Compute Diffusion Coefficients For The Interdiffusion Of Carbon
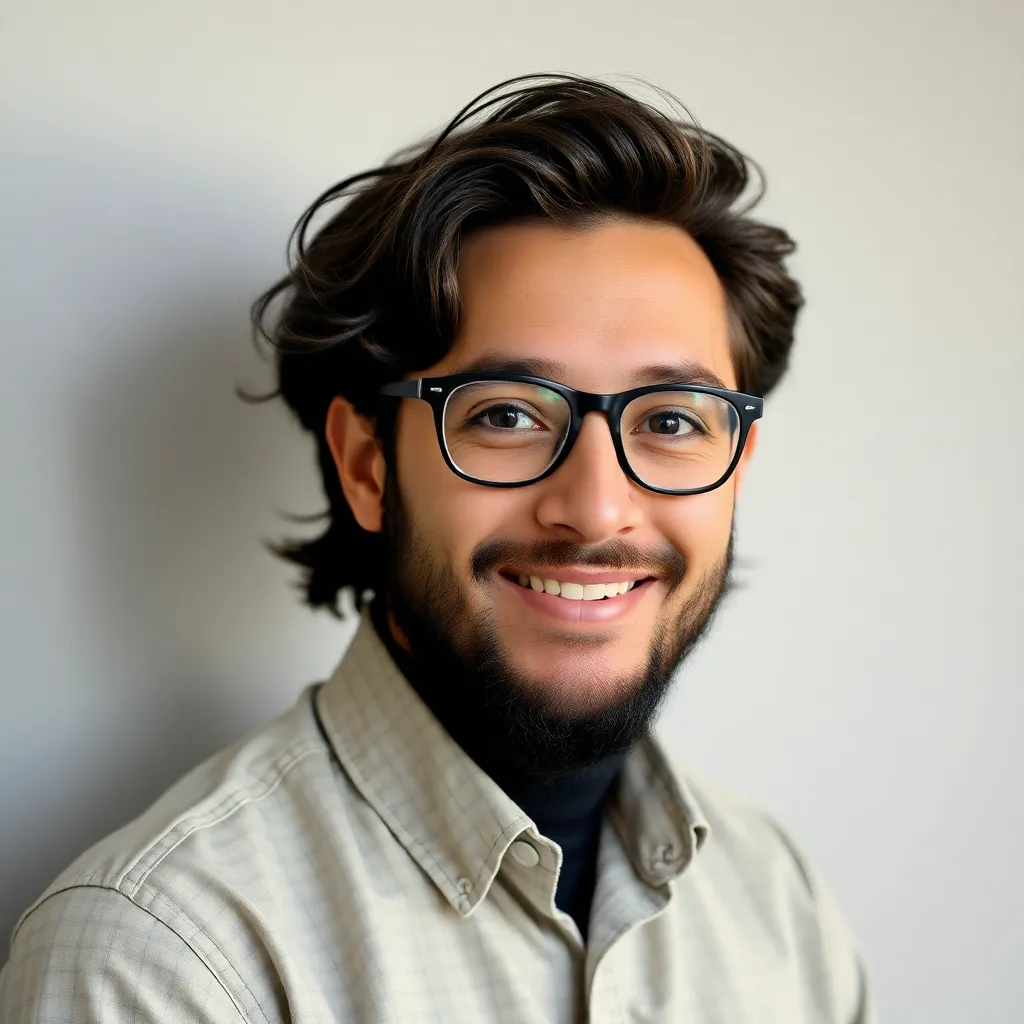
Holbox
May 10, 2025 · 6 min read
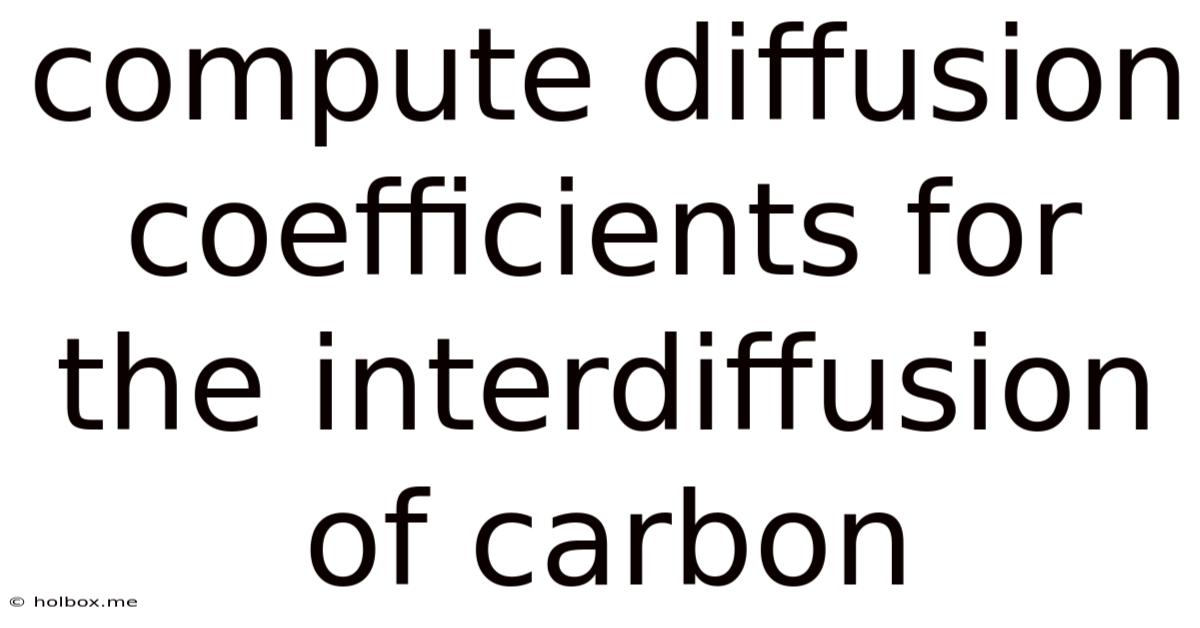
Table of Contents
- Compute Diffusion Coefficients For The Interdiffusion Of Carbon
- Table of Contents
- Computing Diffusion Coefficients for the Interdiffusion of Carbon
- Experimental Determination of Carbon Diffusion Coefficients
- 1. Radiotracer Method
- 2. Gas-Solid Reaction Methods
- 3. Spectroscopic Techniques
- Computational Methods for Determining Carbon Diffusion Coefficients
- 1. Molecular Dynamics (MD) Simulations
- 2. Kinetic Monte Carlo (KMC) Simulations
- 3. Density Functional Theory (DFT) Calculations
- 4. Machine Learning (ML) Techniques
- Factors Affecting Carbon Diffusion Coefficients
- Applications and Significance
- Conclusion
- Latest Posts
- Latest Posts
- Related Post
Computing Diffusion Coefficients for the Interdiffusion of Carbon
The interdiffusion of carbon in various materials is a crucial process in numerous applications, including metallurgy, materials science, and nanotechnology. Understanding and accurately predicting the diffusion coefficient (D) for carbon is essential for optimizing processes like carburization, decarburization, and the synthesis of carbon-based materials. This article provides a comprehensive overview of methods used to compute the diffusion coefficients for the interdiffusion of carbon, covering both experimental and computational techniques.
Experimental Determination of Carbon Diffusion Coefficients
Several experimental techniques are employed to determine the interdiffusion coefficient of carbon in different materials. These methods primarily rely on measuring the concentration profile of carbon as a function of time and position. The accuracy of the obtained diffusion coefficient depends significantly on the precision of the measurement and the choice of the experimental method.
1. Radiotracer Method
This method utilizes radioactive isotopes of carbon, such as <sup>14</sup>C, to track the movement of carbon atoms within the material. By measuring the radioactivity at different positions after a specific diffusion time, the concentration profile can be determined. This technique is highly sensitive and allows for accurate determination of the diffusion coefficient, even at low concentrations. However, it requires specialized equipment to handle radioactive materials and involves safety considerations.
2. Gas-Solid Reaction Methods
These methods involve exposing the material to a controlled atmosphere containing a carbon-bearing gas, such as methane or carbon monoxide. The carbon diffuses into the material, and the resulting concentration profile is analyzed after a specified time. Techniques like carburization and decarburization fall under this category. Precise control over the gas atmosphere and temperature is crucial for accurate results. The concentration profile is often determined using techniques like electron probe microanalysis (EPMA) or secondary ion mass spectrometry (SIMS).
3. Spectroscopic Techniques
Spectroscopic methods, such as Auger electron spectroscopy (AES) and X-ray photoelectron spectroscopy (XPS), can be used to determine the concentration profile of carbon. These techniques offer high spatial resolution, allowing for detailed analysis of the diffusion process. However, the depth profiling capabilities might be limited depending on the instrument and sample preparation.
Computational Methods for Determining Carbon Diffusion Coefficients
Computational methods offer a powerful alternative to experimental techniques, allowing for a detailed understanding of the diffusion mechanisms and prediction of diffusion coefficients under different conditions. These methods typically rely on atomistic simulations, employing various approximations and models.
1. Molecular Dynamics (MD) Simulations
MD simulations model the atomic movements in the material using classical mechanics. The interactions between atoms are described by interatomic potentials, which can be derived from experimental data or ab initio calculations. By tracking the movement of carbon atoms over time, the diffusion coefficient can be calculated using the Einstein relation or similar techniques. MD simulations can provide valuable insights into the diffusion mechanisms and their dependence on factors like temperature, defects, and grain boundaries. However, the accuracy of the results heavily depends on the quality of the interatomic potential used. Furthermore, simulating long diffusion timescales can be computationally expensive.
2. Kinetic Monte Carlo (KMC) Simulations
KMC simulations are stochastic methods that explicitly model the individual jump events of atoms. The jump rates are determined by transition state theory or other methods. KMC is particularly well-suited for simulating diffusion processes over long timescales, as it avoids the computational cost of following the detailed atomic trajectories in MD. KMC simulations can incorporate a wide range of physical phenomena, such as vacancy diffusion, interstitial diffusion, and interactions with defects. However, the accuracy of KMC simulations depends on the accuracy of the input jump rates.
3. Density Functional Theory (DFT) Calculations
DFT calculations provide a first-principles approach for calculating the diffusion coefficient. They rely on solving the Schrödinger equation for the electronic structure of the system, allowing for an accurate description of the atomic interactions. DFT can be used to calculate the energy barriers for atomic jumps and thus obtain accurate jump rates for KMC simulations. However, DFT calculations are computationally expensive, limiting the size of the systems that can be simulated. They are frequently used to obtain high accuracy information for small systems which can then parameterize other techniques like KMC.
4. Machine Learning (ML) Techniques
ML techniques are becoming increasingly popular in materials science for predicting material properties, including diffusion coefficients. ML models can be trained on experimental data or data from ab initio calculations to predict diffusion coefficients for different materials and conditions. This approach can significantly reduce the computational cost associated with traditional computational methods and enable the prediction of diffusion coefficients for a vast range of materials and conditions. However, the accuracy of the prediction depends on the quality and quantity of training data and the choice of ML model.
Factors Affecting Carbon Diffusion Coefficients
Several factors can significantly influence the interdiffusion coefficient of carbon, including:
-
Temperature: The diffusion coefficient generally increases exponentially with temperature, following an Arrhenius-type relationship:
D = D₀ * exp(-Q/RT)
, where D₀ is the pre-exponential factor, Q is the activation energy, R is the gas constant, and T is the absolute temperature. -
Material Composition: The diffusion coefficient varies greatly depending on the host material. Factors such as crystal structure, grain size, and the presence of alloying elements can significantly affect the diffusion behavior.
-
Carbon Concentration: At high carbon concentrations, interactions between carbon atoms can influence the diffusion process, leading to deviations from the simple Arrhenius behavior.
-
Defects: Point defects, such as vacancies and interstitials, can act as diffusion pathways for carbon atoms, leading to enhanced diffusion. Dislocations and grain boundaries also play a significant role in influencing diffusion kinetics.
-
Stress and Strain: Mechanical stresses and strains can alter the diffusion path and potentially accelerate or hinder diffusion.
Applications and Significance
Accurate determination of carbon diffusion coefficients is crucial in various applications, including:
-
Steel Heat Treatments: Controlling the diffusion of carbon during carburization and decarburization is essential for achieving the desired mechanical properties in steel components.
-
Synthesis of Carbon Nanomaterials: Understanding carbon diffusion is critical in the synthesis and processing of carbon nanotubes, graphene, and other carbon-based nanomaterials.
-
Nuclear Materials: Carbon diffusion in nuclear materials affects the integrity and performance of fuel elements.
-
Geological Processes: Carbon diffusion plays a role in the formation and evolution of geological formations.
-
Environmental Science: Carbon diffusion in soils and sediments is essential for understanding the carbon cycle.
Conclusion
Determining the interdiffusion coefficient for carbon requires a multifaceted approach. Experimental techniques offer direct measurements but can be limited by complexity or accessibility. Computational methods provide valuable insights into the mechanisms and allow for exploration under conditions difficult to replicate experimentally. The choice of method depends heavily on the specific material, application, and available resources. Combining experimental and computational techniques often provides the most comprehensive understanding of carbon diffusion and allows for greater confidence in model predictions. Ongoing research in this field continues to refine both experimental and computational approaches, leading to increasingly accurate and efficient techniques for measuring and predicting carbon diffusion coefficients. Future advancements in machine learning and ab initio calculations hold tremendous potential for further improving the accuracy and speed of this critical process.
Latest Posts
Latest Posts
-
What Is 101 Kilos In Stones And Pounds
May 21, 2025
-
What Is 8 5 Stone In Pounds
May 21, 2025
-
How Many Miles Is 6 5 Kilometers
May 21, 2025
-
52 75 Kg In Stones And Pounds
May 21, 2025
-
What Is 40 Percent Of 10
May 21, 2025
Related Post
Thank you for visiting our website which covers about Compute Diffusion Coefficients For The Interdiffusion Of Carbon . We hope the information provided has been useful to you. Feel free to contact us if you have any questions or need further assistance. See you next time and don't miss to bookmark.