Consider A Binomial Experiment With And
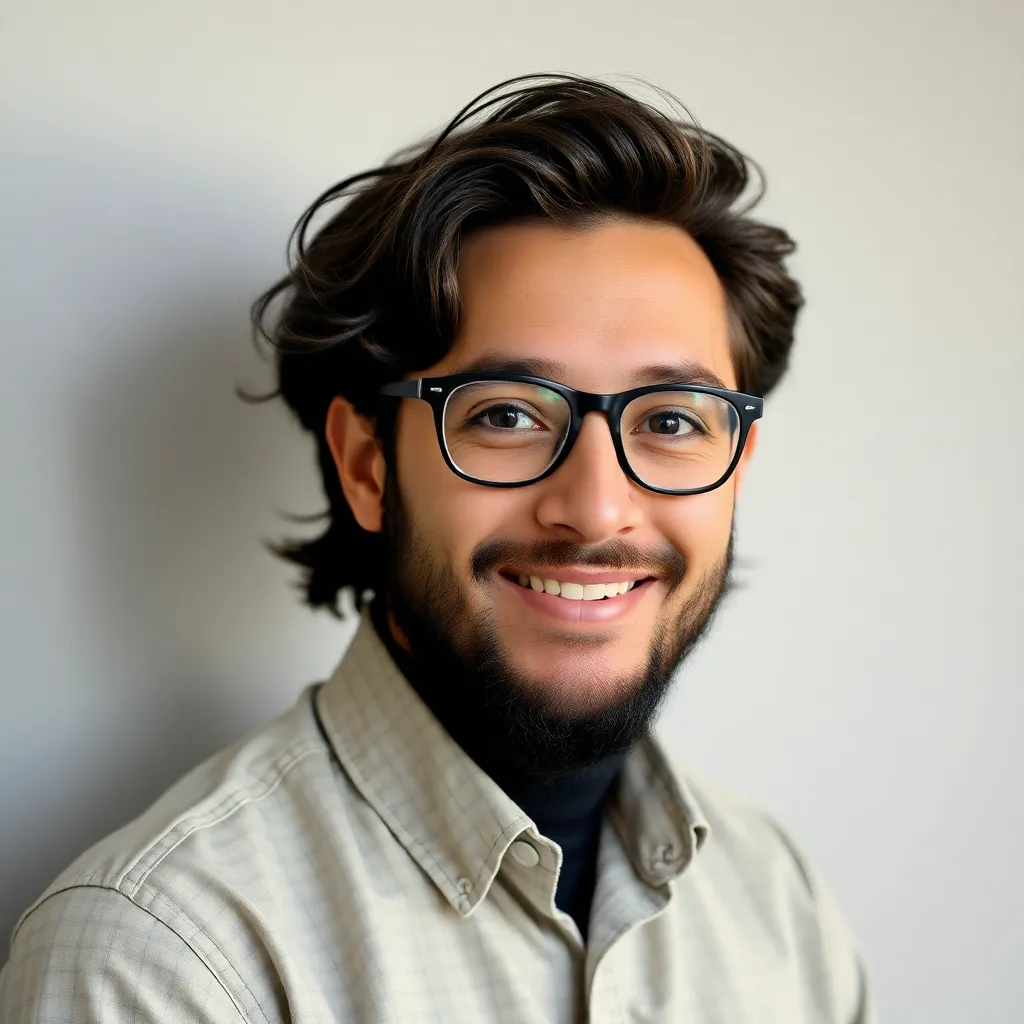
Holbox
Mar 22, 2025 · 6 min read
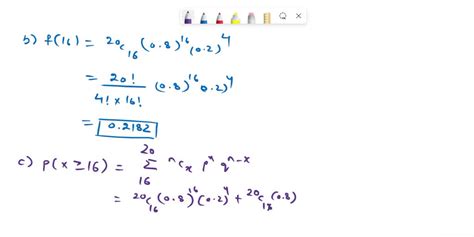
Table of Contents
- Consider A Binomial Experiment With And
- Table of Contents
- Delving Deep into Binomial Experiments: Understanding Probability and Applications
- Understanding the Binomial Experiment's Core Principles
- Examples of Binomial Experiments
- Key Formulas in Binomial Probability
- 1. Probability Mass Function (PMF)
- 2. Cumulative Distribution Function (CDF)
- 3. Mean (Expected Value)
- 4. Variance and Standard Deviation
- Applying Binomial Probability in Real-World Scenarios
- 1. Business and Marketing
- 2. Healthcare and Medicine
- 3. Quality Control and Manufacturing
- 4. Genetics and Biology
- Interpreting Binomial Probabilities and Drawing Conclusions
- Limitations of Binomial Experiments
- Beyond the Basics: Advanced Concepts
- 1. The Normal Approximation to the Binomial
- 2. Confidence Intervals for Binomial Proportions
- 3. Hypothesis Testing with Binomial Data
- Conclusion
- Latest Posts
- Related Post
Delving Deep into Binomial Experiments: Understanding Probability and Applications
The binomial experiment, a cornerstone of probability theory, provides a powerful framework for analyzing situations involving repeated, independent trials with only two possible outcomes. This article will delve into the intricacies of binomial experiments, exploring their underlying assumptions, key formulas, practical applications, and limitations. We’ll move beyond the basics, examining how to interpret results and apply this knowledge to real-world scenarios.
Understanding the Binomial Experiment's Core Principles
A binomial experiment is characterized by the following crucial features:
-
Fixed Number of Trials (n): The experiment consists of a predetermined number of trials, denoted by 'n'. This number remains constant throughout the experiment.
-
Independent Trials: The outcome of each trial does not affect the outcome of any other trial. This independence is a critical assumption.
-
Two Possible Outcomes: Each trial results in one of two mutually exclusive outcomes: success (often denoted by 'p') or failure (often denoted by 'q').
-
Constant Probability of Success: The probability of success ('p') remains constant for every trial. The probability of failure ('q') is simply 1 - p.
These four conditions must be met for an experiment to be considered truly binomial. Any deviation from these principles can significantly impact the validity of the analysis.
Examples of Binomial Experiments
Let's illustrate with some examples:
-
Coin Toss: Flipping a fair coin 10 times. 'Success' could be getting heads (p = 0.5), and 'failure' would be getting tails (q = 0.5).
-
Quality Control: Inspecting 20 randomly selected products from an assembly line. 'Success' could be finding a defective product (p = let's say 0.05, if 5% are defective), and 'failure' would be finding a non-defective product.
-
Medical Trials: Testing a new drug on 50 patients. 'Success' could be observing a positive response to the drug, while 'failure' would be a lack of response or adverse effects.
-
Surveys: Asking 100 randomly selected people whether they support a particular policy. 'Success' could be a 'yes' response, and 'failure' a 'no' response.
Key Formulas in Binomial Probability
The core of binomial analysis lies in understanding and applying these key formulas:
1. Probability Mass Function (PMF)
The PMF calculates the probability of getting exactly 'k' successes in 'n' trials:
P(X = k) = (nCk) * p^k * q^(n-k)
Where:
- P(X = k): Probability of exactly k successes.
- nCk: The binomial coefficient, representing the number of ways to choose k successes from n trials (n! / (k! * (n-k)!)).
- p: Probability of success in a single trial.
- q: Probability of failure in a single trial (1 - p).
- k: The number of successes.
- n: The total number of trials.
2. Cumulative Distribution Function (CDF)
The CDF calculates the probability of getting up to 'k' successes in 'n' trials:
P(X ≤ k) = Σ (from i=0 to k) [ (nCi) * p^i * q^(n-i) ]
This involves summing the probabilities of getting 0, 1, 2,... up to k successes.
3. Mean (Expected Value)
The mean or expected value (μ) of a binomial distribution represents the average number of successes expected over many repetitions of the experiment:
μ = n * p
4. Variance and Standard Deviation
The variance (σ²) measures the spread or dispersion of the binomial distribution, while the standard deviation (σ) is the square root of the variance:
σ² = n * p * q
σ = √(n * p * q)
Applying Binomial Probability in Real-World Scenarios
The power of binomial experiments lies in their diverse applications across various fields:
1. Business and Marketing
-
A/B Testing: Comparing the effectiveness of two different marketing campaigns by randomly assigning customers to each group and analyzing the conversion rates (successes).
-
Predictive Modeling: Estimating the likelihood of customer churn or product defects based on historical data and assumptions about the probability of success (customer retention or non-defective products).
2. Healthcare and Medicine
-
Clinical Trials: Assessing the efficacy of new drugs or treatments by measuring the success rate (positive patient outcomes) in a controlled experimental setting.
-
Epidemiology: Modeling the spread of infectious diseases by analyzing the probability of infection and transmission in a population.
3. Quality Control and Manufacturing
-
Acceptance Sampling: Determining whether to accept or reject a batch of products based on the number of defects found in a random sample.
-
Reliability Analysis: Evaluating the reliability of components or systems by determining the probability of failure within a specified timeframe.
4. Genetics and Biology
-
Hardy-Weinberg Equilibrium: Analyzing the genetic makeup of populations to determine whether allele frequencies are stable over time, assuming random mating and other binomial assumptions.
-
Genetic Inheritance: Predicting the probability of offspring inheriting specific traits based on the genotypes of their parents.
Interpreting Binomial Probabilities and Drawing Conclusions
Interpreting the results of a binomial experiment involves assessing the probabilities calculated using the formulas mentioned earlier. A high probability suggests a likely event, while a low probability indicates a less likely event.
It's crucial to consider the context of the experiment when interpreting the probabilities. For instance, a probability of 0.8 for a clinical trial’s success suggests a high chance of efficacy, whereas a 0.8 probability of a manufacturing defect could be unacceptable depending on the industry standards.
Limitations of Binomial Experiments
While incredibly useful, binomial experiments have limitations:
-
Independence Assumption: Violations of the independence assumption (e.g., dependent trials) can invalidate the results.
-
Constant Probability Assumption: If the probability of success changes from trial to trial, the binomial model becomes inaccurate.
-
Discrete Data: Binomial experiments only deal with discrete data (whole numbers of successes). They cannot be directly applied to continuous data.
-
Large Sample Sizes: For extremely large sample sizes, the calculations can become computationally intensive. In such cases, approximations like the normal approximation to the binomial can be useful.
Beyond the Basics: Advanced Concepts
This section will touch upon some advanced concepts related to binomial experiments:
1. The Normal Approximation to the Binomial
When 'n' is large and 'p' is not too close to 0 or 1, the binomial distribution can be approximated by a normal distribution. This simplifies calculations and allows for the use of normal distribution properties and tables.
2. Confidence Intervals for Binomial Proportions
Instead of simply estimating the probability of success ('p'), researchers often calculate confidence intervals to provide a range of plausible values for 'p', accounting for sampling variability.
3. Hypothesis Testing with Binomial Data
Hypothesis testing techniques can be used to formally test hypotheses about the probability of success, comparing observed results to expected results under a null hypothesis.
Conclusion
The binomial experiment is a fundamental tool in probability and statistics, with wide-ranging applications across diverse fields. Understanding its underlying principles, formulas, and limitations is crucial for accurately analyzing data and drawing meaningful conclusions. By mastering the concepts outlined in this article, you can effectively apply binomial probability to solve real-world problems and make data-driven decisions. Remember to always carefully consider the assumptions of the model before applying it to your data. Incorrect application can lead to erroneous conclusions. Further exploration of advanced topics like confidence intervals and hypothesis testing will strengthen your understanding and allow for more sophisticated analyses.
Latest Posts
Related Post
Thank you for visiting our website which covers about Consider A Binomial Experiment With And . We hope the information provided has been useful to you. Feel free to contact us if you have any questions or need further assistance. See you next time and don't miss to bookmark.