Difference Between Within Group And Between Group Variance
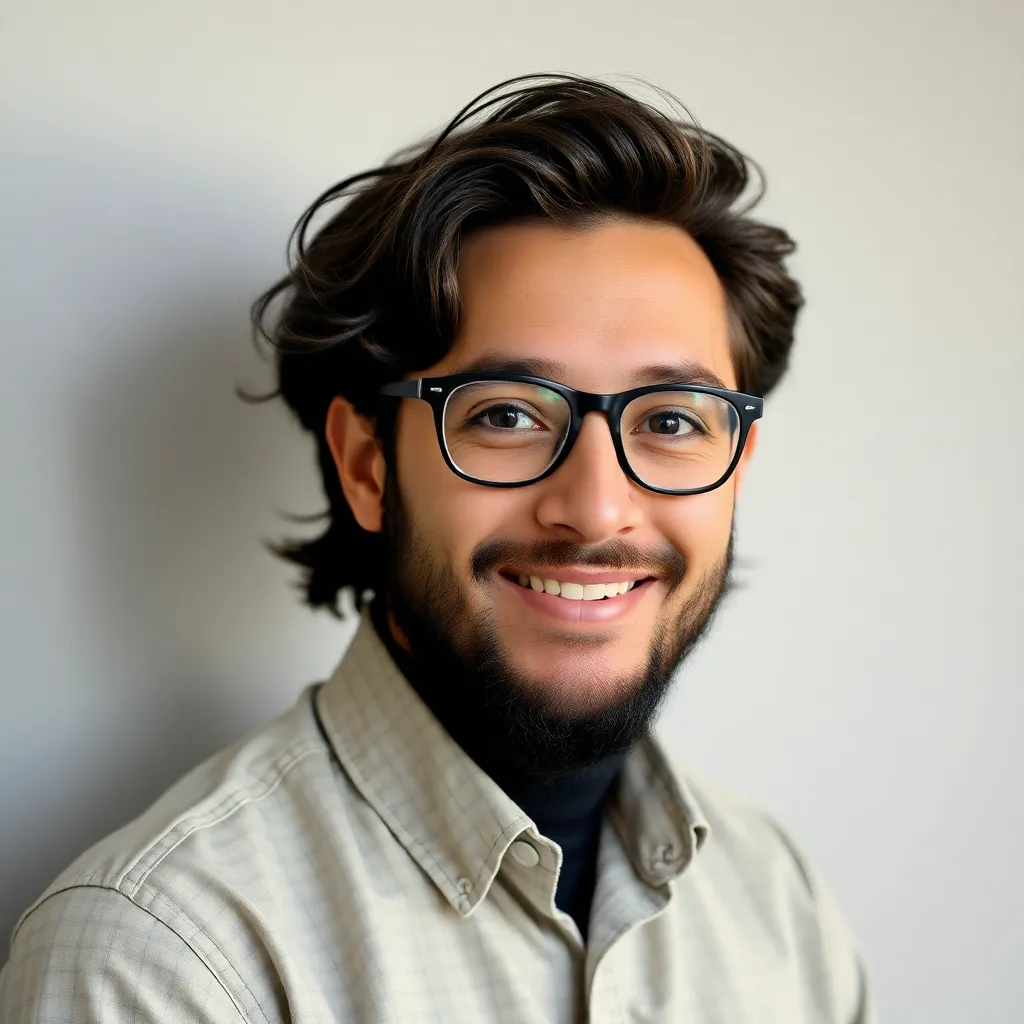
Holbox
Apr 02, 2025 · 6 min read

Table of Contents
- Difference Between Within Group And Between Group Variance
- Table of Contents
- Delving Deep into the Difference Between Within-Group and Between-Group Variance
- What is Variance? A Quick Refresher
- Within-Group Variance: The Variability Within
- Between-Group Variance: The Variability Between
- The Crucial Ratio: F-Statistic and ANOVA
- Visualizing the Differences: Box Plots
- Beyond ANOVA: Applications in Other Statistical Techniques
- Practical Considerations and Interpreting Results
- Conclusion: A Foundation for Statistical Inference
- Latest Posts
- Latest Posts
- Related Post
Delving Deep into the Difference Between Within-Group and Between-Group Variance
Understanding the difference between within-group and between-group variance is fundamental to many statistical analyses, particularly those involving Analysis of Variance (ANOVA) and other comparative methods. While seemingly complex, grasping these concepts unlocks a powerful understanding of data variability and its implications for drawing meaningful conclusions. This article will provide a comprehensive explanation, using clear examples and analogies to illuminate the nuances of each variance type and their crucial role in statistical inference.
What is Variance? A Quick Refresher
Before diving into the specifics of within-group and between-group variance, let's briefly review the concept of variance itself. Variance is a statistical measure that quantifies the spread or dispersion of a set of data points around their mean (average). A high variance indicates that the data points are widely scattered, while a low variance suggests that they are clustered closely around the mean. It's calculated as the average of the squared differences from the mean.
Formula for Variance: ∑(xᵢ - μ)² / N
Where:
- xᵢ represents each individual data point
- μ represents the mean of the data set
- N represents the total number of data points
Within-Group Variance: The Variability Within
Within-group variance, also known as error variance or intra-group variance, measures the variability within individual groups or categories of data. It reflects the natural scatter or randomness inherent in the data points belonging to the same group. Imagine you're measuring the height of students in three different classes. Within-group variance would represent the variation in height within each individual class. Some students in each class will be taller, some shorter, irrespective of the overall average height of the class.
Key Characteristics of Within-Group Variance:
- Reflects Randomness: Primarily captures the inherent variability unrelated to the grouping factor.
- Smaller is Better: A smaller within-group variance indicates that the data points within each group are more homogenous and clustered tightly around their respective group means.
- Used for Comparison: It serves as a benchmark for comparing the variability between groups.
Example: Consider a study comparing the effectiveness of three different fertilizers on plant growth. Within-group variance would represent the variation in plant height within each fertilizer group. Some plants treated with the same fertilizer will grow taller or shorter than others due to factors like slight variations in soil conditions, sunlight exposure, or inherent genetic differences. A lower within-group variance suggests that the fertilizer is highly consistent in its effect on plant growth, leading to less variability in plant heights within each treatment group.
Between-Group Variance: The Variability Between
Between-group variance, also known as inter-group variance, measures the variability between different groups or categories of data. It reflects the differences in the average values (means) of the different groups. In our height example, between-group variance would measure the difference in average heights between the three classes. One class might have a significantly taller average height than the other two.
Key Characteristics of Between-Group Variance:
- Reflects Group Differences: Primarily captures the differences in the means of different groups.
- Larger is Better (in the context of a significant effect): A larger between-group variance, relative to within-group variance, suggests that the grouping factor has a significant impact on the dependent variable. This indicates that the differences between group means are substantial compared to the natural variability within each group.
- Crucial for Hypothesis Testing: The ratio of between-group variance to within-group variance forms the basis of many statistical tests, like ANOVA, to determine if the observed differences between group means are statistically significant or merely due to chance.
Example: Returning to the fertilizer example, between-group variance would represent the difference in average plant heights between the three fertilizer groups. If one fertilizer leads to significantly taller plants on average compared to the others, the between-group variance would be high. This suggests that the type of fertilizer has a substantial effect on plant growth.
The Crucial Ratio: F-Statistic and ANOVA
The power of understanding within-group and between-group variance becomes truly evident when we consider their ratio in the context of ANOVA. ANOVA, or Analysis of Variance, is a statistical test that uses this ratio to determine whether there are statistically significant differences between the means of two or more groups.
The F-statistic, a central component of ANOVA, is calculated as the ratio of between-group variance to within-group variance:
F-statistic = Between-Group Variance / Within-Group Variance
-
A high F-statistic suggests that the between-group variance is significantly larger than the within-group variance. This implies that the differences between the group means are substantial and unlikely to be due to random chance. In this case, we would reject the null hypothesis (which typically states that there are no significant differences between group means).
-
A low F-statistic suggests that the between-group variance is not significantly larger than the within-group variance. This implies that the observed differences between group means might be due to random chance, and we would likely fail to reject the null hypothesis.
Therefore, the F-statistic provides a quantifiable measure of the extent to which differences between groups outweigh the natural variability within groups. This is critical for making sound conclusions about the effect of the independent variable (the grouping factor) on the dependent variable (the measured outcome).
Visualizing the Differences: Box Plots
Box plots offer an excellent visual representation of within-group and between-group variance. Each box represents a group, showing the median, quartiles, and potential outliers. The length of the box indicates the interquartile range (IQR), a measure of within-group variability. The distance between the medians of different boxes represents the between-group variability.
Beyond ANOVA: Applications in Other Statistical Techniques
The concepts of within-group and between-group variance extend beyond ANOVA and are fundamental to various other statistical techniques, including:
- MANOVA (Multivariate Analysis of Variance): Extends ANOVA to handle multiple dependent variables simultaneously.
- ANCOVA (Analysis of Covariance): Includes covariates (control variables) to account for additional sources of variation.
- Repeated Measures ANOVA: Analyzes data where the same subjects are measured multiple times.
- Mixed Models: Account for both fixed and random effects in the data.
Practical Considerations and Interpreting Results
Analyzing within-group and between-group variance requires careful consideration of several factors:
- Sample Size: Sufficient sample size in each group is crucial for accurate estimation of variances.
- Data Distribution: Assumptions about the distribution of data (e.g., normality) may be necessary for certain statistical tests.
- Outliers: Outliers can disproportionately influence variance estimates. Identifying and handling outliers appropriately is essential.
- Effect Size: While statistical significance indicates that differences between groups are unlikely due to chance, effect size measures the practical significance or magnitude of the observed differences.
Conclusion: A Foundation for Statistical Inference
Understanding the distinction between within-group and between-group variance is paramount for effective statistical analysis. These concepts are not merely theoretical constructs; they are the building blocks of many powerful statistical techniques used to draw meaningful conclusions from data. By carefully considering the variability within and between groups, researchers can confidently assess the impact of various factors and make informed decisions based on empirical evidence. Mastering these concepts unlocks a deeper understanding of data analysis and enhances the ability to interpret research findings accurately. Remember that the interplay between these variances is crucial for robust statistical inference, providing a pathway to reliable and meaningful conclusions in diverse fields of study.
Latest Posts
Latest Posts
-
Bob Golomb Attributes His Success As A Car Salesmen To
Apr 09, 2025
-
A Nurse Is Caring For A Preschooler Who Has Neutropenia
Apr 09, 2025
-
Accounting Profits Equal Total Revenue Minus
Apr 09, 2025
-
Angela And Carlos Are Asked To Determine The Relationship
Apr 09, 2025
-
In A Web App Where Is Data Usually Stored
Apr 09, 2025
Related Post
Thank you for visiting our website which covers about Difference Between Within Group And Between Group Variance . We hope the information provided has been useful to you. Feel free to contact us if you have any questions or need further assistance. See you next time and don't miss to bookmark.