Based On The Values In Cells B77 B81
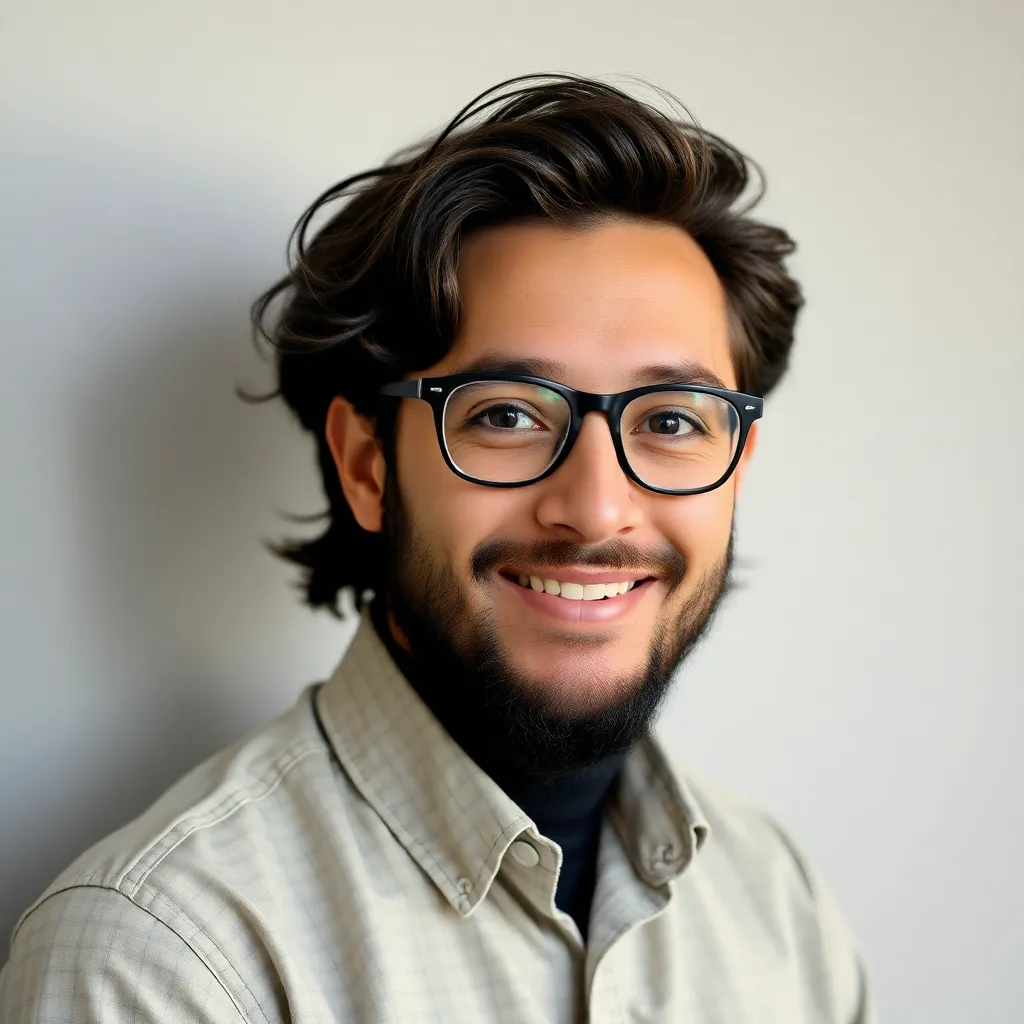
Holbox
Mar 11, 2025 · 5 min read
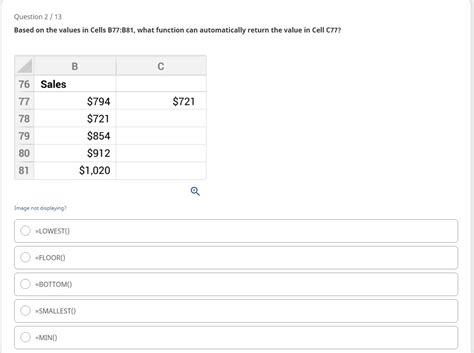
Table of Contents
Data Analysis and Interpretation Based on Values in Cells B77:B81
This article delves into the intricacies of data analysis, focusing specifically on the values found within cells B77 to B81 of a spreadsheet. While the specific content of these cells remains unknown (as it depends on the context of the spreadsheet itself), we will explore various analytical techniques applicable to any numerical or categorical data held within this range. The goal is to provide a comprehensive guide to extracting meaningful insights and actionable conclusions from this data subset.
Understanding the Context: The Importance of Pre-Analysis
Before jumping into the analytical process, it's crucial to understand the context of the data. What do the values in B77:B81 represent? Are they sales figures, test scores, survey responses, or something else entirely? Knowing the nature of the data significantly impacts the choice of analytical methods.
For instance, if the data represents sales figures for five consecutive days, we might focus on calculating averages, identifying trends, and spotting anomalies. If it's survey responses regarding customer satisfaction, we might need to analyze the distribution of responses, calculate percentages, and conduct correlation analyses if other relevant data is available.
Key Questions to Ask Before Analysis:
- What type of data is it? (Numerical, categorical, ordinal, etc.)
- What is the unit of measurement? (Dollars, percentages, units sold, etc.)
- What is the time period covered? (Daily, weekly, monthly, yearly)
- Are there any missing values? How should these be handled?
- Are there any outliers? How should these be handled?
- What are the research questions or objectives? What insights are we trying to gain?
Data Types and Corresponding Analytical Methods
The analytical approach depends heavily on the nature of the data in cells B77:B81. Let's explore various data types and suitable analytical techniques:
1. Numerical Data:
-
Descriptive Statistics: This forms the foundation of any numerical data analysis. Calculating the mean, median, mode, standard deviation, variance, range, and percentiles provides a summary of the central tendency, dispersion, and distribution of the data. These statistics offer valuable insights into the overall characteristics of the data set.
-
Trend Analysis: If the data represents time series data (e.g., daily sales), trend analysis can reveal patterns and predict future values. Techniques like moving averages, exponential smoothing, and linear regression can be employed.
-
Outlier Detection: Identifying and handling outliers is critical. Outliers can significantly skew the results of analyses. Methods include box plots, scatter plots, and Z-score calculations.
-
Correlation Analysis: If there's additional data correlated with the values in B77:B81 (e.g., advertising spend, weather conditions), correlation analysis can help uncover relationships between variables.
2. Categorical Data:
-
Frequency Distribution: Counting the occurrences of each category provides insights into the distribution of the data. This is especially useful for understanding the prevalence of specific responses in surveys or the frequency of different product categories.
-
Contingency Tables: If there's another categorical variable, contingency tables can reveal the relationships between the two variables. Chi-square tests can determine if there's a statistically significant association.
-
Bar Charts and Pie Charts: These visual aids effectively represent categorical data and frequency distributions, making it easier to understand and communicate the results.
Advanced Analytical Techniques
Depending on the data and the research questions, more advanced techniques might be necessary:
-
Regression Analysis: This statistical method explores the relationship between a dependent variable (the values in B77:B81) and one or more independent variables. Linear regression is commonly used for analyzing the relationship between numerical variables. Logistic regression is used when the dependent variable is categorical.
-
Time Series Decomposition: This technique breaks down a time series into its constituent components (trend, seasonality, and residuals) to gain a deeper understanding of the underlying patterns.
-
Clustering Analysis: If the data represents multiple observations, clustering algorithms can group similar data points together, revealing patterns and structures within the data.
-
Machine Learning: For large and complex datasets, machine learning algorithms (such as decision trees, support vector machines, or neural networks) can be used for predictive modeling, classification, and anomaly detection.
Data Visualization: Communicating Insights Effectively
Regardless of the analytical techniques employed, data visualization is crucial for communicating the findings effectively. Appropriate visualizations depend on the data type and the insights to be conveyed.
- Histograms: Display the frequency distribution of numerical data.
- Box plots: Show the distribution, median, quartiles, and outliers of numerical data.
- Scatter plots: Illustrate the relationship between two numerical variables.
- Bar charts: Compare the frequencies of different categories.
- Pie charts: Show the proportion of each category relative to the whole.
- Line charts: Display trends over time.
Handling Missing Values and Outliers
Missing values and outliers are common challenges in data analysis. These need careful consideration:
-
Missing Values: Several strategies can be employed, including deletion (if the proportion of missing values is small), imputation (replacing missing values with estimated values), or using specialized statistical techniques designed to handle missing data.
-
Outliers: Outliers can disproportionately influence the results of statistical analyses. They can be identified using various methods, such as box plots or Z-scores. Depending on the cause and context, outliers can be removed, transformed (e.g., using logarithmic transformations), or included in the analysis with careful consideration of their impact.
Interpreting Results and Drawing Conclusions
Once the data analysis is complete, it's crucial to interpret the results in the context of the initial research questions and objectives. The findings should be presented clearly and concisely, highlighting the key insights and their implications. Any limitations of the analysis should also be acknowledged. Statistical significance should be considered, but equally important is the practical significance and relevance of the findings.
Conclusion: A Holistic Approach to Data Analysis
Analyzing the values in cells B77:B81, or any data subset for that matter, requires a systematic and holistic approach. This involves careful consideration of the data type, context, research questions, choice of analytical methods, handling of missing values and outliers, and effective data visualization. By following these steps, valuable insights can be extracted, leading to informed decision-making and a deeper understanding of the underlying phenomena represented by the data. Remember that the key is to choose the methods most appropriate for your specific data and research objectives. The journey from raw data to meaningful insights is a process of careful planning, meticulous execution, and insightful interpretation. This detailed approach ensures a robust and reliable analysis, ultimately leading to confident and actionable conclusions.
Latest Posts
Latest Posts
-
Please Select The Best Answer From The Choices Provided
Mar 11, 2025
-
How Many Valence Electrons Does Calcium Have
Mar 11, 2025
-
How To Cite A Case Study Apa Format
Mar 11, 2025
-
How Many Valence Electrons Does Na Have
Mar 11, 2025
-
Electric Current Is Measured In Units Of
Mar 11, 2025
Related Post
Thank you for visiting our website which covers about Based On The Values In Cells B77 B81 . We hope the information provided has been useful to you. Feel free to contact us if you have any questions or need further assistance. See you next time and don't miss to bookmark.