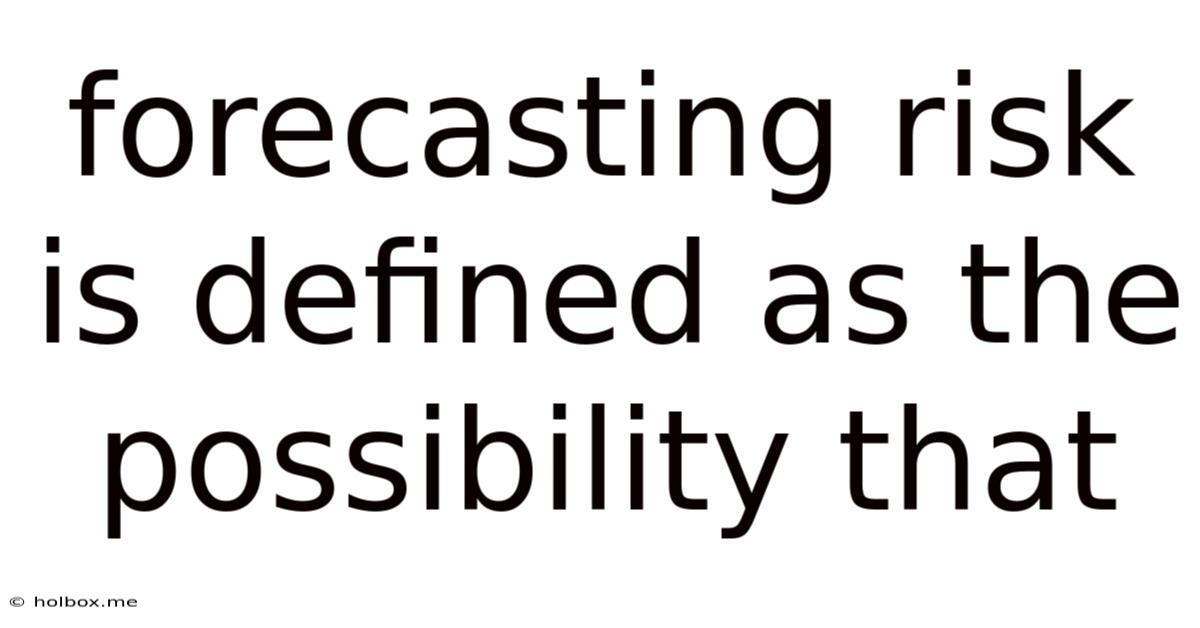
Table of Contents
- Forecasting Risk Is Defined As The Possibility That
- Table of Contents
- Forecasting Risk: Defined as the Possibility That... Your Predictions Are Wrong
- Understanding the Roots of Forecasting Risk
- 1. Data Limitations: The Foundation of Uncertainty
- 2. Model Limitations: The Assumptions We Make
- 3. Human Error: The Subjective Element
- Types of Forecasting Risk
- 1. Accuracy Risk: The Magnitude of the Error
- 2. Precision Risk: The Confidence Interval
- 3. Relevance Risk: The Utility of the Forecast
- 4. Timeliness Risk: The Time Sensitivity of the Information
- Mitigating Forecasting Risk: A Multi-pronged Approach
- 1. Enhancing Data Quality: The Foundation for Success
- 2. Optimizing Model Selection and Development: Choosing the Right Tool
- 3. Incorporating Expert Judgment: The Human Element
- 4. Fostering a Culture of Continuous Improvement: Learning from Mistakes
- The Impact of Forecasting Risk on Organizational Performance
- Conclusion: Embracing Uncertainty, Minimizing Risk
- Latest Posts
- Latest Posts
- Related Post
Forecasting Risk: Defined as the Possibility That... Your Predictions Are Wrong
Forecasting risk is defined as the possibility that your predictions about the future will be inaccurate. It's the inherent uncertainty associated with any attempt to anticipate future events, trends, or outcomes. While forecasting is a crucial element of strategic planning and decision-making across various fields, from finance and economics to marketing and supply chain management, understanding and managing the associated risks is paramount to success. This article delves deep into the multifaceted nature of forecasting risk, exploring its sources, types, mitigation strategies, and overall impact on organizational performance.
Understanding the Roots of Forecasting Risk
The core of forecasting risk lies in the simple fact that the future is inherently unpredictable. Numerous factors contribute to this uncertainty, making accurate prediction a challenging, albeit necessary, task. Let's examine some of the key sources:
1. Data Limitations: The Foundation of Uncertainty
Forecasting models, no matter how sophisticated, rely heavily on historical data. However, data limitations are pervasive and can significantly impact forecast accuracy. These limitations include:
- Incomplete Data: Missing data points create gaps in the historical record, hindering the model's ability to identify patterns and trends accurately.
- Inaccurate Data: Errors in data collection, recording, or entry can lead to skewed results and unreliable forecasts. Data quality is critical.
- Data Bias: Historical data may reflect past biases or anomalies that don't accurately represent future conditions. For example, a forecast based solely on a period of exceptional economic growth might be overly optimistic.
- Limited Data: For new products, services, or emerging markets, historical data might be scarce or non-existent, making forecasting significantly more challenging.
2. Model Limitations: The Assumptions We Make
Even with perfect data, the forecasting model itself introduces a degree of risk. Models are simplifications of reality, relying on assumptions that may not hold true in the future:
- Model Selection Bias: Choosing the wrong model for the specific situation can lead to inaccurate predictions. Different models are better suited to different types of data and forecasting problems.
- Parameter Uncertainty: The parameters within a model (e.g., coefficients in a regression model) are estimated from historical data and are subject to uncertainty. Small changes in these parameters can lead to significant variations in the forecast.
- Overfitting: A model that fits the historical data too closely may fail to generalize to future data, leading to poor predictive performance. This is a common problem with complex models.
- External Factors: Models often fail to account for unforeseen external events (e.g., natural disasters, geopolitical instability) that can drastically alter the forecast.
3. Human Error: The Subjective Element
Human judgment plays a significant role in the forecasting process, from data selection and model building to interpretation and communication of results. This introduces another layer of risk:
- Bias: Forecasting can be influenced by personal biases, cognitive biases, and confirmation bias (seeking out information that confirms pre-existing beliefs).
- Subjectivity: Interpreting forecast results involves subjective judgments, which can vary between individuals.
- Communication Errors: Miscommunication of forecast assumptions, limitations, and results can lead to misinterpretations and poor decision-making.
Types of Forecasting Risk
Forecasting risks manifest in different ways, impacting various aspects of decision-making. Understanding these distinct types helps in developing targeted mitigation strategies.
1. Accuracy Risk: The Magnitude of the Error
This is the most straightforward type of forecasting risk, referring to the potential for the forecast to be significantly different from the actual outcome. A higher accuracy risk implies a larger potential for error, leading to suboptimal decisions.
2. Precision Risk: The Confidence Interval
Precision risk focuses on the uncertainty associated with the forecast. A precise forecast has a narrow confidence interval, indicating high confidence in the prediction. Conversely, a less precise forecast has a wider confidence interval, reflecting greater uncertainty.
3. Relevance Risk: The Utility of the Forecast
Relevance risk refers to the extent to which the forecast provides useful information for decision-making. A forecast, even if accurate and precise, may be irrelevant if it doesn't address the key questions or concerns.
4. Timeliness Risk: The Time Sensitivity of the Information
Timeliness risk arises when the forecast is not available in a timely manner to inform decision-making. For decisions with short lead times, timely forecasts are crucial. Delays can render the forecast obsolete.
Mitigating Forecasting Risk: A Multi-pronged Approach
Managing forecasting risk involves a combination of strategies aimed at improving data quality, enhancing model selection, incorporating expert judgment, and fostering a culture of continuous improvement.
1. Enhancing Data Quality: The Foundation for Success
- Data Cleansing: Identify and correct errors, inconsistencies, and missing values in the data.
- Data Validation: Implement processes to ensure data accuracy and reliability.
- Data Augmentation: Supplement existing data with alternative data sources to improve coverage and reduce bias.
2. Optimizing Model Selection and Development: Choosing the Right Tool
- Model Selection: Choose the most appropriate forecasting model for the specific data and forecasting problem.
- Model Validation: Thoroughly test the model's performance using appropriate validation techniques (e.g., cross-validation).
- Model Calibration: Adjust model parameters to improve accuracy and reduce bias.
- Ensemble Methods: Combine predictions from multiple models to improve overall forecast accuracy.
3. Incorporating Expert Judgment: The Human Element
- Expert Elicitation: Systematically gather expert opinions to incorporate qualitative information and insights not captured in the data.
- Scenario Planning: Develop alternative scenarios to account for potential unforeseen events and their impact on the forecast.
- Delphi Method: Use an iterative process to reach consensus among experts.
4. Fostering a Culture of Continuous Improvement: Learning from Mistakes
- Monitoring and Evaluation: Regularly monitor forecast performance and compare it to actual outcomes.
- Feedback Mechanisms: Establish feedback loops to identify areas for improvement and adjust forecasting processes accordingly.
- Documentation: Maintain detailed records of the forecasting process, assumptions, and results for future reference.
The Impact of Forecasting Risk on Organizational Performance
Poor forecasting can have significant consequences across various aspects of organizational performance:
- Financial Losses: Inaccurate demand forecasts can lead to overstocking, lost sales, and reduced profitability.
- Operational Inefficiencies: Poor production planning and scheduling stemming from inaccurate forecasts can result in increased costs and delays.
- Reputational Damage: Failing to meet customer expectations due to poor forecasting can damage the organization's reputation and erode customer loyalty.
- Strategic Misalignment: Inaccurate long-term forecasts can lead to strategic misalignment, impacting the overall direction and success of the organization.
Conclusion: Embracing Uncertainty, Minimizing Risk
Forecasting risk is an inherent aspect of predicting the future. While eliminating risk entirely is impossible, organizations can significantly mitigate its impact by focusing on data quality, model selection, expert judgment, and continuous improvement. By proactively addressing these factors, businesses can improve the accuracy, precision, and relevance of their forecasts, leading to better decision-making and improved organizational performance. Understanding and managing forecasting risk is not just a best practice; it's a necessity for sustainable growth and success in an increasingly uncertain world. The proactive management of forecasting risk ultimately translates to increased resilience, improved resource allocation, and a stronger competitive advantage.
Latest Posts
Latest Posts
-
How Tall Is 130 Cm In Feet
May 21, 2025
-
How Much Is 83 Kg In Stones
May 21, 2025
-
183 Cm To Inches And Feet
May 21, 2025
-
22 Lbs Is How Many Kg
May 21, 2025
-
122 Cm To Feet And Inches
May 21, 2025
Related Post
Thank you for visiting our website which covers about Forecasting Risk Is Defined As The Possibility That . We hope the information provided has been useful to you. Feel free to contact us if you have any questions or need further assistance. See you next time and don't miss to bookmark.