Based On The Frequency Distribution Above Is 22.5 A
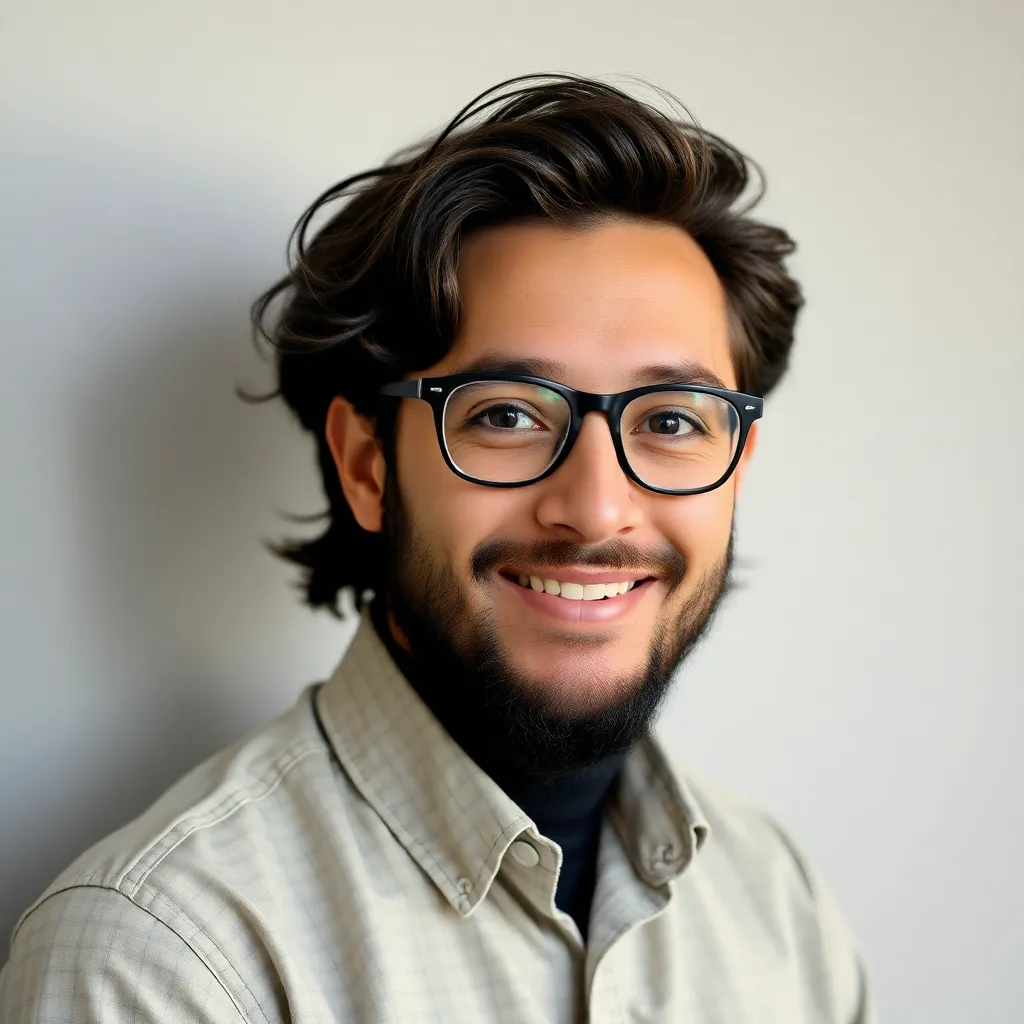
Holbox
Apr 05, 2025 · 7 min read

Table of Contents
- Based On The Frequency Distribution Above Is 22.5 A
- Table of Contents
- Is 22.5 an Outlier? Interpreting Frequency Distributions and Identifying Outliers
- Understanding Frequency Distributions
- Methods for Identifying Outliers
- Choosing the Right Method
- Interpreting the Results
- Case Study: Analyzing 22.5 within a Frequency Distribution
- Conclusion
- Latest Posts
- Latest Posts
- Related Post
Is 22.5 an Outlier? Interpreting Frequency Distributions and Identifying Outliers
Determining whether 22.5 is an outlier requires context. A single number, divorced from its dataset, is meaningless in the outlier assessment. We need the complete frequency distribution to analyze its position relative to the other data points. This article will delve into the methods of identifying outliers within a frequency distribution, using the example of the potential outlier 22.5. We'll explore various statistical techniques and their appropriateness depending on the nature of your data.
Understanding Frequency Distributions
A frequency distribution is a summary of how frequently different values appear in a dataset. It's usually represented in a table or a graph (histogram). The table organizes the data into classes or intervals, showing the number of observations falling within each range. For example:
Data Value Range | Frequency |
---|---|
10-14 | 5 |
15-19 | 12 |
20-24 | 8 |
25-29 | 3 |
30-34 | 2 |
This table shows that 5 observations fall between 10 and 14, 12 between 15 and 19, and so on. Analyzing this distribution helps us understand the central tendency (mean, median, mode), dispersion (variance, standard deviation), and shape of the data. Only with such a distribution can we assess if 22.5 is an outlier.
Methods for Identifying Outliers
Several methods exist to identify outliers, each with its strengths and weaknesses. The choice of method depends on factors such as the size of the dataset, the distribution's shape, and the presence of potential errors in the data.
1. Visual Inspection (Histograms and Box Plots)
The simplest method is a visual inspection of the data using graphical representations.
-
Histograms: These display the frequency distribution as bars, with the height of each bar representing the frequency of observations in that range. Outliers often appear as isolated data points far from the main cluster of data. If 22.5 falls significantly outside the main bars in your histogram, it's a potential outlier.
-
Box Plots (Box-and-Whisker Plots): These provide a visual summary of the data's quartiles, median, and range. Outliers are often depicted as individual points outside the "whiskers" extending from the box. The whiskers typically extend to 1.5 times the interquartile range (IQR) from the first and third quartiles. Any data points beyond these whiskers are considered potential outliers. The IQR is the difference between the third quartile (Q3) and the first quartile (Q1).
Example using a Hypothetical Dataset:
Let's assume your frequency distribution, after creating a histogram, shows a clear clustering of data between 15 and 21, with a few data points around 28-30. If 22.5 falls within the main cluster (15-21), it's unlikely an outlier. However, if it's near 28-30, or even further away, visual inspection suggests it might be an outlier.
2. Z-Score Method
The Z-score measures how many standard deviations a data point is from the mean. A high absolute Z-score (typically above 3 or below -3) indicates a potential outlier.
-
Calculating the Z-score: Z = (X - μ) / σ, where X is the data point (22.5 in our case), μ is the mean of the dataset, and σ is the standard deviation.
-
Interpreting the Z-score: A Z-score of 3 means the data point is 3 standard deviations above the mean. Data points with Z-scores above 3 or below -3 are often flagged as potential outliers.
Limitations: The Z-score method is sensitive to the presence of outliers in the dataset itself. If there are several outliers, the mean and standard deviation will be skewed, affecting the Z-scores of other data points. This method is also best suited for normally distributed data.
3. Modified Z-Score Method
This method addresses the sensitivity of the Z-score to outliers by using the median absolute deviation (MAD) instead of the standard deviation. The MAD is less sensitive to extreme values.
-
Calculating the MAD: The MAD is the median of the absolute deviations from the median.
-
Calculating the Modified Z-score: Modified Z = 0.6745 * (X - Median) / MAD
-
Interpreting the Modified Z-score: Similar to the Z-score, values above 3.5 or below -3.5 are often considered potential outliers.
4. Interquartile Range (IQR) Method
This method uses the IQR to identify outliers. As mentioned earlier, data points falling outside 1.5 times the IQR below Q1 or above Q3 are considered outliers.
-
Calculating the IQR: IQR = Q3 - Q1
-
Identifying Outliers: Lower Bound = Q1 - 1.5 * IQR; Upper Bound = Q3 + 1.5 * IQR
-
Interpretation: Any data points below the lower bound or above the upper bound are considered potential outliers. This method is robust to the presence of outliers because it doesn't depend on the mean.
5. Boxplot Rule
The boxplot rule offers a visual representation of the IQR method, directly showing potential outliers as points outside the whiskers on the boxplot. It provides a clear and easy-to-understand way to identify outliers. This method is best suited for smaller datasets where visual inspection is easier. For larger datasets, consider numerical approaches like Z-scores.
Choosing the Right Method
The best method for identifying outliers depends on the characteristics of your data and your specific goals.
-
Normal Distribution: For data that follows a normal distribution, the Z-score method is a good choice.
-
Skewed Data: For skewed data, the Modified Z-score or IQR method is more robust.
-
Small Datasets: Visual inspection using histograms and box plots is particularly useful for small datasets.
-
Large Datasets: For very large datasets, numerical methods are more efficient.
Interpreting the Results
Once you've identified potential outliers using one or more of these methods, it's crucial to interpret the results carefully.
-
Data Errors: Outliers may represent errors in data collection or recording. Review the original data to identify and correct any errors.
-
True Outliers: Some outliers may represent genuine extreme values within the population you're studying. Consider whether these outliers are valid data points or should be excluded from further analysis.
-
Impact on Analysis: Evaluate the impact of outliers on your statistical analysis. Outliers can significantly influence measures like the mean and standard deviation. Consider using robust statistical methods that are less sensitive to outliers.
Case Study: Analyzing 22.5 within a Frequency Distribution
Let's illustrate the process with a hypothetical example. Suppose we have a frequency distribution of student exam scores:
Score Range | Frequency |
---|---|
0-10 | 2 |
11-20 | 15 |
21-30 | 25 |
31-40 | 12 |
41-50 | 6 |
The score 22.5 falls within the 21-30 range. Visually inspecting a histogram or boxplot made from this data would determine if 22.5 is unusual or sits comfortably within the distribution's main body. If the data appears normally distributed, calculating a Z-score would be appropriate. If there's skewness, the Modified Z-score or IQR method should be used.
Based on this example, 22.5 is unlikely to be an outlier. The score is within the middle range of scores, and the frequencies in surrounding ranges indicate its consistency with the broader pattern. However, without specific data points and the complete frequency distribution, a definitive answer is impossible.
Conclusion
Determining whether 22.5 is an outlier is impossible without the full dataset and its frequency distribution. Multiple methods exist for outlier detection, each suited to different data characteristics. Careful visual inspection, coupled with appropriate statistical techniques like Z-scores, modified Z-scores, or the IQR method, will guide the identification and interpretation of potential outliers. Remember to always consider the context of your data and the potential reasons for outliers before making conclusions. The key is to carefully analyze the entire distribution to understand the context of the value 22.5 within it. By employing a combination of visual and numerical methods, you can confidently assess whether your data point is an outlier and decide how to manage it within your analysis.
Latest Posts
Latest Posts
-
Draw The Neutral Product Of Oxymercuration
Apr 11, 2025
-
The Smallest And Most Significant Taxon Is
Apr 11, 2025
-
The Strategic Appeal Of Related Diversification Is That It
Apr 11, 2025
-
A Patient Has Arrived Late Indeed
Apr 11, 2025
-
Change The Layout Of The Workshop Participants
Apr 11, 2025
Related Post
Thank you for visiting our website which covers about Based On The Frequency Distribution Above Is 22.5 A . We hope the information provided has been useful to you. Feel free to contact us if you have any questions or need further assistance. See you next time and don't miss to bookmark.