Forecasting Risk Is Best Defined As
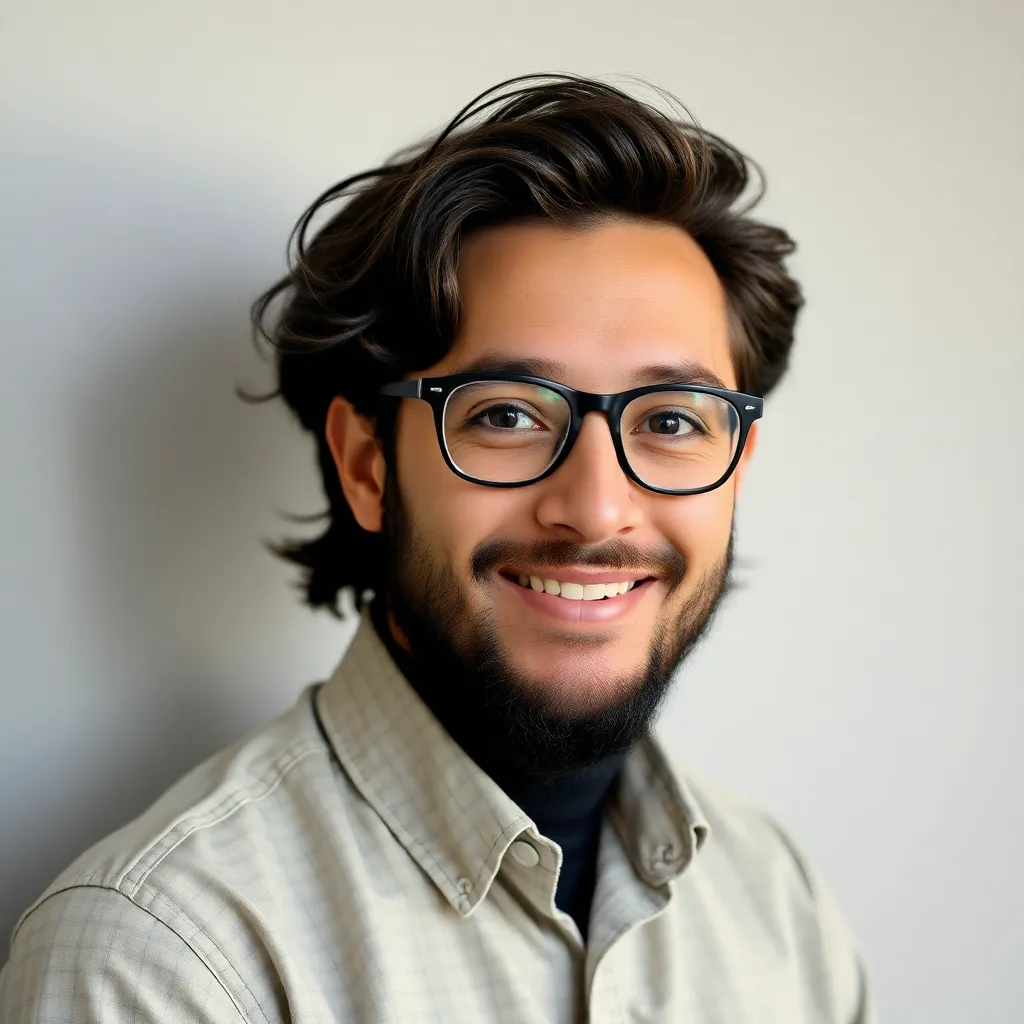
Holbox
May 11, 2025 · 6 min read
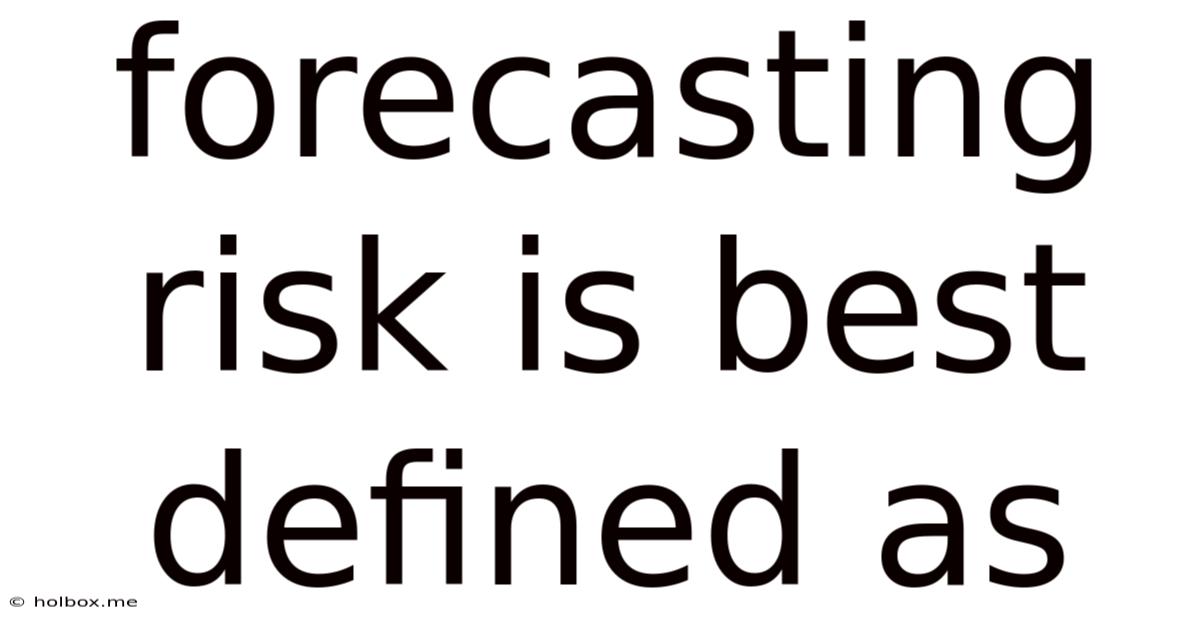
Table of Contents
- Forecasting Risk Is Best Defined As
- Table of Contents
- Forecasting Risk: A Comprehensive Guide to Understanding and Mitigating Uncertainty
- Defining and Understanding Forecasting Risk
- Types of Forecasting Risks
- Sources of Forecasting Risk
- Mitigating Forecasting Risk: Strategies and Techniques
- 1. Data Quality Improvement:
- 2. Model Selection and Validation:
- 3. Scenario Planning and Sensitivity Analysis:
- 4. Incorporating Expert Judgment:
- 5. Monitoring and Adaptation:
- 6. Communication and Transparency:
- Specific Examples of Forecasting Risk Across Industries
- Conclusion: Embracing Uncertainty in Forecasting
- Latest Posts
- Latest Posts
- Related Post
Forecasting Risk: A Comprehensive Guide to Understanding and Mitigating Uncertainty
Forecasting is an essential element of effective decision-making across various fields, from business and finance to weather prediction and public health. However, inherent in the nature of forecasting is risk. Forecasting risk is best defined as the potential for error in predictions, leading to suboptimal decisions and potentially negative consequences. Understanding and mitigating this risk is crucial for making informed choices and achieving desired outcomes. This comprehensive guide delves deep into the multifaceted nature of forecasting risk, exploring its various dimensions, sources, and effective mitigation strategies.
Defining and Understanding Forecasting Risk
Forecasting risk isn't simply about the possibility of an inaccurate prediction. It encompasses the broader implications of those inaccuracies. It's about the potential impact of errors on the decisions made based on those forecasts. This impact can manifest in several ways, including:
- Financial losses: Incorrect sales forecasts, for example, can lead to overstocking or understocking, resulting in lost revenue or increased storage costs.
- Missed opportunities: Underestimating market growth can cause a company to miss out on crucial expansion opportunities.
- Reputational damage: Inaccurate predictions, especially in areas like public health or environmental forecasting, can severely damage an organization's credibility.
- Operational inefficiencies: Poor resource allocation due to flawed forecasts can lead to operational bottlenecks and decreased productivity.
Types of Forecasting Risks
Forecasting risk can be categorized in several ways, depending on the source and nature of the error:
-
Model Risk: This refers to the risk associated with the choice and application of the forecasting model itself. An inappropriate model, or one misapplied, can lead to inaccurate predictions regardless of the data quality. This includes risks associated with simplifying complex systems, ignoring relevant variables or including irrelevant ones, and using inaccurate parameters within the model.
-
Data Risk: This encompasses risks related to the quality and availability of the data used to build the forecast. Inaccurate, incomplete, or outdated data will inevitably lead to unreliable predictions. Outliers in datasets, missing data points, and data biases can all contribute significantly to this type of risk.
-
Parameter Risk: Many forecasting models rely on parameters that need to be estimated from historical data. Uncertainty in the estimation of these parameters can lead to significant variations in forecasts. This is particularly relevant in statistical models where parameter uncertainty is a direct contributor to forecast error.
-
Scenario Risk: This refers to the risk of unforeseen events or "black swan" events significantly impacting the forecast's accuracy. These are typically events that are difficult or impossible to predict using historical data, such as natural disasters, political upheavals, or unexpected technological breakthroughs.
Sources of Forecasting Risk
Understanding the sources of forecasting risk is critical for developing effective mitigation strategies. Key contributors include:
-
Limited Historical Data: Accurately forecasting future trends often requires substantial historical data. When data is scarce, the forecast becomes inherently more uncertain. New businesses or those operating in rapidly evolving markets often face this challenge.
-
Inaccurate Data: The accuracy of a forecast is only as good as the data used to create it. Errors in data collection, processing, or entry can dramatically skew predictions. Biases in data collection methods are also a major concern.
-
Unforeseen Events: External factors beyond the control of the forecaster can significantly impact the accuracy of a prediction. These include economic downturns, changes in government regulations, or unexpected technological advancements.
-
Model Limitations: All forecasting models are simplifications of reality. They make assumptions about the underlying processes being modeled, and these assumptions might not always hold true. This inherent limitation contributes to forecasting risk.
-
Human Error: Subjective biases, errors in judgment, and inadequate expertise can all lead to inaccurate forecasts. This is particularly relevant when human judgment is incorporated into the forecasting process.
Mitigating Forecasting Risk: Strategies and Techniques
Effectively mitigating forecasting risk requires a multi-pronged approach:
1. Data Quality Improvement:
- Data Cleansing: Implementing rigorous data cleansing processes to identify and correct errors, inconsistencies, and outliers in the dataset.
- Data Validation: Establishing robust procedures to verify the accuracy and reliability of data sources.
- Data Augmentation: Employing techniques to supplement existing data with additional information, such as surveys, expert opinions, or external datasets.
2. Model Selection and Validation:
- Model Appropriateness: Selecting forecasting models that are appropriate for the specific data and the nature of the phenomenon being predicted.
- Model Validation: Rigorously testing the model's accuracy and robustness using techniques such as backtesting, cross-validation, and out-of-sample testing.
- Ensemble Methods: Combining multiple forecasting models to improve prediction accuracy and reduce the impact of individual model errors.
3. Scenario Planning and Sensitivity Analysis:
- Developing multiple scenarios: Creating multiple forecasts based on different assumptions about future conditions. This helps to identify potential risks and opportunities under various scenarios.
- Sensitivity analysis: Assessing how sensitive the forecast is to changes in key input variables. This allows for a better understanding of the uncertainty associated with the prediction.
4. Incorporating Expert Judgment:
- Structured Expert Elicitation: Using techniques to systematically gather and integrate the knowledge and experience of domain experts into the forecasting process. This can be crucial for incorporating qualitative factors that are difficult to quantify.
5. Monitoring and Adaptation:
- Continuous Monitoring: Regularly monitoring the forecast's accuracy and updating the model as new data becomes available.
- Adaptive Forecasting: Employing techniques that allow the model to automatically adjust to changing conditions and new information.
6. Communication and Transparency:
- Communicating Uncertainty: Clearly communicating the inherent uncertainty associated with the forecast to decision-makers. This helps to manage expectations and avoid overconfidence in the prediction.
- Transparency in Methodology: Clearly outlining the methodology used to create the forecast, including the data sources, model assumptions, and limitations.
Specific Examples of Forecasting Risk Across Industries
Let's examine how forecasting risk manifests in different sectors:
Finance: Inaccurate financial forecasts can lead to significant investment losses, inaccurate pricing of assets, and flawed risk management strategies. Underestimating credit risk can result in defaults and significant financial losses for lenders.
Supply Chain Management: Poor demand forecasting can lead to stockouts or excessive inventory, resulting in lost sales or increased storage costs. Disruptions in the supply chain due to unforeseen events can severely impact production and profitability.
Healthcare: Inaccurate disease outbreak predictions can lead to inadequate resource allocation, resulting in increased morbidity and mortality. Underestimating the demand for medical services can overwhelm healthcare systems.
Energy: Inaccurate energy demand forecasts can lead to power shortages or inefficient energy production. Inaccurate weather forecasting can severely impact renewable energy generation.
Agriculture: Poor yield forecasts can lead to inadequate resource allocation, resulting in decreased crop production and economic losses for farmers.
Conclusion: Embracing Uncertainty in Forecasting
Forecasting risk is an inherent part of the forecasting process. It cannot be completely eliminated, but it can be effectively managed. By understanding the various dimensions of forecasting risk, its sources, and the available mitigation strategies, organizations can significantly improve the accuracy of their predictions and make more informed decisions. A proactive and multifaceted approach that incorporates robust data management, appropriate model selection, scenario planning, and effective communication is crucial for minimizing the impact of forecasting risk and maximizing the value of forecasting in decision-making. The key is to embrace uncertainty, not avoid it, and to develop strategies that allow for flexibility and adaptation in the face of unforeseen events. Continuous learning and improvement are essential components of effective forecasting risk management.
Latest Posts
Latest Posts
-
How Tall Is 130 Cm In Feet
May 21, 2025
-
How Much Is 83 Kg In Stones
May 21, 2025
-
183 Cm To Inches And Feet
May 21, 2025
-
22 Lbs Is How Many Kg
May 21, 2025
-
122 Cm To Feet And Inches
May 21, 2025
Related Post
Thank you for visiting our website which covers about Forecasting Risk Is Best Defined As . We hope the information provided has been useful to you. Feel free to contact us if you have any questions or need further assistance. See you next time and don't miss to bookmark.