Common Data Quality Problems Include All Of The Following Except
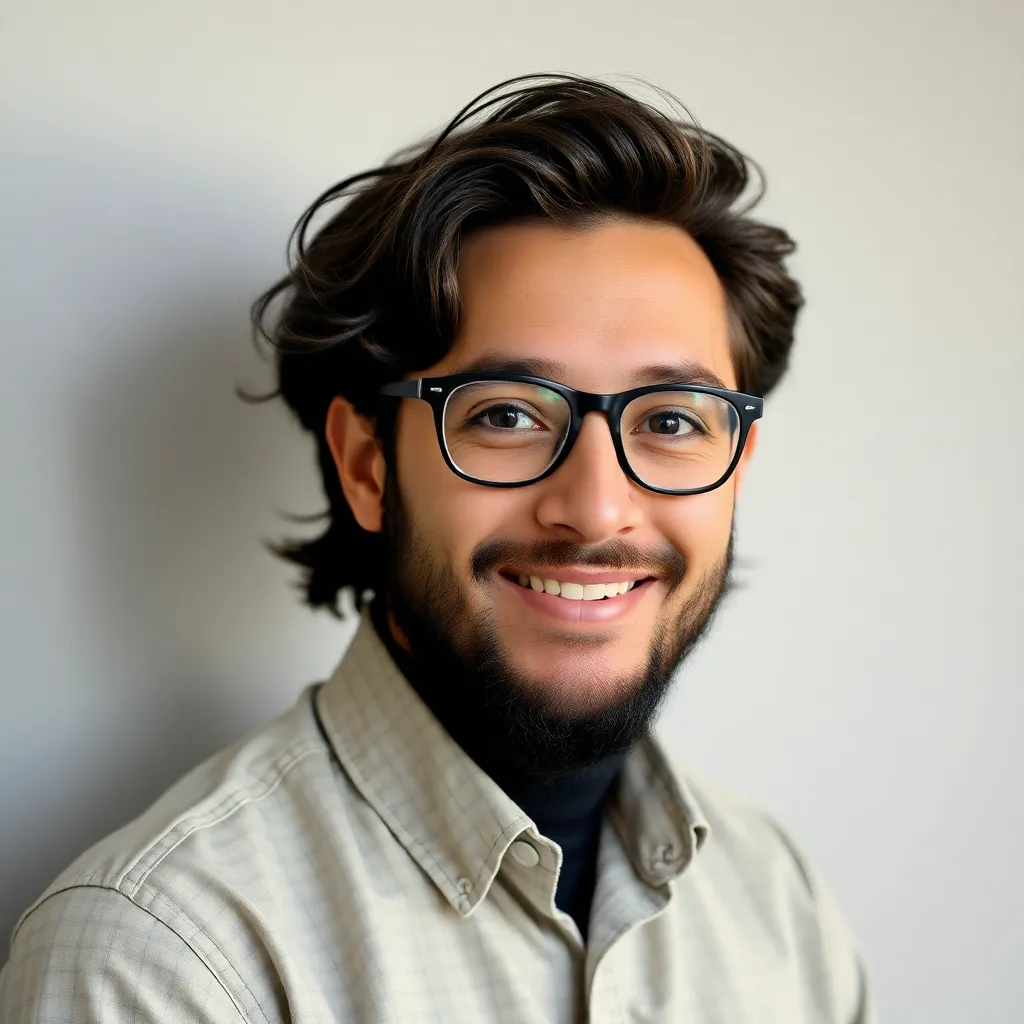
Holbox
May 10, 2025 · 6 min read
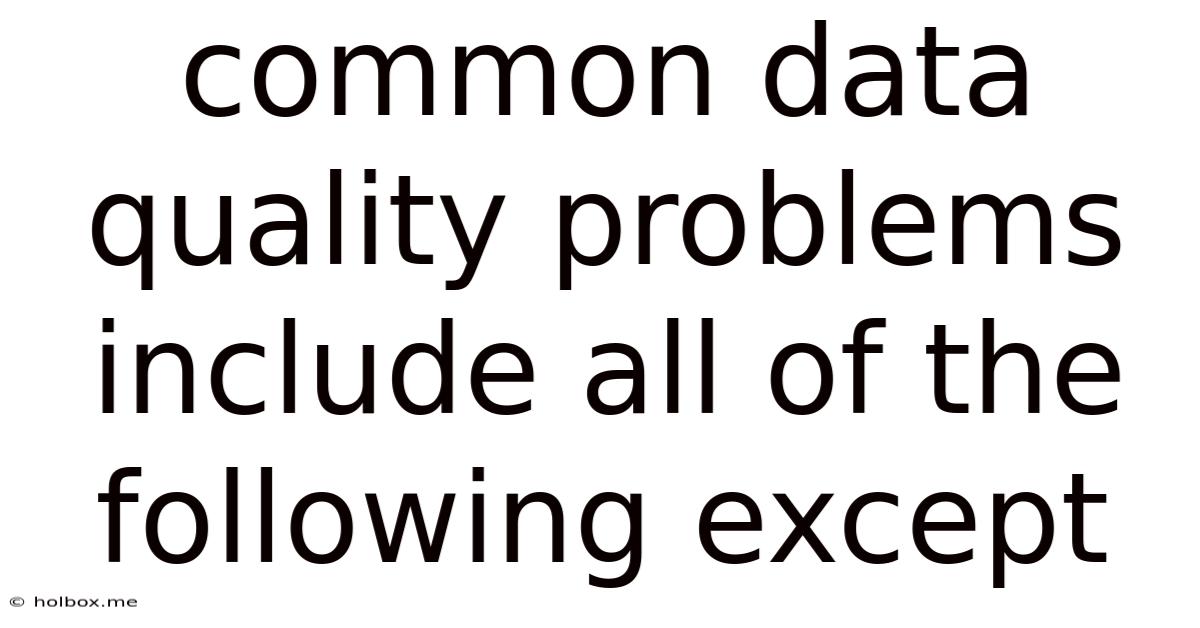
Table of Contents
- Common Data Quality Problems Include All Of The Following Except
- Table of Contents
- Common Data Quality Problems: All Except… Accuracy?
- Common Data Quality Problems: A Deep Dive
- 1. Incompleteness
- 2. Inconsistency
- 3. Inaccuracy
- 4. Invalidity
- 5. Duplication
- The Exception: Accuracy
- Strategies for Improving Data Quality
- Conclusion
- Latest Posts
- Related Post
Common Data Quality Problems: All Except… Accuracy?
Data quality is the cornerstone of any successful business operation. Whether you're using data for marketing campaigns, financial reporting, or product development, the accuracy and reliability of your information directly impact your bottom line. Poor data quality leads to inaccurate insights, flawed decision-making, and ultimately, lost revenue. Understanding common data quality issues is crucial for building robust data management strategies. This article delves into the most prevalent problems, highlighting one notable exception.
Common Data Quality Problems: A Deep Dive
Let's explore the frequently encountered challenges businesses face in maintaining high data quality. These issues often intertwine, creating a complex web of problems that require a multifaceted solution.
1. Incompleteness
Incompleteness refers to missing values within your dataset. This is a pervasive problem, hindering analysis and leading to skewed results. Imagine a customer database with missing phone numbers or email addresses—it significantly limits your ability to reach out to those customers.
Causes of Incompleteness:
- Data entry errors: Human error is a major culprit. Forms might not be filled out completely, or data entry personnel might miss fields.
- Data integration challenges: Combining data from multiple sources often leads to gaps. Different systems might have varying data requirements, resulting in missing information.
- Data migration issues: Moving data from one system to another can introduce incompleteness if the migration process isn't carefully managed.
- Lack of data validation rules: Without clear rules for ensuring data completeness, missing values can easily slip through the cracks.
Consequences of Incompleteness:
- Biased analyses: Incomplete datasets can lead to inaccurate conclusions. Missing data can skew statistical models, making it difficult to draw meaningful insights.
- Limited reporting capabilities: Incomplete data limits your ability to generate comprehensive reports.
- Reduced customer satisfaction: If incomplete customer data hinders effective service delivery, it can lead to dissatisfaction.
2. Inconsistency
Inconsistency refers to conflicting or contradictory data within your dataset. For instance, a customer's name might be spelled differently across various records, or their address might contain conflicting information.
Causes of Inconsistency:
- Multiple data entry points: If data is entered in multiple locations without standardization, inconsistencies are almost guaranteed.
- Lack of data standardization: Without established guidelines for data entry, different people might enter data in different ways.
- Data migration errors: As mentioned earlier, data migration can introduce inconsistencies if data transformation rules are not properly defined.
- Lack of data governance: Without a robust data governance framework, inconsistencies can proliferate unchecked.
Consequences of Inconsistency:
- Difficult data analysis: Inconsistencies make it challenging to aggregate and analyze data accurately.
- Duplicated records: Inconsistent data can lead to the creation of duplicate records, further complicating data management.
- Erroneous reporting: Reports generated from inconsistent data can be unreliable and misleading.
3. Inaccuracy
Inaccuracy refers to data that is simply wrong. This is perhaps the most damaging form of data quality problem. An incorrect customer address, a wrong product price, or a false transaction record can have significant consequences.
Causes of Inaccuracy:
- Human error: This is a primary cause of inaccurate data, ranging from simple typos to more complex mistakes in data entry or calculation.
- Faulty data capture mechanisms: Problems with data collection devices or processes can lead to inaccurate readings or entries.
- Data corruption: Data can become corrupted during storage or transmission, leading to inaccuracies.
- Outdated information: Data that is not regularly updated can become inaccurate over time.
Consequences of Inaccuracy:
- Wrong decisions: Inaccurate data leads to flawed business decisions based on false assumptions.
- Financial losses: Inaccurate data in financial reporting can lead to significant monetary losses.
- Reputational damage: Inaccurate information about customers or products can harm a company's reputation.
4. Invalidity
Invalid data refers to information that doesn't conform to predefined rules or constraints. This might include data that violates data type restrictions, falls outside acceptable ranges, or contains inappropriate characters.
Causes of Invalidity:
- Lack of data validation: Without proper data validation rules, invalid data can easily be entered and stored.
- Poor data entry practices: Failure to adhere to established data entry guidelines can lead to invalid data.
- System limitations: Some systems might not have the capability to enforce all necessary data validation rules.
Consequences of Invalidity:
- System errors: Invalid data can cause errors in applications and systems that process the data.
- Data processing delays: Invalid data often requires manual intervention, causing delays in data processing.
- Data integrity issues: Invalid data can compromise the overall integrity of the dataset.
5. Duplication
Duplicate data refers to redundant records in a dataset. This wastes storage space, complicates analysis, and can lead to inaccurate reporting.
Causes of Duplication:
- Multiple data entry points: Entering data multiple times can easily lead to duplicates.
- Poor data integration practices: Combining data from different sources without proper deduplication can result in duplicates.
- Lack of unique identifiers: Without clear unique identifiers for each record, duplicates are difficult to detect and remove.
Consequences of Duplication:
- Increased storage costs: Storing duplicate data increases storage requirements.
- Skewed analysis: Duplicates can distort analytical results, leading to incorrect conclusions.
- Increased processing time: Processing datasets with duplicates takes longer.
The Exception: Accuracy
While the points above cover many common data quality issues, accuracy isn't an issue separate from the others. In fact, accuracy is the goal in addressing all the other problems. Incompleteness, inconsistency, invalidity, and duplication all contribute to inaccurate data. Addressing these underlying problems directly improves the overall accuracy of your data. Therefore, while we can identify the other four as distinct issues, accuracy is more of a desired outcome rather than a separate category of data quality problems. It’s the ultimate benchmark of successful data management, the fruit of effectively addressing incompleteness, inconsistency, invalidity, and duplication.
Strategies for Improving Data Quality
Improving data quality requires a multifaceted approach. Here are some key strategies:
- Implement data governance: Establish clear policies and procedures for data management.
- Data standardization: Define standard formats and rules for data entry and storage.
- Data validation: Use validation rules to prevent invalid data from entering the system.
- Data cleansing: Regularly clean your data to remove duplicates, inconsistencies, and errors.
- Data integration tools: Employ tools that help integrate data from multiple sources efficiently and accurately.
- Data monitoring: Continuously monitor your data quality to identify and address issues proactively.
- Invest in data quality tools: There are many software tools available to help automate data quality processes.
- Training and education: Educate your staff on best practices for data entry and management.
Conclusion
Maintaining high data quality is paramount for any organization that relies on data-driven decision-making. By understanding and addressing the common data quality problems outlined above – focusing on their contribution to the overarching goal of accuracy – you can ensure the reliability and integrity of your data, enabling you to make better decisions, improve efficiency, and achieve your business objectives. Remember, clean, accurate data is the foundation of a successful data strategy. Don't underestimate its importance.
Latest Posts
Related Post
Thank you for visiting our website which covers about Common Data Quality Problems Include All Of The Following Except . We hope the information provided has been useful to you. Feel free to contact us if you have any questions or need further assistance. See you next time and don't miss to bookmark.